畜禽粪便堆肥中CH4和N2O排放及其微生物学机制研究
项目介绍
AI项目解读
基本信息
- 批准号:41401321
- 项目类别:青年科学基金项目
- 资助金额:26.0万
- 负责人:
- 依托单位:
- 学科分类:D0710.土壤侵蚀与土壤肥力
- 结题年份:2017
- 批准年份:2014
- 项目状态:已结题
- 起止时间:2015-01-01 至2017-12-31
- 项目参与者:刘树伟; 赵军; 靳亚果; 宋黎娜; 高翔;
- 关键词:
项目摘要
Agricultural wastes, such as manure, is the considerable emission source of CH4 and N2O, which are important atmospheric greenhouse gases (GHGs). Manure composting reveals significant mitigation potential of CH4 and N2O emission in agriculture. This study will investigate the emission patterns of CH4 and N2O and the related microbial mechanisms during composting of different organic substrates by closed-chamber method and molecular techniques. This study will address the following items as listed: (i) The emission patterns and intensity of GHGs (CH4 and N2O) during the composting of different organic substrates (chicken manure, pig manure, and dairy manure). (ii) The effects of different mitigation measures (application of biochar and azotobacterin) on the emission of GHGs during manure composting and the mitigation potential. (iii) The microbial community structure and gene functions associated with the effects of substrates type and mitigation measures on GHGs emission during manure composting. The results of this study will provide data support for assessment of the emission intensity of CH4 and N2O and the mitigation potential during manure composting, and offer scientific evidence for revealing the microbial-driven mechanisms associated to GHGs emission during composting.
