野生大豆优异基因型的发掘搜集及大豆起源进化研究
项目介绍
AI项目解读
基本信息
- 批准号:39670448
- 项目类别:面上项目
- 资助金额:8.0万
- 负责人:
- 依托单位:
- 学科分类:C1307.作物基因组及遗传学
- 结题年份:1999
- 批准年份:1996
- 项目状态:已结题
- 起止时间:1997-01-01 至1999-12-31
- 项目参与者:杨光宇; 廖林; 陆静梅; 刘玉芝; 马淑时; 朱长甫;
- 关键词:
项目摘要
采用田间自然鉴定、人工接种鉴定等方法,对我国500份野生、半野生大豆进行了为期3年的抗性鉴定,从中发掘出抗灰斑病种质3份,高抗孢囊线虫种质5份,高耐盐种质5份,为大豆育种提供了新的基因源,也为大豆抗病、耐盐机理研究提供了可靠的研究素材。对我国不进化类型大豆进行结构植物学、孢粉学研究表明,大豆次生木质部结构及花粉的形态特征在大豆进化过程中发生了明显的变化,即随着进化程度的提高,维管束的数目和导管数目由少变多,花粉的外壁纹饰由皱块向粗皱块、穿孔演化,明确了大豆各种组织细胞的来源及功能。构建了大豆rbcS基因的系统进化树。根据我国不同纬度、不同进化型大豆的SSR、AFLP、RAPD的综合分析,探讨了大豆的分类问题。
结项摘要
项目成果
期刊论文数量(0)
专著数量(0)
科研奖励数量(0)
会议论文数量(0)
专利数量(0)
数据更新时间:{{ journalArticles.updateTime }}
{{
item.title }}
{{ item.translation_title }}
- DOI:{{ item.doi || "--"}}
- 发表时间:{{ item.publish_year || "--" }}
- 期刊:{{ item.journal_name }}
- 影响因子:{{ item.factor || "--"}}
- 作者:{{ item.authors }}
- 通讯作者:{{ item.author }}
数据更新时间:{{ journalArticles.updateTime }}
{{ item.title }}
- 作者:{{ item.authors }}
数据更新时间:{{ monograph.updateTime }}
{{ item.title }}
- 作者:{{ item.authors }}
数据更新时间:{{ sciAawards.updateTime }}
{{ item.title }}
- 作者:{{ item.authors }}
数据更新时间:{{ conferencePapers.updateTime }}
{{ item.title }}
- 作者:{{ item.authors }}
数据更新时间:{{ patent.updateTime }}
其他文献
其他文献
{{
item.title }}
{{ item.translation_title }}
- DOI:{{ item.doi || "--" }}
- 发表时间:{{ item.publish_year || "--"}}
- 期刊:{{ item.journal_name }}
- 影响因子:{{ item.factor || "--" }}
- 作者:{{ item.authors }}
- 通讯作者:{{ item.author }}
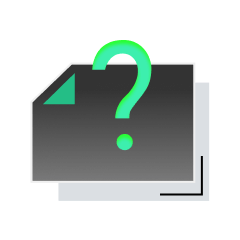
内容获取失败,请点击重试
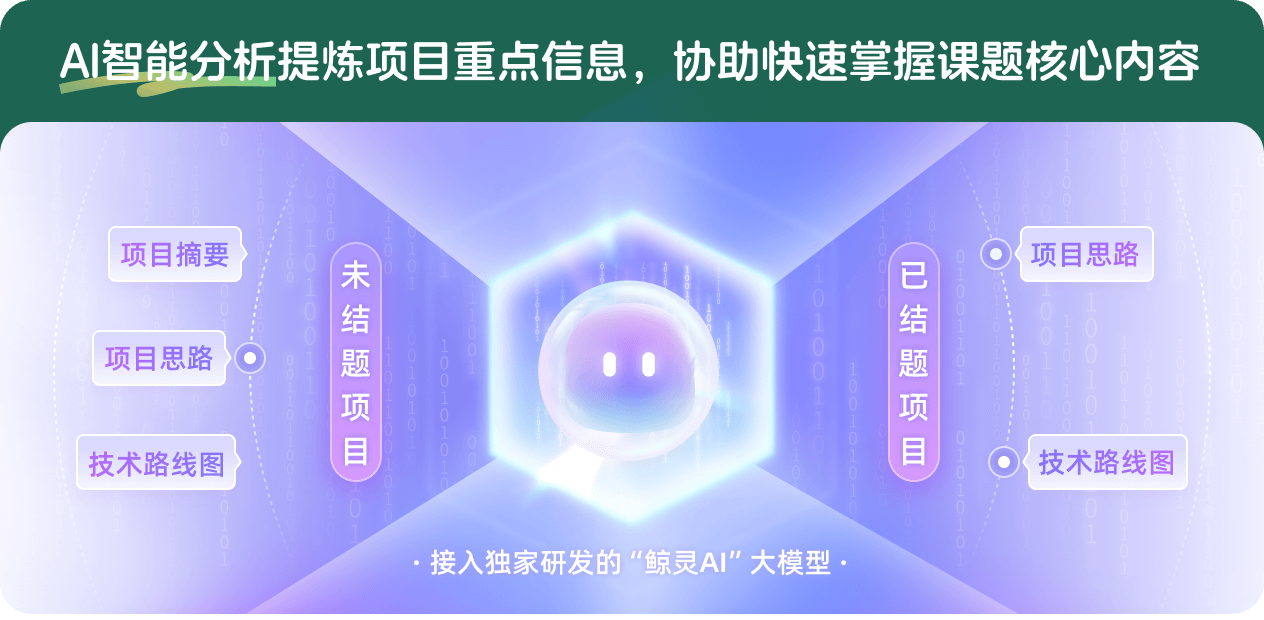
查看分析示例
此项目为已结题,我已根据课题信息分析并撰写以下内容,帮您拓宽课题思路:
AI项目摘要
AI项目思路
AI技术路线图
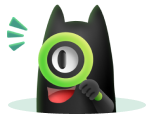
请为本次AI项目解读的内容对您的实用性打分
非常不实用
非常实用
1
2
3
4
5
6
7
8
9
10
您认为此功能如何分析更能满足您的需求,请填写您的反馈:
庄炳昌的其他基金
野生大豆(G.soja)基础生物学研究
- 批准号:39230250
- 批准年份:1992
- 资助金额:35.0 万元
- 项目类别:重点项目
相似国自然基金
{{ item.name }}
- 批准号:{{ item.ratify_no }}
- 批准年份:{{ item.approval_year }}
- 资助金额:{{ item.support_num }}
- 项目类别:{{ item.project_type }}
相似海外基金
{{
item.name }}
{{ item.translate_name }}
- 批准号:{{ item.ratify_no }}
- 财政年份:{{ item.approval_year }}
- 资助金额:{{ item.support_num }}
- 项目类别:{{ item.project_type }}