基于优化片段搜索和残基接触预测的全新蛋白质结构从头预测算法设计
项目介绍
AI项目解读
基本信息
- 批准号:31670723
- 项目类别:面上项目
- 资助金额:60.0万
- 负责人:
- 依托单位:
- 学科分类:C0504.物理生物学
- 结题年份:2020
- 批准年份:2016
- 项目状态:已结题
- 起止时间:2017-01-01 至2020-12-31
- 项目参与者:熊大鹏; 王童; 毛闻志; 孙芮宁;
- 关键词:
项目摘要
In order to perform their physiological roles, macromolecules like proteins have to fold into their unique native conformations, which renders the importance of the structural studies of proteins. However, the experimental protein structural determinations, albeit well developed, are lagging far behind the derivation of amino acid sequences of proteins. Protein structure prediction, the computational technique that utilizes the amino acid sequences to predict the tertiary structures, can effectively fill the gap between sequencing and structural determination. Unlike the relatively mature protein structure prediction techniques including the homologous modeling and threading, the ab initio prediction methods predict the tertiary structures from the amino acid sequences purely based on first principles and are thus independent of the presence of homologous templates in the structural database. Unfortunately, none of the available ab initio prediction algorithms can predict the protein tertiary structures reliably. In this project, we plan to improve the accuracy and reliability of the present ab initio methods. On one hand, we will optimize the searching of fragments with low homology, which may greatly improve the efficiency of the subsequent fragment assembly that predict the protein structures by randomly assembling the identified fragments. On the other hand, we will further improve the present algorithm for predicting the contacts between amino acid residues, by effectively combining the respective information derived from the structure and sequence databases. The predicted residue contacts can further facilitate the protein structure prediction by reducing the sampling space. Combining the above two improvements, we will develop a novel algorithm for the ab initio protein structure prediction.
蛋白质需要折叠到其天然态构象行使生理功能,因此蛋白质的结构研究非常重要。虽然实验测定蛋白质结构的方法发展很快,但是仍远远落后于氨基酸序列测定的速度。蛋白质结构预测通过理论计算,根据氨基酸序列预测三级结构,因此能有效地填补结构和序列测定间的鸿沟。在蛋白质结构预测方法中,不同于较为成熟的同源建模法和穿线法,从头预测法完全根据物理化学规律进行预测,因此不依赖于结构数据库中是否存在同源模板。但是,目前没有一种从头预测法能可靠地预测蛋白质结构。本项目中,我们计划提高从头预测法的准确度和可靠性。一方面,我们优化远同源片段的搜索,进一步提高使用片段组装法通过拼接这些片段模板来预测蛋白质结构的效率。另一方面,我们通过有效结合得自于结构和序列数据库的信息,优化氨基酸残基间接触的预测。预测所得的残基接触信息可以缩减采样空间,从而进一步辅助结构预测。结合以上改进,我们计划发展一种全新的蛋白质结构从头预测算法。
结项摘要
蛋白质需要折叠到其天然态构象行使生理功能,因此蛋白质的结构研究非常重要。虽然实验测定蛋白质结构的方法发展很快,但是仍远远落后于氨基酸序列测定的速度。蛋白质结构预测通过理论计算,根据氨基酸序列预测三级结构,因此能有效地填补结构和序列测定间的鸿沟。在蛋白质结构预测方法中,不同于较为成熟的同源建模法和穿线法,从头预测法完全根据物理化学规律进行预测,因此不依赖于结构数据库中是否存在同源模板。但是,目前少有从头预测法能可靠地预测蛋白质结构。本项目中,我们开发了多种算法从多个角度提升从头预测方法的准确率和可靠性。特别是DeepFragLib、AmoebaContact+GDFold和GANProDist等三种算法或流程,不仅在算法的设计思路上具有高度的创新性,而且在性能上也至少达到了与领域内主流算法的持平的效果。
项目成果
期刊论文数量(14)
专著数量(0)
科研奖励数量(0)
会议论文数量(0)
专利数量(0)
Molecular dynamics study of ion transport through an open model of voltage-gated sodium channel
通过电压门控钠通道开放模型进行离子传输的分子动力学研究
- DOI:10.1016/j.bbamem.2017.02.003
- 发表时间:2017
- 期刊:Biochimica ET Biophysica Acta-Biomembranes
- 影响因子:3.4
- 作者:Li Yang;Sun Ruining;Liu Huihui;Gong Haipeng
- 通讯作者:Gong Haipeng
Molecular determinants for the thermodynamic and functional divergence of uniporter GLUT1 and proton symporter XylE.
