基于异构信息融合的实时竞价广告响应预测方法研究
项目介绍
AI项目解读
基本信息
- 批准号:61602131
- 项目类别:青年科学基金项目
- 资助金额:20.0万
- 负责人:
- 依托单位:
- 学科分类:F0211.信息检索与社会计算
- 结题年份:2019
- 批准年份:2016
- 项目状态:已结题
- 起止时间:2017-01-01 至2019-12-31
- 项目参与者:林磊; 刘远超; 徐振; 孙雅铭; 邓丽芳; 刘阳; 周博通;
- 关键词:
项目摘要
Recent years, due to its ability of precisely capturing the three-dimensional interaction, tensor decomposition models have been adopted to learn the three-dimensional interaction between user, context and ad in addressing response prediction for real time bidding. But real time bidding confronts with much sparser data than both recommender system and sponsored search. In order to fight with severe data sparsity occurred in response prediction, we try to integrate heterogeneous information available on demand-side platform into tensor decomposition models. This project focuses on how to integrate heterogeneous information with various properties into tensor decomposition models and seek the integrated solutions and frameworks. At first, we divide all information available for DSP into five types: general object features, hierarchy and cluster information, temporal information, click and conversion response information. Then, according to different type of information, we analyze their characteristics and functions in prediction process and design different strategies to integrate them into tensor decomposition models. Finally, we research the integrated solutions and frameworks which can incorporate all kinds of information and improve the prediction performance both on regression and on ranking. The final goal is to outperform the state of art methods on response prediction for real time bidding.
为了有效地挖掘用户、上下文和广告之间的三维交互关系,张量分解模型开始被用于解决实时竞价广告响应预测问题。然而实时竞价广告响应预测面临严峻的数据稀疏问题,尤其是广告转化率预测,单纯地只依靠融合某类或某些信息不能有效地解决严峻的数据稀疏问题,只有同时综合利用各种各样的异质、异构信息共同应对。本项目重点研究如何将各种各样的异构信息融合集成到张量分解模型中缓解数据稀疏问题,提升模型预测的准确性和可靠性。首先,我们根据可以获得并利用的信息的类型、性质、结构、表示方式及其在解决数据稀疏问题中所起的作用等将所有信息分成五个类别,普通对象特征、层次和聚类信息、时间序列信息、点击与转化反馈信息及全局特征。然后,我们针对不同类型信息的性质和特点,基于张量分解模型,研究不同的融合方案和方法。最后,我们研究同时能够融合五类不同信息的综合框架和解决方案,并通过排序和回归组合优化同时提升基于排序和回归的性能指标。
