基于视频大数据的城市内涝积水深度信息提取方法研究
项目介绍
AI项目解读
基本信息
- 批准号:41601423
- 项目类别:青年科学基金项目
- 资助金额:21.0万
- 负责人:
- 依托单位:
- 学科分类:D0114.地理信息学
- 结题年份:2019
- 批准年份:2016
- 项目状态:已结题
- 起止时间:2017-01-01 至2019-12-31
- 项目参与者:吴辉; 黄杰; 徐启帅; 郑德杰; 熊碧辉; 周里程;
- 关键词:
项目摘要
Spatially detailed depth information of urban waterlogging provides decision-making and scientific basis for urban flood and draining waterlogging. Due to the high costs of existing waterlogging monitoring methods, only limited numbers of monitoring points exist, which makes it difficult to obtain the spatially detailed depth information of urban waterlogging. Urban storm-inundation models can simulate the spatially detailed depth information of urban waterlogging, but they require big amounts of data, and some of these data can be difficult to obtain. So, they cannot be applied easily in urban large areas. The ubiquitous video supervising equipments in cities record the waterlogging process in the visual ways, which have yielded big video data. In this study, we aim to develop a methodology to extract spatially detailed and real-time information of urban waterlogging from big video data. First, the automatic identification method for waterlogging indicators is proposed to identify the objects which are ubiquitous and can be used as water-level scales. Then the waterlogging depth calculation method is used to calculate waterlogging depth based on these indicators. Second, the sampling method, which takes changing of waterlogging area, crowd density, and the spatial-temporal characteristics of waterlogging into account, is used to guarantee the real-time capability of the waterlogging depth calculation method, when dealing with big video data. Finally, the proposed methodology is validated and their adaptabilities are evaluated. This study offers a new angle for urban waterlogging researches. It guarantees to extract the urban waterlogging depth information in the spatially detailed and real-time way from big video data. The achievements of this study can strengthen the capability of monitoring, early warning and forecasting for urban waterlogging.