单向转运蛋白 GLUT1 和质子同向转运蛋白 XylE 的热力学和功能差异的分子决定因素
- DOI:10.1371/journal.pcbi.1005603
- 发表时间:2017-06
- 期刊:PLoS computational biology
- 影响因子:4.3
- 作者:Ke M;Yuan Y;Jiang X;Yan N;Gong H
- 通讯作者:Gong H
Simulating the ion permeation and ion selection for a eukaryotic voltage-gated sodium channel Na(V)PaS.
模拟真核电压门控钠通道 NaVPaS 的离子渗透和离子选择
- DOI:10.1007/s13238-018-0522-y
- 发表时间:2018-06
- 期刊:Protein & cell
- 影响因子:21.1
- 作者:Zhang J;Mao W;Ren Y;Sun RN;Yan N;Gong H
- 通讯作者:Gong H
Simulating the Activation of Voltage Sensing Domain for a Voltage-Gated Sodium Channel Using Polarizable Force Field
使用极化力场模拟电压门控钠通道的电压传感域的激活
- DOI:10.1021/acs.jpclett.7000023
- 发表时间:2017
- 期刊:Journal of Physical Chemistry Letters
- 影响因子:5.7
- 作者:Sun Rui Ning;Gong Haipeng
- 通讯作者:Gong Haipeng
Predicting the Real-Valued Inter-Residue Distances for Proteins.
预测蛋白质的实值残基间距离。
- DOI:10.1002/advs.202001314
- 发表时间:2020-10
- 期刊:Advanced science (Weinheim, Baden-Wurttemberg, Germany)
- 影响因子:--
- 作者:Ding W;Gong H
- 通讯作者:Gong H
数据更新时间:{{ journalArticles.updateTime }}
{{
item.title }}
{{ item.translation_title }}
- DOI:{{ item.doi || "--"}}
- 发表时间:{{ item.publish_year || "--" }}
- 期刊:{{ item.journal_name }}
- 影响因子:{{ item.factor || "--"}}
- 作者:{{ item.authors }}
- 通讯作者:{{ item.author }}
数据更新时间:{{ journalArticles.updateTime }}
{{ item.title }}
- 作者:{{ item.authors }}
数据更新时间:{{ monograph.updateTime }}