畜禽粪便等农业废弃物是大气温室气体甲烷(CH4)和氧化亚氮(N2O)的重要排放源,畜禽粪便等农业废弃物综合利用对减缓CH4和N2O农业源排放具有较大的潜力。本项目拟以不同有机物料的堆肥为对象,采用静态箱法与分子手段相结合,研究堆肥过程中温室气体CH4和N2O排放及其微生物学机制。项目将完成:(1)不同有机物料(鸡粪、猪粪及牛粪)堆肥过程中的温室气体(CH4、N2O)排放特征及其排放强度;(2)碳氮调控(生物质炭及固氮菌剂施用)对畜禽粪便堆肥中温室气体排放通量的影响规律及其减排潜力;(3)不同有机物料及碳氮调控措施对堆肥过程中温室气体排放影响的微生物群落结构及其基因功能。研究结果将为合理评估畜禽粪便堆肥中CH4和N2O排放量及其减排潜力提供数据支撑,为揭示堆肥过程中温室气体排放的微生物学驱动机制提供科学依据。
结项摘要
畜禽粪便好氧堆肥是实现废弃物稳定化、无害化和资源化的重要途径,然而在堆肥过程中会产生大量温室气体,造成环境污染。目前有关堆肥过程中温室气体排放特征的观测、减排潜力评估和具体微生物学驱动机制的报道还很少。本项目以工厂化条垛式畜禽粪便堆肥为研究对象,围绕其温室气体排放强度及驱动机制开展研究,获得了以下主要结果:(1)通过原位工厂化堆肥实验,利用静态暗箱-气相色谱法测定了堆肥全过程的CH4与N2O排放速率,堆肥过程中的CH4与N2O排放趋势与稻田中类似,呈现出此消彼长(trade-off)的关系。CH4的排放主要集中在堆肥过程的前期,而N2O的排放主要在堆肥成熟期,即堆肥温度与氧气含量均出现下降的后熟阶段。通过定量PCR微生物功能基因丰度同步检测技术,揭示了堆体理化特性与微生物功能基因丰度变化相互作用共同调控有机肥堆制过程中CH4与N2O排放的动态变化的微生物学机制。微生物功能基因丰度与CH4和N2O排放通量呈显著相关关系。逐步回归拟合结果表明,CH4排放通量动态主要由堆体温度、mcrA基因丰度以及NH4+变化得以解释;而N2O排放通量动态主要取决于nirk、nosZ和pmoA基因丰度变化。(2)研究了生物质炭的使用对堆肥过程中N2O的排放趋势的影响及相关的微生物学机制。实验结果表明,生物质炭的添加能显著降低N2O的排放通量主要原因是因为生物质炭对nirK基因(亚硝酸还原酶)丰度的抑制。相关性分析表明,N2O的排放通量与堆肥过程中nirK基因的丰度呈显著正相关关系,而与nosZ基因呈显著负相关关系。本实验结果说明生物质炭的添加可以作为减少温室气体N2O排放量的途径之一。(3)比较了农业废弃物常规堆置与条垛式堆肥温室气体排放差异。与常规堆置相比,农业废弃物条垛式分别增加了226.2%和374.6%的CO2和CH4排放,但能够减少72.5%的N2O排放。本项目明确了农业废弃物条垛式堆肥CH4及N2O的排放系数,并揭示了其排放机制,该研究结果为合理评估畜禽粪便堆肥中CH4和N2O排放量及其减排潜力提供数据支撑,为揭示堆肥过程中温室气体排放的微生物学驱动机制提供科学依据。
项目成果
期刊论文数量(3)
专著数量(0)
科研奖励数量(0)
会议论文数量(0)
专利数量(0)
接种复合菌剂对牛粪好氧堆肥进程及温室气体(CH4和N2O)排放的影响
- DOI:--
- 发表时间:2017
- 期刊:南京农业大学学报
- 影响因子:--
- 作者:李舒清;张镜丹;纪程;顾雯雯;李荣;邹建文
- 通讯作者:邹建文
Microbial Abundances Predict Methane and Nitrous Oxide Fluxes from a Windrow Composting System.
微生物丰度可预测料堆堆肥系统中的甲烷和一氧化二氮通量
- DOI:10.3389/fmicb.2017.00409
- 发表时间:2017
- 期刊:Frontiers in microbiology
- 影响因子:5.2
- 作者:Li S;Song L;Gao X;Jin Y;Liu S;Shen Q;Zou J
- 通讯作者:Zou J
生物质炭对堆肥N2O排放的影响及其微生物学机制研究
- DOI:--
- 发表时间:2016
- 期刊:AMB express
- 影响因子:3.7
- 作者:李舒清;刘树伟;邹建文
- 通讯作者:邹建文
数据更新时间:{{ journalArticles.updateTime }}
{{
item.title }}
{{ item.translation_title }}
