基于深度学习的冠脉血流动力学指标快速计算方法研究
项目介绍
AI项目解读
基本信息
- 批准号:61771464
- 项目类别:面上项目
- 资助金额:62.0万
- 负责人:
- 依托单位:
- 学科分类:F0125.医学信息检测与处理
- 结题年份:2021
- 批准年份:2017
- 项目状态:已结题
- 起止时间:2018-01-01 至2021-12-31
- 项目参与者:高智凡; 吴丹; 赵屾; 杨玉洁; 刘欣; 夏玉发; 董子刚; sandeep pirbhulal; 樊小毛;
- 关键词:
项目摘要
Fractional flow reserve (FFR) is a technique used in coronary catheterization to measure pressure differences across a coronary artery stenosis (narrowing, usually due to atherosclerosis) to determine the likelihood that the stenosis impedes oxygen delivery to the heart muscle (myocardial ischemia), but it is expensive and invasive. Therefore, it is of great demand to develop a noninvasive technique in health informatics to calculate the FFR. The rapid development of artificial intelligence technology brings new solutions to the intelligent processing of medical data, especially the rapid development of deep learning technology, so that intelligent medical diagnosis becomes more and more possibility, but the use of deep learning technology for coronary artery disease diagnosis is still rare. This project first generates a large number of reliable training samples by computer fluid simulation technology, which is used for the training of the deep learning algorithm to establish the correspondence between the vascular morphological features and the vascular centerline pressure distribution. After reconstructing the patient's coronary structure from the noninvasive CT coronary angiography data, the trained deep learning algorithm can quickly calculate myocardial FFR score in the region of interest. The effectiveness and accuracy of our noninvasive framework will be evaluated through clinical trials finally.
心肌血流储备分数是基于冠脉内压力测量获得的评价冠脉病变生理功能的金标准,即在心肌最大充血状态下冠脉狭窄病变远端血管内的平均压与病变近端管腔的平均压力比值,它需要通过有创介入测量才能得到,因此无创测量FFR指标一直信息科学和心血管疾病交叉领域的研究热点。人工智能技术的快速发展给医学数据智能处理带来新的解决思路,特别是深度学习技术的快速发展,让医学辅助智能诊断变得越来越有可能性,然而使用深度学习技术进行冠脉血管异常结构无创精准诊断却是一个较为空白的研究领域。该项目首先通过计算机流体仿真技术生成大量的可靠训练样本,用于深度学习器的训练来建立血管形态特征和血管中心线压力分布的对应关系,然后从无创CT冠脉造影数据重建病人个体精准的冠脉血管三维结构,使用训练好的深度学习器来快速计算感兴趣区域的心肌血流储备分数,最后通过临床试验来验证该项技术的有效性和准确性。
结项摘要
冠心病的发病原因是冠状动脉狭窄引起的血流供血不足,从而造成心肌梗死等常见冠心病。根据本项目的国内外研究现状及发展动态分析,我们选择了一种流体力学机制指标,冠状动脉血流储备分数(FFR),来作为本项目的临床应用实例。运用多尺度仿真技术模拟冠状动脉内血流场(心脏先验知识),且从病人冠状动脉CTA上鲁棒重建冠状动脉解剖结构(个体测量信息),然后研究主动推理深度学习策略(优化算法)从仿真数据学习流体力学和血管形态之间的映射关系,并运用到计算患者冠脉血流动力学参数上,即是实现无创计算血流储备分数来精准评估动脉狭窄处供血能力。为了能够让本项目的人工智能方法学习到疾病机制,我们跳出之前的常规研究思路,不在使用临床医生标注的医学影像数据来做训练数据,而是先探索心血管疾病中的冠心病发病机制理论,这将为本项目打下扎实理论基础。然后我们采取的研究思路是将冠脉三维解剖结构和血管内流体力学表现定义为三维时空行为,选择双向时间递归神经网络来学习冠脉三维解剖结构和血管内中心线上流体压力分布的耦合机制,也就是牛顿第二定律所描述的血液流场在冠状动脉中的时空变化规律,从而能够实时快速计算出血流储备分数。 为了能够保证本项目研究成果的有效性,我们设计了临床双盲对比实验来完成本项目研究成果的验证。
项目成果
期刊论文数量(20)
专著数量(0)
科研奖励数量(0)
会议论文数量(0)
专利数量(1)
Salient Object Detection in the Distributed Cloud-Edge Intelligent Network
分布式云边智能网络中的显着目标检测
- DOI:10.1109/mnet.001.1900260
- 发表时间:2020-03-01
- 期刊:IEEE NETWORK
- 影响因子:9.3
- 作者:Gao, Zhifan;Zhang, Heye;de Albuquerque, Victor Hugo C.
