基于Deep-learning的三江源区冰川监测动态识别技术研究
项目介绍
AI项目解读
基本信息
- 批准号:51769027
- 项目类别:地区科学基金项目
- 资助金额:38.0万
- 负责人:
- 依托单位:
- 学科分类:E0901.工程水文与水资源利用
- 结题年份:2021
- 批准年份:2017
- 项目状态:已结题
- 起止时间:2018-01-01 至2021-12-31
- 项目参与者:石爽; 柏文文; 朱晓梅; 白晓兰; 强安丰; 赵杰;
- 关键词:
项目摘要
With the glacier retreats in the three-river headwater region, the water supply in the downstream and the ecological surrounding environments will be affected, and the risk of flooding due to collapse lake will also increase. Therefore, it should be vigorously strengthened to monitor glacial changes in the region. In addition to the field survey, the remote sensing image analysis technology has been an important tool for glacier monitoring. However, affected by various factors, reliability and accurate degree of the existing glacier recognition methods is low for remote sensing image analysis. Moreover, in most cases human interventions are required and so the degree of automation is not high, resultantly it is difficult to realize accurate estimations of the glacier area and volume, in turn, the impaction anticipation and countermeasures of the glacier change will be affected. In order to overcome the defects of the existing glacier recognition technologies, continuously improve the indicators, this application will undertake researches on dynamic recognition technologies about the glacier monitoring in the three-river headwater region, through fusion of region and edge, dynamic and static features, abstract and concrete features of the sensing images, the glacier target detection and recognition task will be effectively implemented by using of the deep-learning method based on convolution neural-network algorithms and BP neural-network for classification. In the future, the glacier dynamic recognition technologies are applied, and it will enhance greatly level of glacier monitoring.
随着三江源区冰川的逐渐退缩,该地区的下游淡水供给及周围生态环境不但受到影响,而且发生溃湖洪灾的风险也将增大。因此,应当大力加强对该地区冰川变化的监测工作。除了实地勘测之外,目前遥感影像分析是监测冰川动态非常重要的技术手段。然而,受多种因素影响,在遥感影像分析中现有的冰川识别技术可靠性、准确程度偏低,多数情况下仍需人工干预自动化程度不高,因而难以实现冰川面积与体量等的准确估计,进而影响冰川变化的预判及对策的制定。为了克服现有冰川识别技术的缺陷、不断提高技术的性能指标,本申请将开展三江源区冰川监测动态识别技术研究,在融合图像区域与边缘特征、动态与静态特征、抽象与具体特征的基础上,利用基于卷积神经网络的深度学习算法和BP神经网络分类算法实现对冰川目标的检测与识别任务。本申请所研究的关键技术得到应用后,将大幅提高三江源区冰川监测水平。
结项摘要