结项摘要
为了有效地挖掘用户、上下文和广告之间的三维交互关系,张量分解模型开始被用于解决实时竞价广告响应预测问题。然而实时竞价广告响应预测面临严峻的数据稀疏问题,尤其是广告转化率预测,单纯地只依靠融合某类或某些信息不能有效地解决严峻的数据稀疏问题,只有同时综合利用各种各样的异质、异构信息共同应对。本项目重点研究如何将各种各样的异构信息融合集成到张量分解模型中缓解数据稀疏问题,提升模型预测的准确性和可靠性。.首先,本项目提出了三元组排序优化算法,解决了同时利用历史反馈数据中的点击信息和转化信息的问题,更好地提升了转化预测的性能。此算法还具有很好的扩展性,能够扩展应用到其它的三分类排序问题,能更好地解决三分类排序问题。其次,本项目提出了回归与三分类排序组合优化策略,在预测广告反馈时同时考虑了三分类排序损失和预估值损失,有效地提升了预测性能,此方法也具有扩展性,可以用于具有相似性质的问题中。最后,本项目提出了异构信息综合融合方案解决了普通对象特征、层次聚类特征、全局特征和用户反馈特征同时融合的问题。本项目在重要期刊或会议上共发表论文15篇;其中发表国际顶级会议1篇,国际重要会议3篇,发表SCI期刊4篇。培养博士研究生1名,硕士研究生3名。作为成果的一部分获得黑龙江省自然科学技术奖三等奖一项,获得黑龙江省高校科学技术奖三等奖一项。.本项目研究成果可以应用于在线预测广告点击或转化率的模型,模型融合方法简单,实用性好。另外,本项目提出的三分类排序优化方法以及回归与三分类排序组合优化策略都有较好的扩展应用价值,可以应用于所有的三分类排序问题中,例如在情感分析中,用户对产品的评价有时不能明确地分为正向和负向,这时可以将评价从极性上分为正向,负向和中性三类,然后转化为三分类问题进行预测,这将是我们未来的研究工作。
项目成果
期刊论文数量(6)
专著数量(0)
科研奖励数量(2)
会议论文数量(9)
专利数量(0)
Content-Oriented User Modeling for Personalized Response Ranking in Chatbots
聊天机器人中个性化响应排名的面向内容的用户建模
- DOI:10.1109/taslp.2017.2763243
- 发表时间:2018
- 期刊:IEEE/ACM Transactions on Audio Speech & Language Processing
- 影响因子:--
- 作者:Bingquan Liu;Zhen Xu;Chengjie Sun;Baoxun Wang
- 通讯作者:Baoxun Wang
Modelling Context with Neural Networks for Recommending Idioms in Essay Writing
用神经网络对上下文进行建模,以推荐论文写作中的习语
- DOI:--
- 发表时间:2017
- 期刊:Neurocomputing
- 影响因子:6
- 作者:Yuanchao Liu;Bingquan Liu;Lili Shan;Xin Wang
- 通讯作者:Xin Wang
基于异构信息融合的广告响应预测方法
- DOI:10.1360/n112017-00157
- 发表时间:2019
- 期刊:中国科学
- 影响因子:--
- 作者:单丽莉;林磊;孙承杰
- 通讯作者:孙承杰
Optimizing ranking for response prediction via triplet-wise learning from historical feedback
通过历史反馈的三重学习来优化响应预测的排名
- DOI:10.1007/s13042-016-0558-3
- 发表时间:2017
- 期刊:International Journal of Machine Learning and Cybernetics
- 影响因子:5.6
- 作者:Lili Shan;Lei Lin;Chengjie Sun;Xiaolong Wang;Bingquan Liu
- 通讯作者:Bingquan Liu
InsunKBQA:一个基于知识库的问答系统
- DOI:--
- 发表时间:2017
- 期刊:智能计算机与应用
- 影响因子:--
- 作者:周博通;孙承杰;林 磊;刘秉权
- 通讯作者:刘秉权