- 通讯作者:de Albuquerque, Victor Hugo C.
Learning the implicit strain reconstruction in ultrasound elastography using privileged information
使用特权信息学习超声弹性成像中的隐式应变重建
- DOI:10.1016/j.media.2019.101534
- 发表时间:2019-12-01
- 期刊:MEDICAL IMAGE ANALYSIS
- 影响因子:10.9
- 作者:Gao, Zhifan;Wu, Sitong;Li, Shuo
- 通讯作者:Li, Shuo
A novel machine-learning algorithm to estimate the position and size of myocardial infarction for MRI sequence
一种新颖的机器学习算法,用于估计 MRI 序列心肌梗塞的位置和大小
- DOI:10.1007/s00607-018-0675-9
- 发表时间:2018-11
- 期刊:Computing
- 影响因子:3.7
- 作者:Xu Chenchu;Xu Lin;Zhang Heye;Zhang Yanping;Du Xiuquan;Li Shuo
- 通讯作者:Li Shuo
Evaluation of fractional flow reserve in patients with stable angina: can CT compete with angiography?
稳定型心绞痛患者血流储备分数的评估:CT 能否与血管造影竞争?
- DOI:10.1007/s00330-019-06023-z
- 发表时间:2019-07-01
- 期刊:EUROPEAN RADIOLOGY
- 影响因子:5.9
- 作者:Liu, Xin;Wang, Yabin;Huang, Wenhua
- 通讯作者:Huang, Wenhua
An Automatic Cardiac Arrhythmia Classification System With Wearable Electrocardiogram
具有可穿戴心电图的自动心律失常分类系统
- DOI:10.1109/access.2018.2807700
- 发表时间:2018-01-01
- 期刊:IEEE ACCESS
- 影响因子:3.9
- 作者:Xia, Yufa;Zhang, Huailing;Li, Shou
- 通讯作者:Li, Shou
数据更新时间:{{ journalArticles.updateTime }}
{{
item.title }}
{{ item.translation_title }}
- DOI:{{ item.doi || "--"}}
- 发表时间:{{ item.publish_year || "--" }}
- 期刊:{{ item.journal_name }}
