混合模型下贝叶斯信息标准的拓展研究
项目介绍
AI项目解读
基本信息
- 批准号:61403337
- 项目类别:青年科学基金项目
- 资助金额:25.0万
- 负责人:
- 依托单位:
- 学科分类:F0603.机器学习
- 结题年份:2017
- 批准年份:2014
- 项目状态:已结题
- 起止时间:2015-01-01 至2017-12-31
- 项目参与者:李淑兰; 李鑫; 刘超; 曹阳; 卢金长; 刘梦琦;
- 关键词:
项目摘要
This project concerns model selection in mixtures models. The well-known Bayesian information criterion (BIC) is frequently used for this purpose. However, it is found that BIC penalizes the sub-model in each cluster implausibly using the whole sample size, leading to over-penalization. To overcome this problem, based on the available research works of PI, this project will originally propose a new criterion called hierarchical BIC for Gaussian mixture models. The novelty is that each sub-model is penalized using its own effective sample size only. Theoretically, it will be shown that hierarchical BIC is a large sample approximation of variational Bayesian (VB) lower bound and BIC is a further approximation. Empirically, hierarchical BIC and BIC will be compared comprehensively on choosing the number of clusters and the type of covariance structures simultaneously. For mixtures of factor analyzers where the covariance has a factor structure, an efficient learning algorithm which can perform parameter estimation and model selection simultaneously will be developed, alleviating greatly the heavy computational load suffered by the existing two-stage learning algorithm. Finally, the above results such as hierarchical BIC and the learning algorithm under the Gaussian cases will be extended to the counterparts modelling by multivariate t distributions. Applications such as unsupervised clustering and statistical classification will be investigated.
