地面LiDAR探测滑坡体小形变场的关键技术研究
项目介绍
AI项目解读
基本信息
- 批准号:41801398
- 项目类别:青年科学基金项目
- 资助金额:24.7万
- 负责人:
- 依托单位:
- 学科分类:D0115.测量与地图学
- 结题年份:2021
- 批准年份:2018
- 项目状态:已结题
- 起止时间:2019-01-01 至2021-12-31
- 项目参与者:李大安; 李德伟; 陈玉兴;
- 关键词:
项目摘要
The small surface deformation is one precursor signal of landslide, and the detection of three-dimensional deformation field with high resolution plays a significant role in disaster monitoring and prewarning. Ground-based LiDAR has become a new means for monitoring the small deformation due to its ability to obtain three-dimensional point clouds with high accuracy and density. However, there are still several problems that need to be solved urgently in the processing of point clouds from ground-based LiDAR in a landslide scene, such as big difficulty, low accuracy and low degree of automation. The project will be carried out following the outline of “Registration – Ground Points Extraction – Deformation Detection”. Three novel methods are considered to address the technical challenges, including the assessment of the reliability of each registration primitive, the robust extraction of ground points, and the computation of nonlinear deformations with a high accuracy. Firstly, a dynamic model fusing multiple weights is proposed to better estimate the reliability of each registration primitive, and to match point clouds with different geo-references. Then, a Conditional Random Field based on a Transfer Random Forest is developed to robustly extract ground points. Finally, the project proposes an elastic deformation energy model with constraints of terrain features, and to calculating three-dimensional non-linear small deformation by the finite element method. By these methods, the project aims to establish a methodology for precisely processing of point clouds, and to robustly detect the small deformation in a landslide scene. The outcome of this project is supposed to improve the ability of ground-based LiDAR to monitor the landslide, and to provide a good foundation for the study of disaster mechanism and disaster prevention and mitigation.