空间详细的城市内涝积水深度信息是防洪排涝的决策基础和科学依据,现有的积水监测手段成本高昂,监测点数量有限,难以获取空间详细的积水深度信息。雨洪模拟技术虽然可模拟空间上较为详细的积水信息,然而对输入数据要求较高,不易在城市大范围应用。城市中普及的视频监控设备以视觉方式记录着城市的内涝过程,是典型的视频大数据。本项目以城市视频大数据为研究对象,从中挖掘空间详细且实时的城市内涝积水信息。首先,研究积水参照物自动识别方法,从视频图像中识别出泛在的、具有积水指示作用的地物作为积水参照物,进而研究面向泛在积水参照物的积水深度计算方法;然后,研究考虑内涝范围变化和人群密度、积水时空特征的积水点抽样方法,确保积水深度提取的合理性和实时性;最后,对本文方法进行检验与适用性评价。该研究通过对视频大数据进行深入挖掘,提取其中蕴含的内涝积水信息,可进一步提升城市内涝预报预警能力,为城市内涝研究提供一个崭新的视角。
结项摘要
城市大范围且空间精细的内涝积水深度信息可为城市内涝预警和预报提供关键数据,并为应急响应提供决策支持,从而减轻城市内涝造成的生命和财产损失。现有的积水监测手段难以获取城市大范围且空间精细的积水深度信息。遍布城市的摄像头以视觉方式记录城市内涝过程,是获取城市大范围且空间精细的积水深度信息的新型数据源,具有重大的应用价值和潜力。如何从视频大数据中提取积水深度信息是迫切需要解决的问题。为此,本项目提出了基于视频大数据的内涝积水提取方法体系。该方法体系由四部分组成:(1)基于泛在参照物的城市内涝积水深度提取方法。首先,利用基于CNN的目标识别算法从视频图像中检测出非积水状态下的积水参照物(即完整的参照物)和积水状态下的积水参照物(即参照物的水面以上部分);然后,根据完整参照物和参照物水面以上部分的高度差异计算积水深度。(2)基于迁移学习的城市内涝积水深度提取方法。首先,利用迁移学习从城市内涝视频图像中提取特征向量;然后,基于Lasso回归建立特征向量与积水深度的回归模型。(3)基于合成图像集的城市内涝积水深度提取方法。首先,搜集含有参照物的图像和城市内涝场景图像,并从原始图像中裁剪出参照物和水面;然后,分别对参照物和水面图像做数据增强处理以保证图像的数量和多样性;最后,将参照物“粘贴”在水面图像生成带有标注的合成图像集。利用合成图像集训练目标识别算法,继而从视频图像中识别参照物,最后基于参照物计算积水深度。(4)积水点抽样方法。首先,根据暴雨内涝风险确定积水点分布范围和布设密度;然后,根据积水点处积水深度的时空相关性对积水点进行时空抽样。案例应用表明,本项目方法可以有效地从视频图像中提取积水深度,在获取城市大范围且空间精细的积水深度信息方面具有广阔的应用前景。
项目成果
期刊论文数量(3)
专著数量(0)
科研奖励数量(0)
会议论文数量(0)
专利数量(0)
Automatic Estimation of Urban Waterlogging Depths from Video Images Based on Ubiquitous Reference Objects
基于无处不在的参考对象的视频图像自动估计城市内涝深度
- DOI:10.3390/rs11050587
- 发表时间:2019-03-01
- 期刊:REMOTE SENSING
- 影响因子:5
- 作者:Jiang, Jingchao;Liu, Junzhi;Xue, Anke
- 通讯作者:Xue, Anke
A knowledge-based method for the automatic determination of hydrological model structures
基于知识的水文模型结构自动确定方法
- DOI:10.2166/hydro.2019.029
- 发表时间:2019-09
- 期刊:Journal of Hydroinformatics
- 影响因子:2.7
- 作者:Jingchao Jiang;A-Xing Zhu;Cheng-Zhi Qin;Junzhi Liu
- 通讯作者:Junzhi Liu