本项目关心的是有限混合模型的模型选择问题,著名的贝叶斯信息标准(BIC)频繁地用于此目的。然而,BIC不合理地用整个样本量惩罚每个聚类子模型的模型复杂性,造成过惩罚。为克服这一问题,在课题申请人现有工作基础上,本项目针对高斯混合模型原创性提出一种新的模型选择标准称为分级BIC,新颖性在于仅用各个子模型自身的有效子样本量来惩罚模型复杂性。理论上,将证明提出的分级BIC是变分贝叶斯置信下界的大样本极限且BIC是其进一步近似。实验上将全面比较分级BIC和BIC同时选择类别个数和子模型协方差结构的表现。对于协方差结构为因子结构的高斯混合因子分析模型,将开发计算上更为有效的学习算法,能够同时进行参数估计和模型选择,克服存在两阶段法计算负荷较严重的问题。拓展方面将上述高斯情形的结果如分级BIC以及有效学习算法推广到多元t分布相应的混合模型。应用方面将考查非监督聚类、统计分类等。
结项摘要
本项目对有限混合模型的模型选择问题开展研究,完成了原定研究计划,主要取得了如下多项研究成果和阶段性成果。(1)提出了一种新的混合模型选择准则,适用于不同成分模型具有共同参数约束,理论上分析了提出的新准则与变分贝叶斯置信下界以及BIC的关系, 并应用于高斯混合模型族;(2)提出了一种新的混合模型选择准则,适用于不同成分模型没有共同参数约束,新颖性在于仅用各个成分模型自身的有效子样本量来惩罚模型复杂性。提出的准则应用于混合因子分析模型,开发了一个新的有效学习算法,将参数估计和模型选择同时进行;(3)在缺失数据情形下,提出了一个新型的模型选择标准,其特点在于仅利用实际观测数据信息来惩罚模型。(4)在判别分析拓展研究方面,提出一种新的针对二维数据的两阶段正则化判别分析方法,实证研究结果表明,提出的方法比传统的正则化线性判别分析以及一些相关方法具有更为优异的分类表现。..项目组在国内外机器学习领域、统计学领域著名期刊发表论文3篇,均为SCI收录,包括1篇《IEEE Transactions on Neural Networks and Learning Systems》,2篇统计学领域期刊《Computational Statistics & Data Analysis》,另有2篇论文处于撰写、修改过程中;获得云南省哲学社科优秀成果奖三等奖1项;培养研究生共计9名。按预期目标完成了研究任务。
项目成果
期刊论文数量(3)
专著数量(0)
科研奖励数量(1)
会议论文数量(0)
专利数量(0)
Mixture model selection via hierarchical BIC
通过分层 BIC 进行混合模型选择
- DOI:10.1016/j.csda.2015.01.019
- 发表时间:2015
- 期刊:Computational Statistics & Data Analysis
- 影响因子:1.8
- 作者:Jianhua Zhao;Libin Jin;Lei Shi
- 通讯作者:Lei Shi
Case deletion diagnostics for GMM estimation
GMM 估计的案例删除诊断
- DOI:10.1016/j.csda.2015.10.003
- 发表时间:2016-03
- 期刊:Computational Statistics & Data Analysis
- 影响因子:1.8
- 作者:Lei Shi;Jun Lu;Jianhua Zhao;Gemai Chen
- 通讯作者:Gemai Chen
数据更新时间:{{ journalArticles.updateTime }}
{{
item.title }}
{{ item.translation_title }}
- DOI:{{ item.doi || "--"}}
- 发表时间:{{ item.publish_year || "--" }}
- 期刊:{{ item.journal_name }}
- 影响因子:{{ item.factor || "--"}}
- 作者:{{ item.authors }}
- 通讯作者:{{ item.author }}
数据更新时间:{{ journalArticles.updateTime }}
{{ item.title }}
- 作者:{{ item.authors }}
数据更新时间:{{ monograph.updateTime }}
{{ item.title }}
- 作者:{{ item.authors }}
数据更新时间:{{ sciAawards.updateTime }}
{{ item.title }}