- DOI:{{ item.doi || "--"}}
- 发表时间:{{ item.publish_year || "--" }}
- 期刊:{{ item.journal_name }}
- 影响因子:{{ item.factor || "--"}}
- 作者:{{ item.authors }}
- 通讯作者:{{ item.author }}
数据更新时间:{{ journalArticles.updateTime }}
{{ item.title }}
- 作者:{{ item.authors }}
数据更新时间:{{ monograph.updateTime }}
{{ item.title }}
- 作者:{{ item.authors }}
数据更新时间:{{ sciAawards.updateTime }}
{{ item.title }}
- 作者:{{ item.authors }}
数据更新时间:{{ conferencePapers.updateTime }}
{{ item.title }}
- 作者:{{ item.authors }}
数据更新时间:{{ patent.updateTime }}
其他文献
土壤一氧化氮耦合氧化亚氮排放的整合分析研究
- DOI:--
- 发表时间:2016
- 期刊:Global Change Biology
- 影响因子:11.6
- 作者:靳亚果;李舒清;李兆富;邹建文
- 通讯作者:邹建文
稻田和鱼/蟹养殖湿地甲烷和氧化亚氮排放的观测比较研究
- DOI:--
- 发表时间:2015
- 期刊:Environmental Science & Technology
- 影响因子:11.4
- 作者:吴双;李舒清;李兆富;邹建文
- 通讯作者:邹建文
其他文献
{{
item.title }}
{{ item.translation_title }}
- DOI:{{ item.doi || "--" }}
- 发表时间:{{ item.publish_year || "--"}}
- 期刊:{{ item.journal_name }}
- 影响因子:{{ item.factor || "--" }}
- 作者:{{ item.authors }}
- 通讯作者:{{ item.author }}
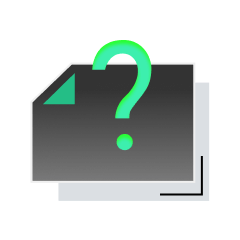
内容获取失败,请点击重试
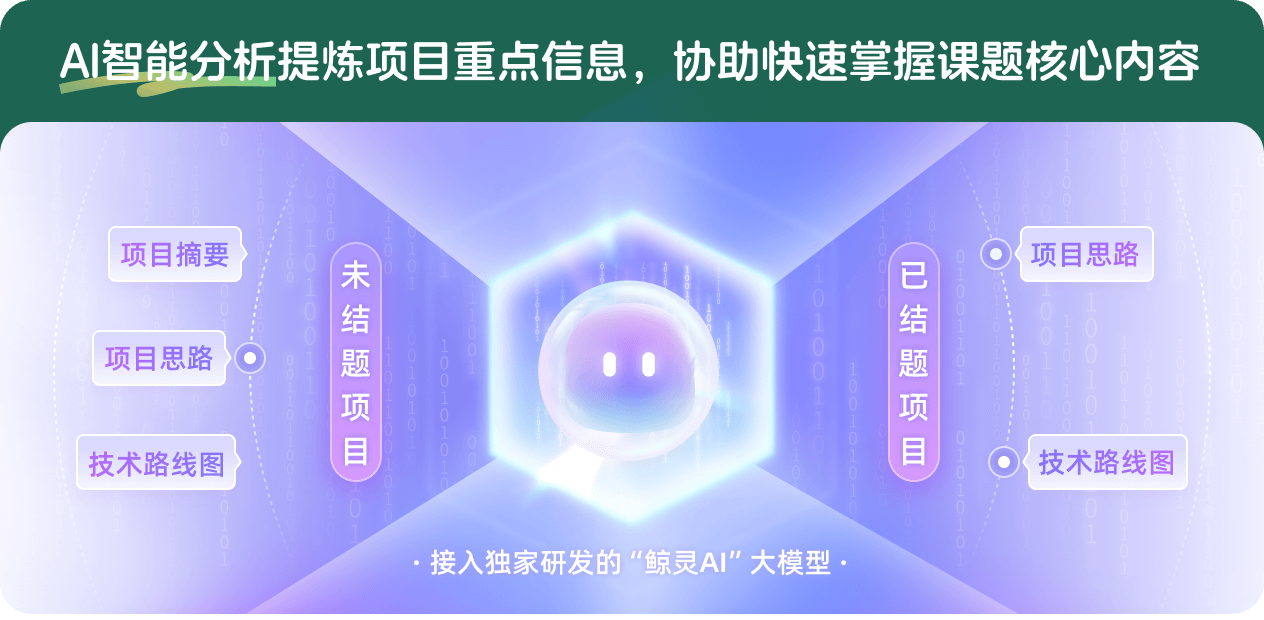
查看分析示例
此项目为已结题,我已根据课题信息分析并撰写以下内容,帮您拓宽课题思路:
AI项目摘要
AI项目思路
AI技术路线图
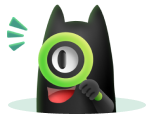
请为本次AI项目解读的内容对您的实用性打分
非常不实用
非常实用
1
2
3
4
5
6
7
8
9
10
您认为此功能如何分析更能满足您的需求,请填写您的反馈:
李舒清的其他基金
有机肥施用对设施菜地土壤氮氧化物排放影响的底物效应与微生物学机制
- 批准号:41877093
- 批准年份:2018
- 资助金额:61.0 万元
- 项目类别:面上项目
相似国自然基金
{{ item.name }}
- 批准号:{{ item.ratify_no }}
- 批准年份:{{ item.approval_year }}
- 资助金额:{{ item.support_num }}
- 项目类别:{{ item.project_type }}
相似海外基金
{{
item.name }}
{{ item.translate_name }}
- 批准号:{{ item.ratify_no }}
- 财政年份:{{ item.approval_year }}
- 资助金额:{{ item.support_num }}
- 项目类别:{{ item.project_type }}