数据更新时间:{{ journalArticles.updateTime }}
{{
item.title }}
{{ item.translation_title }}
- DOI:{{ item.doi || "--"}}
- 发表时间:{{ item.publish_year || "--" }}
- 期刊:{{ item.journal_name }}
- 影响因子:{{ item.factor || "--"}}
- 作者:{{ item.authors }}
- 通讯作者:{{ item.author }}
数据更新时间:{{ journalArticles.updateTime }}
{{ item.title }}
- 作者:{{ item.authors }}
数据更新时间:{{ monograph.updateTime }}
{{ item.title }}
- 作者:{{ item.authors }}
数据更新时间:{{ sciAawards.updateTime }}
{{ item.title }}
- 作者:{{ item.authors }}
数据更新时间:{{ conferencePapers.updateTime }}
{{ item.title }}
- 作者:{{ item.authors }}
数据更新时间:{{ patent.updateTime }}
其他文献
文本分类中特征选择方法的比较与改进
- DOI:--
- 发表时间:--
- 期刊:哈尔滨工业大学学报(增刊)
- 影响因子:--
- 作者:孙承杰;单丽莉;刘秉权
- 通讯作者:刘秉权
Modelling context with neural networks for recommending idioms in essay writing
使用神经网络对上下文进行建模,以推荐论文写作中的习语
- DOI:--
- 发表时间:2018
- 期刊:Neurocomputing
- 影响因子:6
- 作者:刘远超;刘秉权;单丽莉;王鑫
- 通讯作者:王鑫
Predicting ad click-through rates via feature-based fully coupled interaction tensor factorization
通过基于特征的全耦合交互张量分解预测广告点击率
- DOI:10.1016/j.elerap.2016.01.004
- 发表时间:2016
- 期刊:Electronic Commerce Research and Applications
- 影响因子:6
- 作者:单丽莉
- 通讯作者:单丽莉
其他文献
{{
item.title }}
{{ item.translation_title }}
- DOI:{{ item.doi || "--" }}
- 发表时间:{{ item.publish_year || "--"}}
- 期刊:{{ item.journal_name }}
- 影响因子:{{ item.factor || "--" }}
- 作者:{{ item.authors }}
- 通讯作者:{{ item.author }}
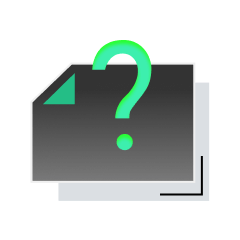
内容获取失败,请点击重试
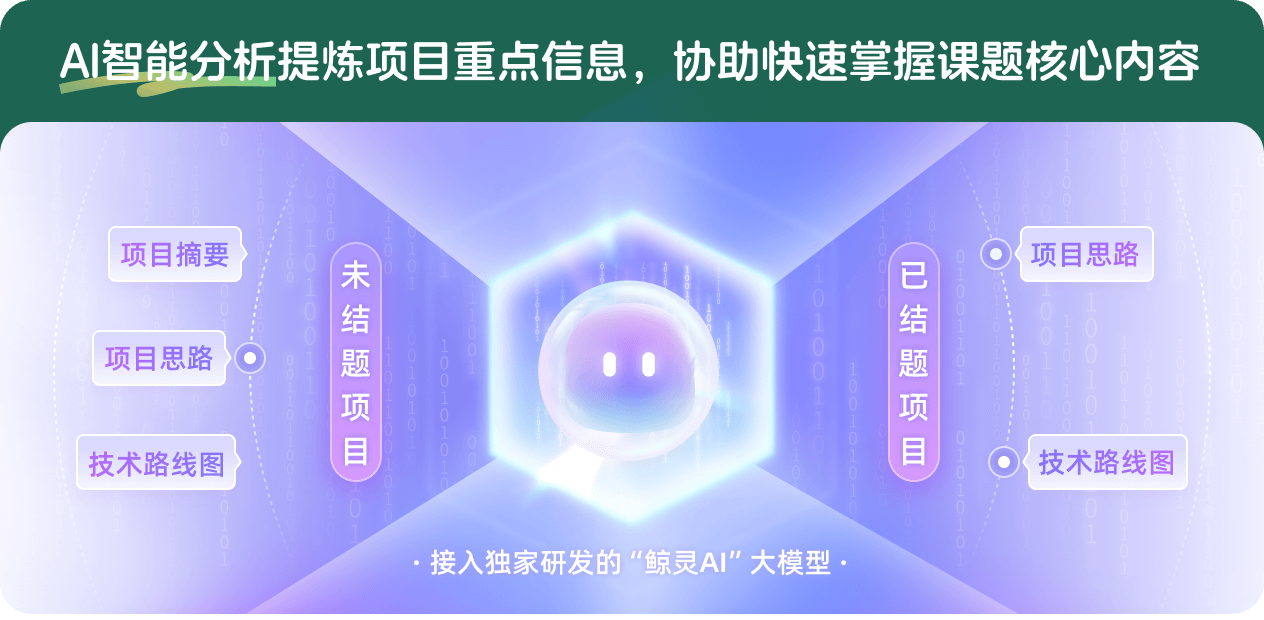
查看分析示例
此项目为已结题,我已根据课题信息分析并撰写以下内容,帮您拓宽课题思路:
AI项目摘要
AI项目思路
AI技术路线图
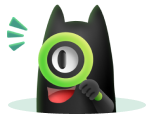
请为本次AI项目解读的内容对您的实用性打分
非常不实用
非常实用
1
2
3
4
5
6
7
8
9
10
您认为此功能如何分析更能满足您的需求,请填写您的反馈:
相似国自然基金
{{ item.name }}
- 批准号:{{ item.ratify_no }}
- 批准年份:{{ item.approval_year }}
- 资助金额:{{ item.support_num }}
- 项目类别:{{ item.project_type }}
相似海外基金
{{
item.name }}
{{ item.translate_name }}
- 批准号:{{ item.ratify_no }}
- 财政年份:{{ item.approval_year }}
- 资助金额:{{ item.support_num }}
- 项目类别:{{ item.project_type }}