多节高铟InGaN基量子阱太阳能电池的研究
项目介绍
AI项目解读
基本信息
- 批准号:61574114
- 项目类别:面上项目
- 资助金额:60.0万
- 负责人:
- 依托单位:
- 学科分类:F0401.半导体材料
- 结题年份:2019
- 批准年份:2015
- 项目状态:已结题
- 起止时间:2016-01-01 至2019-12-31
- 项目参与者:冯仑刚; 王帅; 张敏妍; 赵宇坤; 刘浩; 弓志娜;
- 关键词:
项目摘要
Compared to the traditional solar cell, the InGaN material system, a direct bandgap material, has higher efficiency limit and open circuit voltage. It can be better applied in harsh environment due to its supreme resistance to high temperature and radiation. Therefore, it is very important to area such as national defense, aviation and space exploration. However, the critical problems include the low efficiency of bottom cell with high indium quantum wells; inefficient carrier collection due to large polarization; high resistance of the tunnel junction, a crucial component to connect multiple cells with different indium content. So far the efficiency of the nitride solar cell is rather low, and the growth of tandem cell by MOVPE is far from being mature. In this research, the InGaN/GaN multiple quantum wells is chosen as the basic structures to study the epitaxial growth of tandem solar cell by MOVPE. Based on our experience in optimizing epitaxial defects, modulation of polarization field and micro/nano-machining by electro-chemical etching, we plan to explore the following three major topics: the modification of the structural defects in depletion region with large number of quantum wells; low polarization in high indium quantum wells by selective lateral growth on semi-polar patterned sapphire substrate; highly conductive tunnel junction by activating the buried p-type material using nano-hole structure formed by electro-chemical etching. In the end, it is our goal to triple the efficiency of the current InGaN based solar cell.
与传统太阳能电池相比,直接带隙材料系统InGaN具有更高的转化效率极限,开路电压。其耐高温,抗辐射的特点使其更适合在极端条件下工作,在国防军事,航空航天,空间探测等领域有重要意义。然而目前该方向存在的主要问题包括高铟底层电池量子阱质量差;极化效应导致光生载流子收集效率低;连接不同铟组分单节电池的隧穿结电阻太高。目前氮化物太阳能电池的效率还很低,多节电池的材料生长还远不成熟。本项目采用MOVPE外延材料生长多节InGaN太阳能电池。利用前期在氮化物材料位错优化、高铟材料极化电场控制、电化学刻蚀微纳结构和垂直结构大功率芯片的研究基础,在下面三个大方面进行探索:大数目量子阱耗尽层中结构缺陷的研究和利用;图案化半极性衬底选择性外延生长方式实现弱极化高铟量子阱;电化学刻蚀纳米多孔结构辅助填埋式p-型材料活化实现低阻抗隧穿结。有望将目前InGaN太阳能电池的转换效率提高三倍。
结项摘要
电化学刻蚀形成多孔结构后导致样品内应力有效释放,使得应力诱导的量子限制斯塔克效应(QCSE)减弱,从而有效禁带宽度变大,光谱蓝移。继续增大刻蚀电压,可以观察到更明显的 PL强度提升,一方面同样由于QCSE效应减弱,导致电子-空穴波函数重叠度增加,内量子效率增加;另一方面是由于微孔结构促进了光线多次反射,即提高了器件的光收集效率。纺锤状Ga2O3由于其在深紫外区域的强烈吸收,在日盲solar cell探测中具有巨大的潜力。.NSOM使我们能够测量纳米尺度的EL,它显示出占主导地位的底部QWs发射,并且与附近相比,大V缺陷附近的衰减要小得多。在辐射复合过程中,发现离p-GaN较远的量子阱更多,由此产生的均匀载流子分布增强了总光电转换效率。像质计测量表明,引入大的V型缺陷不会降低晶体质量。改进的V缺陷的实现为提高GaN/InGaN基QWs的大电流效率提供了一条有希望的途径。.我们重点探讨了表面等离子体增强发光二极管发光的机理、结构和关键技术。对具有二维金属光子晶体的氮化镓solar cell光电转换增强机理进行了分析和预测。系统地研究了高In组分solar cell的有源区量子阱(QWs)与表面等离子体(SP, Surface Plasmon)的耦合特性。.综上,成功制备出具有表面等离子体的高In组分solar cell量子阱,并对其光学特性和电学特性进行分析。纳米颗粒的引入,还改善了高In组分solar cell量子阱的热特性。利用这种方法提高solar cell的光电转换效率不仅工艺简单,而且可以大幅度提高工作条件下的光功率。.我们已经成功地获得了基于金字塔阵列的可变形solar cell器件,由于金字塔结构的高机械稳定性,它适合于超柔性器件的制造。.我们系统地研究了半球形空腔的形成机理及其对GaN基500nm高In组分solar cell量子阱的影响。扫描电镜、原子力显微镜和XRD显示,生长在HPSS上的solar cell具有比FSS更好的外延质量。.我们采用金属键合和激光剥离的技术制备垂直结构InGaN/GaN多量子阱太阳能电池。经过金属键合、激光剥离等过程,芯片结构已经被反转,p-GaN被置于底部,n-GaN反转到了上表面。n-GaN表面的粗化和银反射镜的引入,提高了电池对光的有效吸收长度。
项目成果
期刊论文数量(10)
专著数量(0)
科研奖励数量(0)
会议论文数量(0)
专利数量(8)
Metamaterial study of quasi-three-dimensional bowtie nanoantennas at visible wavelengths.