Extraction of Urban Waterlogging Depth from Video Images Using Transfer Learning
使用迁移学习从视频图像中提取城市内涝深度
- DOI:10.3390/w10101485
- 发表时间:2018-10-01
- 期刊:WATER
- 影响因子:3.4
- 作者:Jiang, Jingchao;Liu, Junzhi;Wang, Dongliang
- 通讯作者:Wang, Dongliang
数据更新时间:{{ journalArticles.updateTime }}
{{
item.title }}
{{ item.translation_title }}
- DOI:{{ item.doi || "--"}}
- 发表时间:{{ item.publish_year || "--" }}
- 期刊:{{ item.journal_name }}
- 影响因子:{{ item.factor || "--"}}
- 作者:{{ item.authors }}
- 通讯作者:{{ item.author }}
数据更新时间:{{ journalArticles.updateTime }}
{{ item.title }}
- 作者:{{ item.authors }}
数据更新时间:{{ monograph.updateTime }}
{{ item.title }}
- 作者:{{ item.authors }}
数据更新时间:{{ sciAawards.updateTime }}
{{ item.title }}
- 作者:{{ item.authors }}
数据更新时间:{{ conferencePapers.updateTime }}
{{ item.title }}
- 作者:{{ item.authors }}
数据更新时间:{{ patent.updateTime }}
其他文献
分布式水文模型的并行计算研究进展
- DOI:--
- 发表时间:2013
- 期刊:地理科学进展
- 影响因子:--
- 作者:刘军志;朱阿兴;秦承志;陈腊娇;吴辉;江净超
- 通讯作者:江净超
道路网的实况连通度指标
- DOI:--
- 发表时间:--
- 期刊:测绘与空间地理信息
- 影响因子:--
- 作者:陈江平;江净超;余洁
- 通讯作者:余洁
一种线状对象空间自相关测度算法
- DOI:--
- 发表时间:--
- 期刊:测绘信息与工程
- 影响因子:--
- 作者:余洁;李岩;陈江平;江净超
- 通讯作者:江净超
其他文献
{{
item.title }}
{{ item.translation_title }}
- DOI:{{ item.doi || "--" }}
- 发表时间:{{ item.publish_year || "--"}}
- 期刊:{{ item.journal_name }}
- 影响因子:{{ item.factor || "--" }}
- 作者:{{ item.authors }}
- 通讯作者:{{ item.author }}
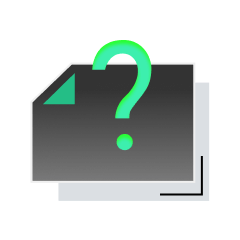
内容获取失败,请点击重试
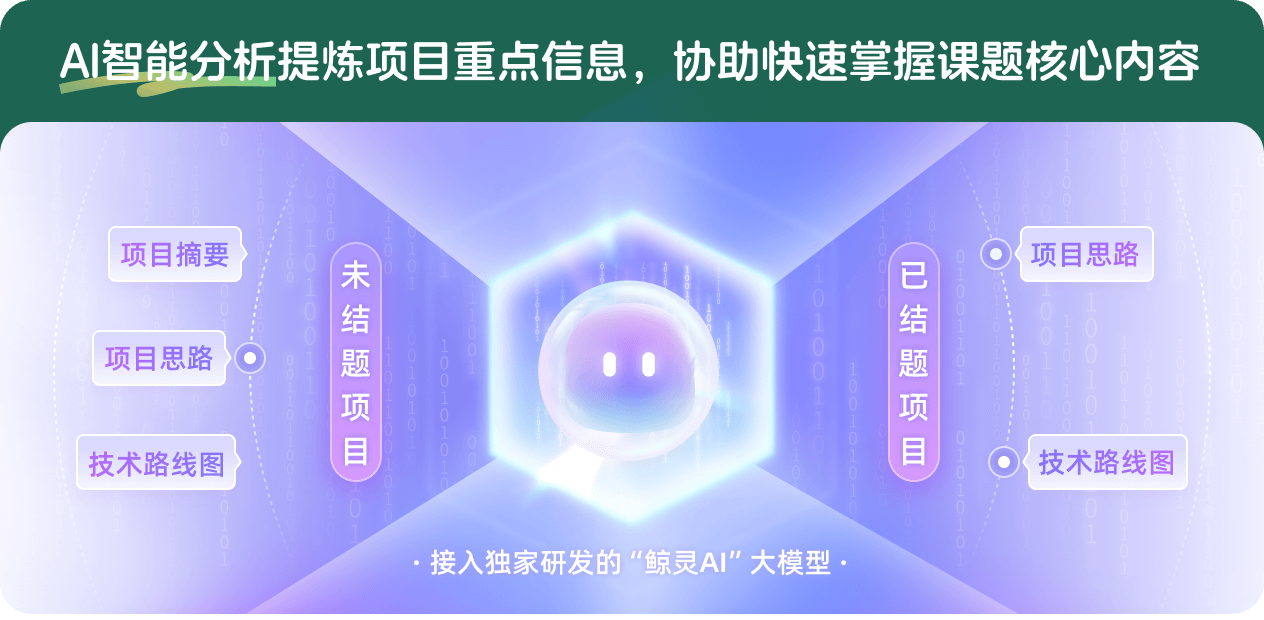
查看分析示例
此项目为已结题,我已根据课题信息分析并撰写以下内容,帮您拓宽课题思路:
AI项目摘要
AI项目思路
AI技术路线图
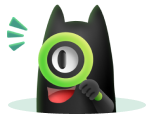
请为本次AI项目解读的内容对您的实用性打分
非常不实用
非常实用
1
2
3
4
5
6
7
8
9
10
您认为此功能如何分析更能满足您的需求,请填写您的反馈:
相似国自然基金
{{ item.name }}
- 批准号:{{ item.ratify_no }}
- 批准年份:{{ item.approval_year }}
- 资助金额:{{ item.support_num }}
- 项目类别:{{ item.project_type }}
相似海外基金
{{
item.name }}
{{ item.translate_name }}
- 批准号:{{ item.ratify_no }}
- 财政年份:{{ item.approval_year }}
- 资助金额:{{ item.support_num }}
- 项目类别:{{ item.project_type }}