地表小形变是滑坡灾害前兆信号之一,精细探测其三维小形变场对灾害监测预警具有重要意义。地面LiDAR凭借其三维点云的高精度、高密度特性,已成为滑坡体小形变场精细监测的新手段。目前,滑坡场景地面LiDAR点云处理还存在难度大、精度差、自动化程度低等不足,是当前亟待解决的问题。本项目以“点云配准—>地面点云提取—>形变场探测”为研究主线,针对配准基元可靠性评估质量低、地面点云稳健提取难、非线性形变计算精度差等技术瓶颈,研究多类型权重动态组合模型,高质量评估基元可靠性,实现点云高精度配准;发展迁移随机森林的条件随机场模型,稳健提取地面点云;提出地形特征约束的弹性形变能量模型,并采用有限元方法高精度解算三维非线性小形变场,从而建立一套滑坡场景地面LiDAR点云高精度处理方法,实现滑坡小形变场稳健探测。本项目研究成果有望提高地面LiDAR监测滑坡灾害的能力,为灾害机理研究及防灾减灾提供重要的技术支撑。
结项摘要
地表小形变是滑坡灾害前兆信号之一,如何准确探测滑坡体小形变场,对灾害监测预警具有重要意义。地面LiDAR通过获取滑坡区域多时相高精度、高密度三维点云,能够实现厘米级甚至更小的形变场探测,受到国内外学者的广泛关注。其中,地面LiDAR点云高质量获取、多时相点云配准、地面点云提取和高精度形变场计算是从点云中探测小形变场的重要关键,也是地面LiDAR滑坡监测中面临的重要问题。因此,针对以上问题,本项目开展了系列研究,其中重要研究进展如下:1)研究了一种顾及点对可靠性的多时相地面LiDAR点云高精度配准方法,通过构建点对可靠性多类型因子动态评估组合模型,定量确定点对的可靠性程度,有效提高了多时相点云精配准质量;2)发展了一种基于机器学习的滑坡体多时相激光点云滤波分类新方法,通过挖掘缓慢滑坡体多时相地形之间的强相关性,极大地降低了对分类器泛化能力的要求;3)发展了一种自适应局部区域C2M高精度形变场计算方法,有效解决了滑坡场景复杂地形形态和地面LiDAR点云漏洞对形变计算的影响;4)提出了基于多目标优化的滑坡场景地面LiDAR观测网优化方法,进行滑坡地面LiDAR观测网科学布设,以提高地面LiDAR点云采集质量。研究成果突破了地面LiDAR观测网优化、多时相点云配准、多时相点云滤波分类和小形变场计算等关键技术,为实现复杂滑坡场景地面LiDAR形变场高质量探测提供了技术支撑,并在三峡库区大树镇滑坡和川藏铁路洛隆滑坡进行了实验。按照既定的研究计划,项目圆满完成了规定的研究内容。在项目执行中,项目组在Landslides、ISPRS等期刊上发表SCI标注论文4篇,其中3篇中科院1区Top、1篇中科院2区Top,获批软件著作权1项,申请国家发明专利1项,参加国内外学术会议6次,获测绘科技进步奖二等奖1次。
项目成果
期刊论文数量(4)
专著数量(0)
科研奖励数量(1)
会议论文数量(0)
专利数量(1)
A Bidirectional Analysis Method for Extracting Glacier Crevasses from Airborne LiDAR Point Clouds
从机载激光雷达点云中提取冰川裂缝的双向分析方法
- DOI:10.3390/rs11202373
- 发表时间:2019
- 期刊:Remote Sensing
- 影响因子:5
- 作者:Huang Ronggang;Jiang Liming;Wang Hansheng;Yang Bisheng
- 通讯作者:Yang Bisheng
A novel skyline context descriptor for rapid localization of terrestrial laser scans to airborne laser scanning point clouds
一种新颖的天际线上下文描述符,用于将地面激光扫描快速定位到机载激光扫描点云
- DOI:10.1016/j.isprsjprs.2020.04.018
- 发表时间:2020
- 期刊:ISPRS Journal of Photogrammetry and Remote Sensing
- 影响因子:12.7
- 作者:Liang Fuxun;Yang Bisheng;Dong Zhen;Huang Ronggang;Zang Yufu;Pan Yue
- 通讯作者:Pan Yue
An efficient method of monitoring slow-moving landslides with long-range terrestrial laser scanning: a case study of the Dashu landslide in the Three Gorges Reservoir Region, China
远程地面激光扫描监测缓慢移动滑坡的有效方法——以中国三峡库区大树滑坡为例
- DOI:10.1007/s10346-018-1118-6
- 发表时间:2019
- 期刊:Landslides
- 影响因子:6.7
- 作者:Huang Ronggang;Jiang Liming;Shen Xiang;Dong Zhen;Zhou Qiaoli;Yang Bisheng;Wang Hansheng
- 通讯作者:Wang Hansheng