可见波长准三维蝴蝶结纳米天线的超材料研究
- DOI:10.1038/srep41966
- 发表时间:2017-02-08
- 期刊:Scientific reports
- 影响因子:4.6
- 作者:Zhao Y;Yun F;Huang Y;Wang S;Feng L;Li Y;Guo M;Ding W;Zhang Y
- 通讯作者:Zhang Y
Freestanding GaN-based light-emitting diode membranes on Y3Al5O12:Ce3+ crystal phosphor plate for efficient white light emission
Y3Al5O12:Ce3 晶体荧光板上的独立式 GaN 基发光二极管薄膜可实现高效白光发射
- DOI:10.7567/apex.9.081003
- 发表时间:2016
- 期刊:Applied Physics Express
- 影响因子:2.3
- 作者:Feng Lungang;Li Yufeng;Xiong Han;Wang Shuai;Wang Jiangteng;Ding Wen;Zhang Ye;Yun Feng
- 通讯作者:Yun Feng
Super flexible GaN light emitting diodes using microscale pyramid arrays through laser lift-off and dual transfer
通过激光剥离和双转移使用微型金字塔阵列的超柔性 GaN 发光二极管
- DOI:10.1364/oe.26.001817
- 发表时间:2018-01-22
- 期刊:OPTICS EXPRESS
- 影响因子:3.8
- 作者:Tian, Zhenhuan;Li, Yufeng;Lee, S. W. Ricky
- 通讯作者:Lee, S. W. Ricky
InGaN microtube optical resonator with sub-wavelength wall thickness and its application to refractive index sensing
亚波长壁厚InGaN微管光学谐振腔及其在折射率传感中的应用
- DOI:10.1063/1.5098295
- 发表时间:2019-08
- 期刊:Journal of Applied Physics
- 影响因子:3.2
- 作者:Li Yufeng;Hu Peng;Feng Lungang;Du Mengqi;Su Xilin;Li Qiang;Yun Feng
- 通讯作者:Yun Feng
Whispering gallery mode lasing from InGaN/GaN quantum well microtube
InGaN/GaN 量子阱微管发出回音壁模式激光
- DOI:10.1364/oe.25.018072
- 发表时间:2017
- 期刊:Optics Express
- 影响因子:3.8
- 作者:Li Yufeng;Feng Lungang;Su Xilin;Li Qiang;Yun Feng;Yuan Ge;Han Jung
- 通讯作者:Han Jung
数据更新时间:{{ journalArticles.updateTime }}
{{
item.title }}