- 影响因子:{{ item.factor || "--"}}
- 作者:{{ item.authors }}
- 通讯作者:{{ item.author }}
数据更新时间:{{ journalArticles.updateTime }}
{{ item.title }}
- 作者:{{ item.authors }}
数据更新时间:{{ monograph.updateTime }}
{{ item.title }}
- 作者:{{ item.authors }}
数据更新时间:{{ sciAawards.updateTime }}
{{ item.title }}
- 作者:{{ item.authors }}
数据更新时间:{{ conferencePapers.updateTime }}
{{ item.title }}
- 作者:{{ item.authors }}
数据更新时间:{{ patent.updateTime }}
其他文献
其他文献
{{
item.title }}
{{ item.translation_title }}
- DOI:{{ item.doi || "--" }}
- 发表时间:{{ item.publish_year || "--"}}
- 期刊:{{ item.journal_name }}
- 影响因子:{{ item.factor || "--" }}
- 作者:{{ item.authors }}
- 通讯作者:{{ item.author }}
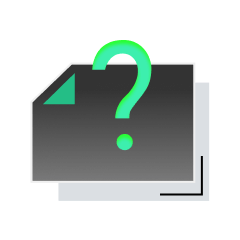
内容获取失败,请点击重试
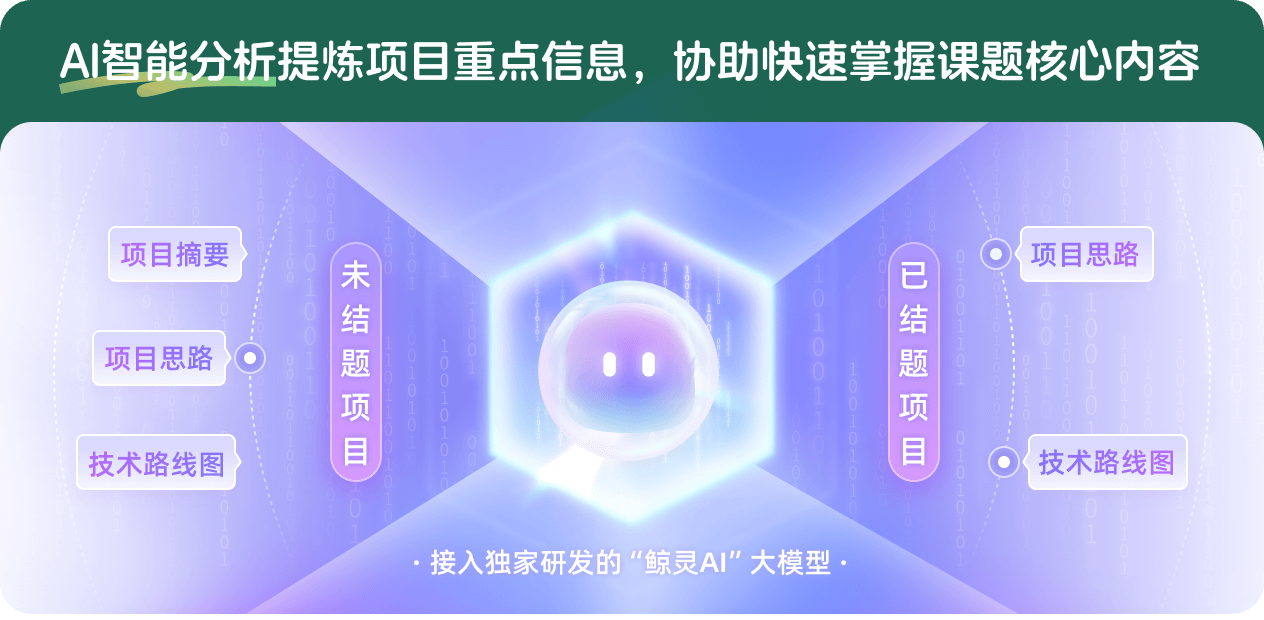
查看分析示例
此项目为已结题,我已根据课题信息分析并撰写以下内容,帮您拓宽课题思路:
AI项目摘要
AI项目思路
AI技术路线图
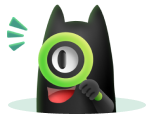
请为本次AI项目解读的内容对您的实用性打分
非常不实用
非常实用
1
2
3
4
5
6
7
8
9
10
您认为此功能如何分析更能满足您的需求,请填写您的反馈:
张贺晔的其他基金
冠状动脉介入手术快速辅助支持系统关键技术研究
- 批准号:
- 批准年份:2022
- 资助金额:53 万元
- 项目类别:面上项目
相似国自然基金
{{ item.name }}
- 批准号:{{ item.ratify_no }}
- 批准年份:{{ item.approval_year }}
- 资助金额:{{ item.support_num }}
- 项目类别:{{ item.project_type }}
相似海外基金
{{
item.name }}
{{ item.translate_name }}
- 批准号:{{ item.ratify_no }}
- 财政年份:{{ item.approval_year }}
- 资助金额:{{ item.support_num }}
- 项目类别:{{ item.project_type }}