{{ item.title }}
- 作者:{{ item.authors }}
数据更新时间:{{ sciAawards.updateTime }}
{{ item.title }}
- 作者:{{ item.authors }}
数据更新时间:{{ conferencePapers.updateTime }}
{{ item.title }}
- 作者:{{ item.authors }}
数据更新时间:{{ patent.updateTime }}
其他文献
其他文献
{{
item.title }}
{{ item.translation_title }}
- DOI:{{ item.doi || "--" }}
- 发表时间:{{ item.publish_year || "--"}}
- 期刊:{{ item.journal_name }}
- 影响因子:{{ item.factor || "--" }}
- 作者:{{ item.authors }}
- 通讯作者:{{ item.author }}
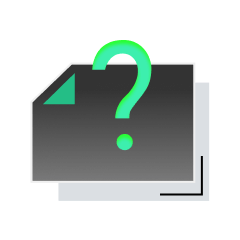
内容获取失败,请点击重试
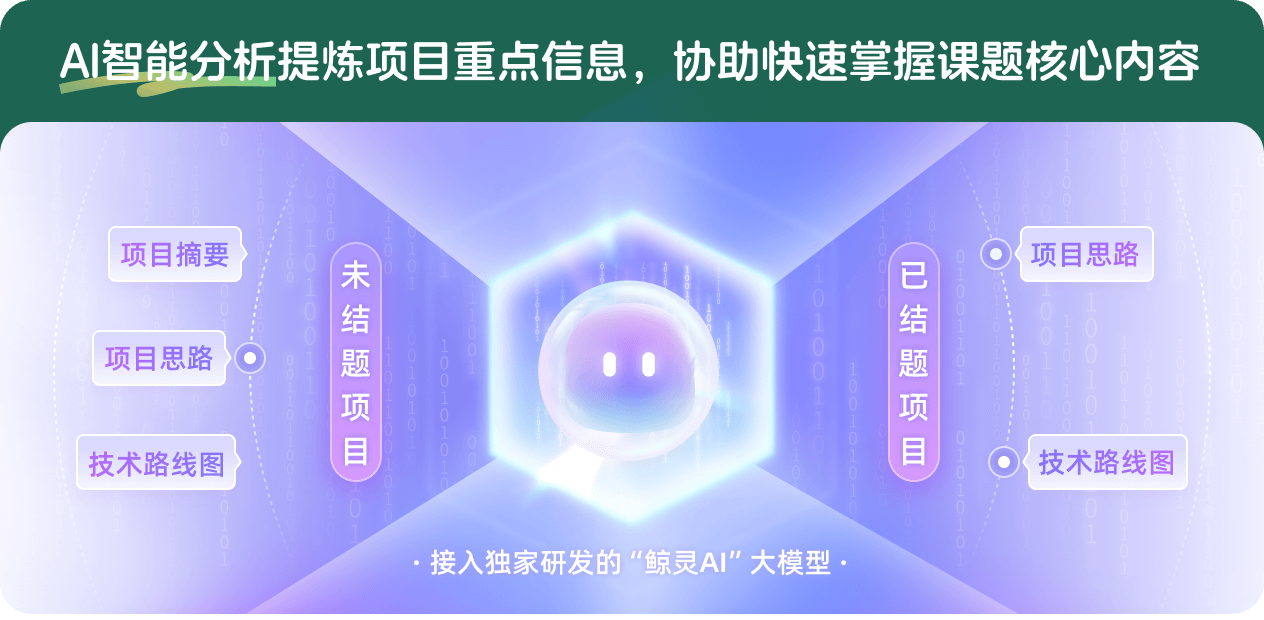
查看分析示例
此项目为已结题,我已根据课题信息分析并撰写以下内容,帮您拓宽课题思路:
AI项目摘要
AI项目思路
AI技术路线图
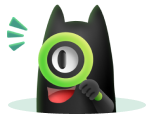
请为本次AI项目解读的内容对您的实用性打分
非常不实用
非常实用
1
2
3
4
5
6
7
8
9
10
您认为此功能如何分析更能满足您的需求,请填写您的反馈:
龚海鹏的其他基金
基于人工智能的蛋白质结构预测算法开发
- 批准号:
- 批准年份:2021
- 资助金额:58 万元
- 项目类别:面上项目
基于人工智能的蛋白质结构预测算法开发
- 批准号:32171243
- 批准年份:2021
- 资助金额:58.00 万元
- 项目类别:面上项目
使用分子模拟研究电压门控钠离子通道的分子机理
- 批准号:31470033
- 批准年份:2014
- 资助金额:30.0 万元
- 项目类别:面上项目
MFS家族膜转运蛋白转运机理的研究
- 批准号:31170674
- 批准年份:2011
- 资助金额:60.0 万元
- 项目类别:面上项目
相似国自然基金
{{ item.name }}
- 批准号:{{ item.ratify_no }}
- 批准年份:{{ item.approval_year }}
- 资助金额:{{ item.support_num }}
- 项目类别:{{ item.project_type }}
相似海外基金
{{
item.name }}
{{ item.translate_name }}
- 批准号:{{ item.ratify_no }}
- 财政年份:{{ item.approval_year }}
- 资助金额:{{ item.support_num }}
- 项目类别:{{ item.project_type }}