冰川监测是生态环境监测的一项重要内容。它主要通过监测冰川面积、体积等变化来反映生态环境的变化。基于深度学习遥感图像语义分割已经引起了生态环境监测领域研究人员的普遍关注,并取得了诸多研究成果。本项目课题在深入探讨和分析深度学习冰川监测应用已有研究结果的基础上,以提高已有冰川监测遥感图像识别和分析算法性能为目标,就冰川监测存在的可用于冰川遥感图像语义分割的数据集少、冰水难以区分和阴影区域下冰川难以识别等问题展开研究。.(1)利用格拉丹冬冰川及其周围冰川群遥感影像数据,对9种典型的冰川识别方法进行对比试验,并采用基于混淆矩阵进行定量评价,结果发现神经网络分类方法的总体分类精度为99.372%,提取冰川效果最好。因而认为,借助于深度学习网络建立冰川提取模型,进行深度训练将是未来的一个研究方向;单一冰川提取方法不能够解决冰川提取中的所有问题,结合多种技术方法的优点来提取冰川也是一个比较好的方法。.(2)数据集的质量和数量对于深度学习来说至关重要,目前可用于冰川语义分割的公开遥感数据集较少。为更好的研究利用语义分割理论进行冰川分割,面对缺乏标注好的冰川数据集问题,自行制作了针对冰川识别问题研究所需要的两套数据集:带阴影区域的冰川数据集和冰水数据集。.(3)针对遥感图像受光照、山体高度和成像角度等因素影响形成目标阴影区域,影响冰川识别效果的问题,提出一种增强多尺度特征融合的网络架构UPSPNet。该方法通过融合多尺度特征信息,丰富特征图提取的冰川特征,从而提高网络架构识别冰川的能力(包括阴影区域下的冰川)。实验结果表明,所提方法的平均像素准确率达到95.84﹪,其平均交并比达到92.79﹪。相对于未融合金字塔池化结构的网络架构,平均像素准确率提高0.61﹪,平均交并比提高0.92﹪,能够更好地识别出阴影下的冰川区域。.(4)针对冰川、积雪和水体对波段的反射率相近,利用传统冰川识别方法区分效果差的问题,提出一种融合注意力模块的冰水分割架构ResUNet-dan。其中,注意力模块可以增强相似特征之间的关联性,降低无关特征之间的连接性,增强图像中的上下文信息;UNet架构中的跳跃连接结构便于融合编码层特征信息和解码层特征信息,融合全局信息和局部信息。该方法结合两者能够丰富特征图信息和增强特征图表现力,从而提高冰川分割的准确率,有利于有效分割冰川和水体。实验结果表
项目成果
期刊论文数量(2)
专著数量(0)
科研奖励数量(0)
会议论文数量(1)
专利数量(0)
基于改进U-Net 网络的复杂背景下冰川遥感图像检测方法
- DOI:--
- 发表时间:--
- 期刊:应用基础与工程科学学报
- 影响因子:--
- 作者:张大奇;范慧颖;康宝生;高健;李铁键
- 通讯作者:李铁键
基于遥感图像的山地冰川识别方法对比
- DOI:10.13928/j.cnki.wrahe.2020.05.006
- 发表时间:2020
- 期刊:水利水电技术
- 影响因子:--
- 作者:范慧颖;董武;康宝生;张大奇
- 通讯作者:张大奇
数据更新时间:{{ journalArticles.updateTime }}
{{
item.title }}
{{ item.translation_title }}
- DOI:{{ item.doi || "--"}}
- 发表时间:{{ item.publish_year || "--" }}
- 期刊:{{ item.journal_name }}
- 影响因子:{{ item.factor || "--"}}
- 作者:{{ item.authors }}
- 通讯作者:{{ item.author }}
数据更新时间:{{ journalArticles.updateTime }}
{{ item.title }}
- 作者:{{ item.authors }}
数据更新时间:{{ monograph.updateTime }}
{{ item.title }}
- 作者:{{ item.authors }}
数据更新时间:{{ sciAawards.updateTime }}
{{ item.title }}
- 作者:{{ item.authors }}
数据更新时间:{{ conferencePapers.updateTime }}
{{ item.title }}
- 作者:{{ item.authors }}
数据更新时间:{{ patent.updateTime }}
其他文献
其他文献
{{
item.title }}
{{ item.translation_title }}
- DOI:{{ item.doi || "--" }}
- 发表时间:{{ item.publish_year || "--"}}
- 期刊:{{ item.journal_name }}
- 影响因子:{{ item.factor || "--" }}
- 作者:{{ item.authors }}
- 通讯作者:{{ item.author }}
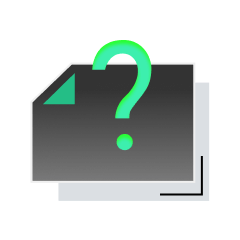
内容获取失败,请点击重试
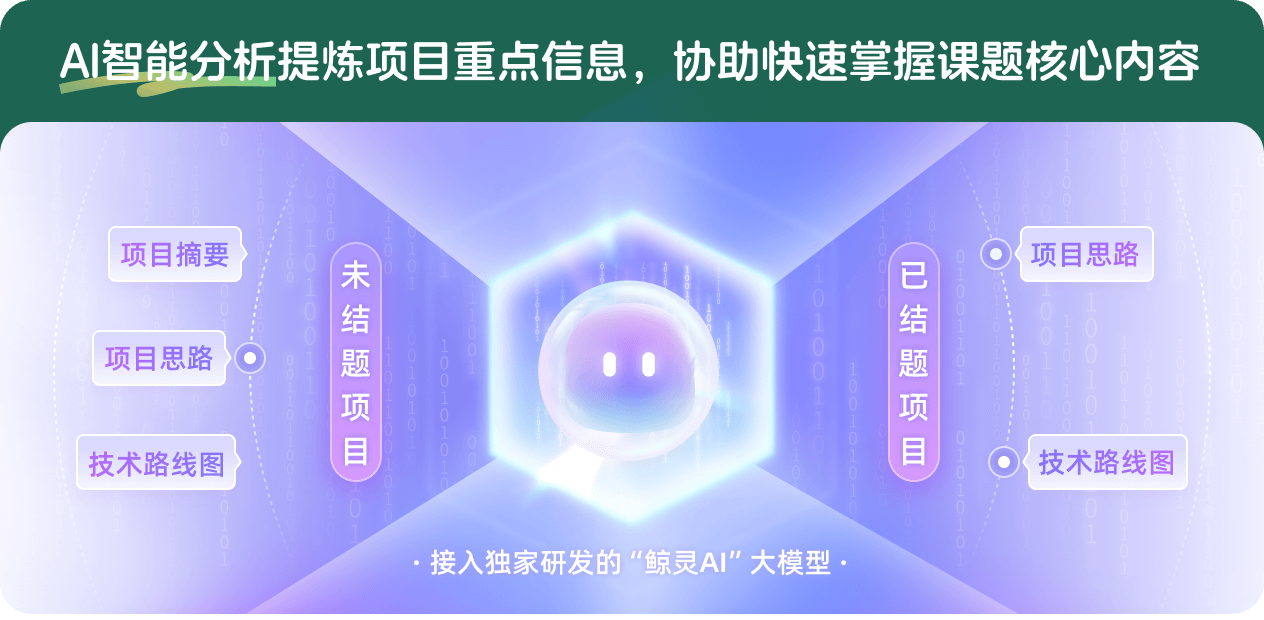
查看分析示例
此项目为已结题,我已根据课题信息分析并撰写以下内容,帮您拓宽课题思路:
AI项目摘要
AI项目思路
AI技术路线图
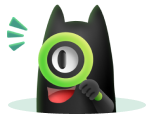
请为本次AI项目解读的内容对您的实用性打分
非常不实用
非常实用
1
2
3
4
5
6
7
8
9
10
您认为此功能如何分析更能满足您的需求,请填写您的反馈:
相似国自然基金
{{ item.name }}
- 批准号:{{ item.ratify_no }}
- 批准年份:{{ item.approval_year }}
- 资助金额:{{ item.support_num }}
- 项目类别:{{ item.project_type }}
相似海外基金
{{
item.name }}
{{ item.translate_name }}
- 批准号:{{ item.ratify_no }}
- 财政年份:{{ item.approval_year }}
- 资助金额:{{ item.support_num }}
- 项目类别:{{ item.project_type }}