{{ item.translation_title }}
- DOI:{{ item.doi || "--"}}
- 发表时间:{{ item.publish_year || "--" }}
- 期刊:{{ item.journal_name }}
- 影响因子:{{ item.factor || "--"}}
- 作者:{{ item.authors }}
- 通讯作者:{{ item.author }}
数据更新时间:{{ journalArticles.updateTime }}
{{ item.title }}
- 作者:{{ item.authors }}
数据更新时间:{{ monograph.updateTime }}
{{ item.title }}
- 作者:{{ item.authors }}
数据更新时间:{{ sciAawards.updateTime }}
{{ item.title }}
- 作者:{{ item.authors }}
数据更新时间:{{ conferencePapers.updateTime }}
{{ item.title }}
- 作者:{{ item.authors }}
数据更新时间:{{ patent.updateTime }}
其他文献
其他文献
{{
item.title }}
{{ item.translation_title }}
- DOI:{{ item.doi || "--" }}
- 发表时间:{{ item.publish_year || "--"}}
- 期刊:{{ item.journal_name }}
- 影响因子:{{ item.factor || "--" }}
- 作者:{{ item.authors }}
- 通讯作者:{{ item.author }}
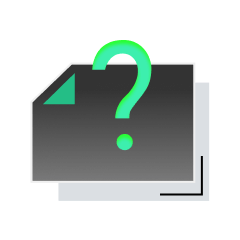
内容获取失败,请点击重试
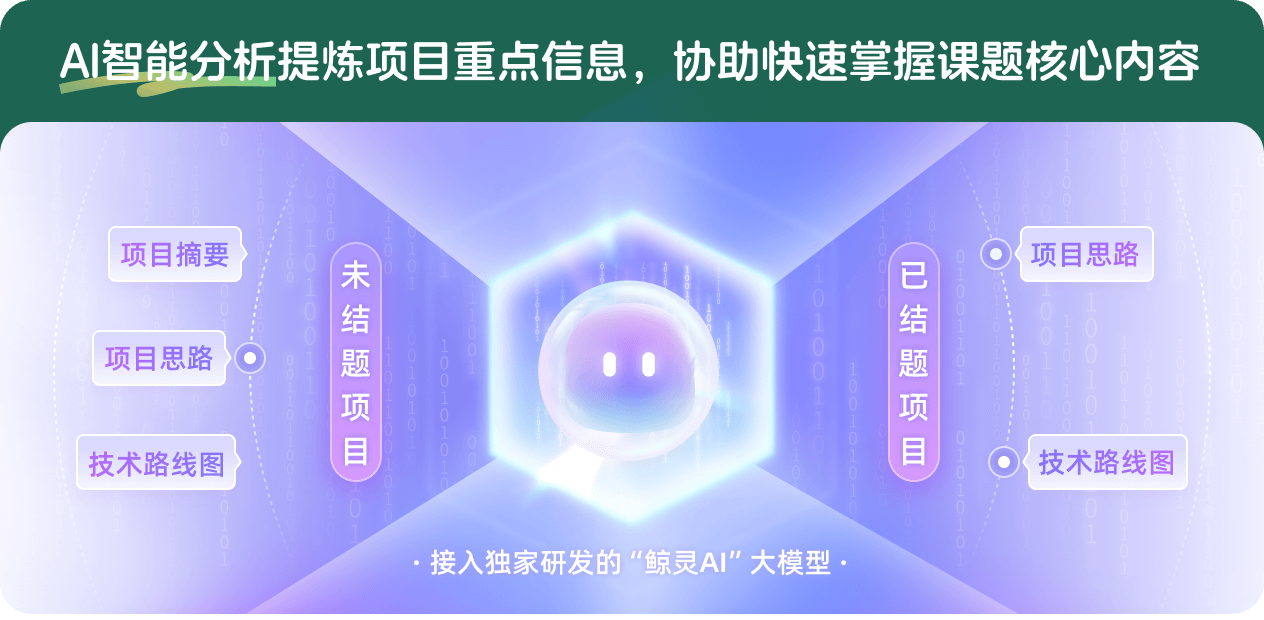
查看分析示例
此项目为已结题,我已根据课题信息分析并撰写以下内容,帮您拓宽课题思路:
AI项目摘要
AI项目思路
AI技术路线图
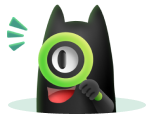
请为本次AI项目解读的内容对您的实用性打分
非常不实用
非常实用
1
2
3
4
5
6
7
8
9
10
您认为此功能如何分析更能满足您的需求,请填写您的反馈:
李虞锋的其他基金
大电流密度下绿光LED内量子效率的研究
- 批准号:61404101
- 批准年份:2014
- 资助金额:26.0 万元
- 项目类别:青年科学基金项目
相似国自然基金
{{ item.name }}
- 批准号:{{ item.ratify_no }}
- 批准年份:{{ item.approval_year }}
- 资助金额:{{ item.support_num }}
- 项目类别:{{ item.project_type }}
相似海外基金
{{
item.name }}
{{ item.translate_name }}
- 批准号:{{ item.ratify_no }}
- 财政年份:{{ item.approval_year }}
- 资助金额:{{ item.support_num }}
- 项目类别:{{ item.project_type }}