HPM-TDP: An efficient hierarchical PatchMatch depth estimation approach using tree dynamic programming
HPM-TDP:一种使用树动态规划的高效分层 PatchMatch 深度估计方法
- DOI:10.1016/j.isprsjprs.2019.06.015
- 发表时间:2019
- 期刊:ISPRS Journal of Photogrammetry and Remote Sensing
- 影响因子:12.7
- 作者:Tian Mao;Yang Bisheng;Chen Chi;Huang Ronggang;Huo Liang
- 通讯作者:Huo Liang
数据更新时间:{{ journalArticles.updateTime }}
{{
item.title }}
{{ item.translation_title }}
- DOI:{{ item.doi || "--"}}
- 发表时间:{{ item.publish_year || "--" }}
- 期刊:{{ item.journal_name }}
- 影响因子:{{ item.factor || "--"}}
- 作者:{{ item.authors }}
- 通讯作者:{{ item.author }}
数据更新时间:{{ journalArticles.updateTime }}
{{ item.title }}
- 作者:{{ item.authors }}
数据更新时间:{{ monograph.updateTime }}
{{ item.title }}
- 作者:{{ item.authors }}
数据更新时间:{{ sciAawards.updateTime }}
{{ item.title }}
- 作者:{{ item.authors }}
数据更新时间:{{ conferencePapers.updateTime }}
{{ item.title }}
- 作者:{{ item.authors }}
数据更新时间:{{ patent.updateTime }}
其他文献
利用目标区域拓扑关系图提取建筑物点云
- DOI:10.13203/j.whugis20160112
- 发表时间:2017
- 期刊:武汉大学学报信息科学版
- 影响因子:--
- 作者:黄荣刚;杨必胜;李健平;田茂;梁新美
- 通讯作者:梁新美
三维激光扫描点云数据处理研究进展、挑战与趋势
- DOI:--
- 发表时间:2017
- 期刊:测绘学报
- 影响因子:--
- 作者:杨必胜;梁福逊;黄荣刚
- 通讯作者:黄荣刚
车载探地雷达地下目标实时探测法
- DOI:10.11947/j.agcs.2020.20190293
- 发表时间:2020
- 期刊:测绘学报
- 影响因子:--
- 作者:杨必胜;宗泽亮;陈驰;孙文鹿;米晓新;吴唯同;黄荣刚
- 通讯作者:黄荣刚
其他文献
{{
item.title }}
{{ item.translation_title }}
- DOI:{{ item.doi || "--" }}
- 发表时间:{{ item.publish_year || "--"}}
- 期刊:{{ item.journal_name }}
- 影响因子:{{ item.factor || "--" }}
- 作者:{{ item.authors }}
- 通讯作者:{{ item.author }}
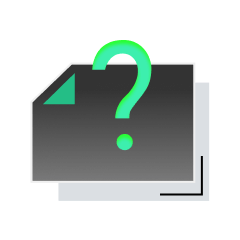
内容获取失败,请点击重试
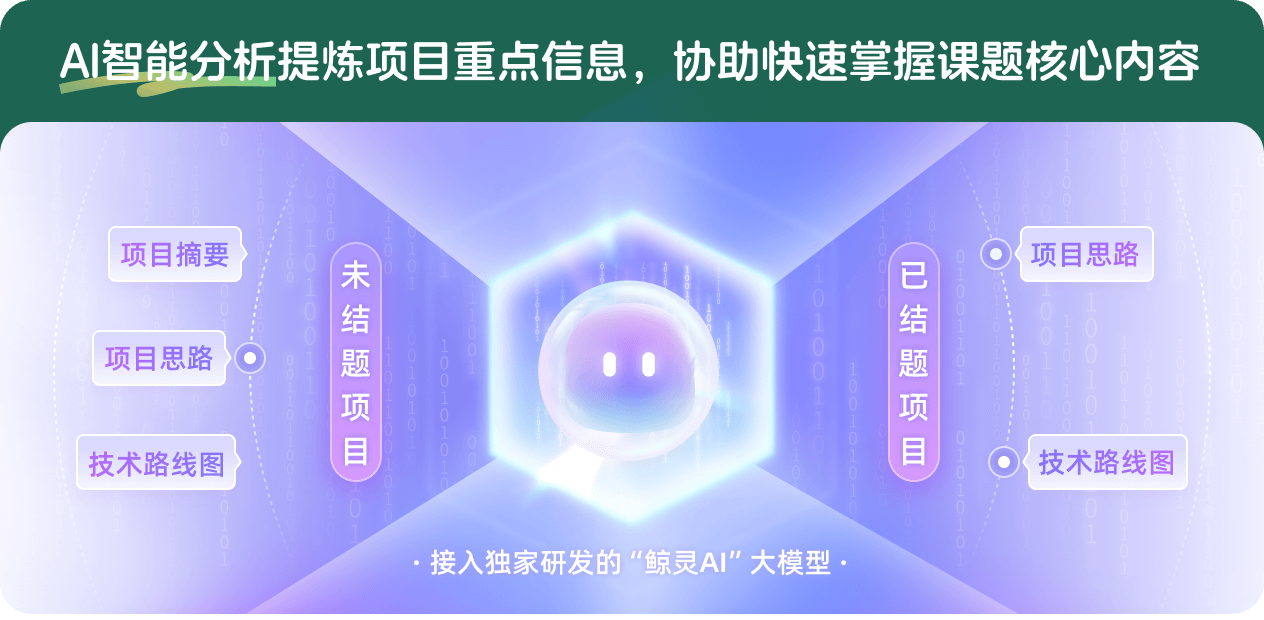
查看分析示例
此项目为已结题,我已根据课题信息分析并撰写以下内容,帮您拓宽课题思路:
AI项目摘要
AI项目思路
AI技术路线图
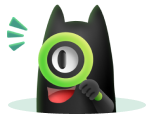
请为本次AI项目解读的内容对您的实用性打分
非常不实用
非常实用
1
2
3
4
5
6
7
8
9
10
您认为此功能如何分析更能满足您的需求,请填写您的反馈:
黄荣刚的其他基金
格陵兰冰盖表面裂隙的三维参数遥感提取方法与应用研究
- 批准号:42171443
- 批准年份:2021
- 资助金额:54 万元
- 项目类别:面上项目
相似国自然基金
{{ item.name }}
- 批准号:{{ item.ratify_no }}
- 批准年份:{{ item.approval_year }}
- 资助金额:{{ item.support_num }}
- 项目类别:{{ item.project_type }}
相似海外基金
{{
item.name }}
{{ item.translate_name }}
- 批准号:{{ item.ratify_no }}
- 财政年份:{{ item.approval_year }}
- 资助金额:{{ item.support_num }}
- 项目类别:{{ item.project_type }}