- 作者:{{ item.authors }}
数据更新时间:{{ conferencePapers.updateTime }}
{{ item.title }}
- 作者:{{ item.authors }}
数据更新时间:{{ patent.updateTime }}
其他文献
Growth parameter dependence of magnetic property of CrAs thin film
CrAs薄膜磁性能的生长参数依赖性
- DOI:10.1088/1009-1963/16/12/053
- 发表时间:2007-12
- 期刊:Chin. Phys.
- 影响因子:--
- 作者:赵建华
- 通讯作者:赵建华
黄土高原冬季湍流相干结构在地表能量平衡中的作用
- DOI:10.6038/cjg2022q0184
- 发表时间:--
- 期刊:地球物理学报
- 影响因子:--
- 作者:赵建华;张良;王胜;梁芸;隆霄;王涓力
- 通讯作者:王涓力
塔北隆起哈拉哈塘凹陷东河砂岩成岩作用及其控制因素
- DOI:--
- 发表时间:2014
- 期刊:现代地质
- 影响因子:--
- 作者:刘永福;赵建华;范秋海等
- 通讯作者:范秋海等
枸杞木糖异构酶基因LbxylA的克隆_原核表达及多克隆抗体的制备
- DOI:--
- 发表时间:2018
- 期刊:食品科学
- 影响因子:--
- 作者:赵建华;李浩霞;尹跃;王亚军;李彦龙;樊云芳;安巍;曹有龙
- 通讯作者:曹有龙
后方韧带复合体回植重建椎管内肿瘤切除术后脊柱稳定性
- DOI:--
- 发表时间:2015
- 期刊:局解手术学杂志
- 影响因子:--
- 作者:殷翔;朱军;王颖博;刘佰易;靳羽飞;刘鹏;赵建华
- 通讯作者:赵建华
其他文献
{{
item.title }}
{{ item.translation_title }}
- DOI:{{ item.doi || "--" }}
- 发表时间:{{ item.publish_year || "--"}}
- 期刊:{{ item.journal_name }}
- 影响因子:{{ item.factor || "--" }}
- 作者:{{ item.authors }}
- 通讯作者:{{ item.author }}
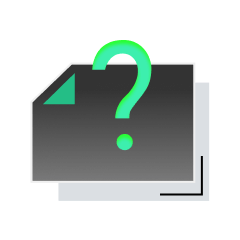
内容获取失败,请点击重试
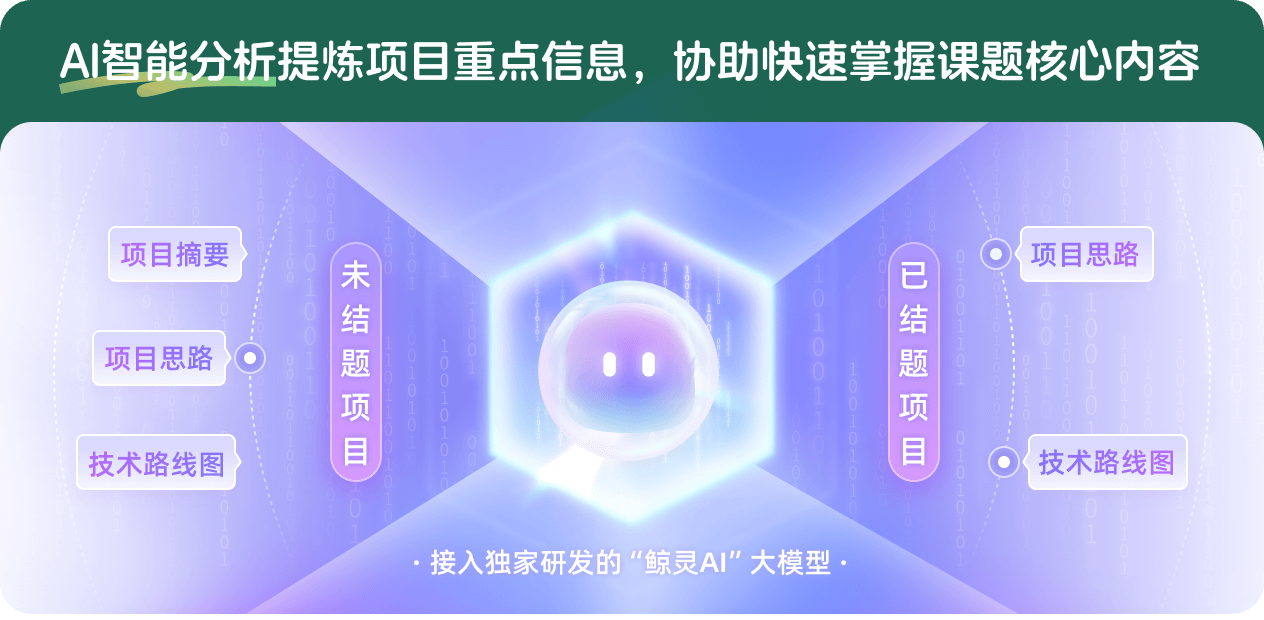
查看分析示例
此项目为已结题,我已根据课题信息分析并撰写以下内容,帮您拓宽课题思路:
AI项目摘要
AI项目思路
AI技术路线图
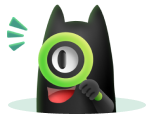
请为本次AI项目解读的内容对您的实用性打分
非常不实用
非常实用
1
2
3
4
5
6
7
8
9
10
您认为此功能如何分析更能满足您的需求,请填写您的反馈:
赵建华的其他基金
稳健双线性概率主成分分析及拓展研究
- 批准号:12161089
- 批准年份:2021
- 资助金额:33 万元
- 项目类别:地区科学基金项目
简约混合矩阵正态模型及其有效模型选择
- 批准号:11761076
- 批准年份:2017
- 资助金额:36.5 万元
- 项目类别:地区科学基金项目
双线性因子分析及其拓展研究
- 批准号:11361071
- 批准年份:2013
- 资助金额:40.0 万元
- 项目类别:地区科学基金项目
相似国自然基金
{{ item.name }}
- 批准号:{{ item.ratify_no }}
- 批准年份:{{ item.approval_year }}
- 资助金额:{{ item.support_num }}
- 项目类别:{{ item.project_type }}
相似海外基金
{{
item.name }}
{{ item.translate_name }}
- 批准号:{{ item.ratify_no }}
- 财政年份:{{ item.approval_year }}
- 资助金额:{{ item.support_num }}
- 项目类别:{{ item.project_type }}