基于深度感知增量式联想记忆神经网络的信息融合系统研究
项目介绍
AI项目解读
基本信息
- 批准号:61876076
- 项目类别:面上项目
- 资助金额:62.0万
- 负责人:
- 依托单位:
- 学科分类:F0601.人工智能基础
- 结题年份:2022
- 批准年份:2018
- 项目状态:已结题
- 起止时间:2019-01-01 至2022-12-31
- 项目参与者:赵健; 赵金熙; 徐百乐; 张天玥; 刘小亮; 张旭; 黄羽佳; 高钟烨; 姚杨;
- 关键词:
项目摘要
Information fusion of multi-sensing channels is one of the basic functions of the human brain. The integration of many basic sensing channels such as vision and hearing through the brain's synesthetic area can increase the accuracy of perception. The information fusion technologies simulating this ability of human brain have a wide range of applications in many fields such as artificial intelligence, medical treatment and military weaponry. This project will simulate the human brain's information fusion mechanism and construct an information fusion intelligent system. In view of the shortcomings of current information fusion systems such as multi-channel deep perception, the fusion of abstractive patterns in heterogeneous channels, incremental learning of new targets, and incremental expansion of new perceptual channels, this project will study the multi-channel associative memory neural network and apply it to the information fusion system. The information fusion system designed by this project will be capable of acquiring and expressing multi-channel perceptive information, adapting to dynamic environments and incremental learning, associative memorizing multi-channel information, recognizing and understanding targets in environments. The research results of this project would promote the development of theories and technologies in the fields of brain-like computing and neural networks. This project is also important for understanding the human brain mechanism, the development of artificial intelligence and various types of AI applications.
多感知通道的信息融合是人类大脑的基本功能之一,视觉、听觉等多种基本感觉通道通过大脑联觉区域有机地整合起来,能够增加感知的精确性。基于人类这一能力发展起来的信息融合技术在人工智能、医疗、军事武器装备等多个领域均有着广泛的应用。本项目将模拟人类大脑的信息融合机制构建信息融合智能系统。针对目前的信息融合系统在多通道深层感知、异构通道抽象模式的融合、增量学习新型目标、增量式扩展新型感知通道等问题上存在的不足,本项目将研究多通道联想记忆神经网络并将其应用于信息融合系统,使信息融合系统具有多模态感知信息的获取与表示能力、动态实际环境下的系统适应性与增量学习能力、对多通道信息的联想记忆能力、对环境中目标成分的认知与理解能力。本项目的研究成果有利于促进在类脑计算与神经网络领域的理论与技术发展,对理解人类大脑机理、发展人工智能技术、推动各类智能应用有着非常重要的意义。
结项摘要
本项目模拟人类感知系统的神经结构特征,以联想记忆网络为基础构建一种能适应实际动态环境的增量式信息融合系统,并在该多模态数据的特征提取、特征交互、特征级与决策级信息融合等多个方面展开理论研究。课题组着重研究实际环境中海量数据的增量式特征学习、数据降维、聚类等基础理论和算法,并实现基于信息融合的自动驾驶、身份验证等应用系统来验证本项目的理论研究成果。在为期四年的项目研究期间,课题组研究人员依照项目计划书拟订的研究内容和计划,围绕深度感知神经网络、增量学习、特征交互算法、信息融合的应用等方面取得了创新性研究成果,完成了项目研究目标。研究成果共计发表论文12篇,在项目资助下,共计发表论文 11 篇,其中 9 篇被 SCI 收录,包括著名国际 刊 物<IEEE Trans. On Neural Networks and Learning System> 、<IEEE Trans. On Image Processing>、<Neural Networks>、<IEEE Trans. On Vehicular Technology>等,并申请国家发明专利30项,获得24项专利授权。
项目成果
期刊论文数量(11)
专著数量(0)
科研奖励数量(0)
会议论文数量(0)
专利数量(30)
Operation-aware Neural Networks for User Response Prediction
用于用户响应预测的操作感知神经网络
- DOI:10.1016/j.neunet.2019.09.020
- 发表时间:2019
- 期刊:Neural Networks
- 影响因子:7.8
- 作者:Yi Yang;Baile Xu;Furao Shen;Jian Zhao
- 通讯作者:Jian Zhao
Artificial Evolution Network: A Computational Perspective on the Expansibility of the Nervous System
人工进化网络:神经系统可扩展性的计算视角
- DOI:--
- 发表时间:2020
- 期刊:IEEE Transactions on Neural Networks and Learning Systems
- 影响因子:10.4
- 作者:You-Lu Xing;Hui Sun;Gui-Huan Feng;Fu-Rao Shen;Jian Zhao
- 通讯作者:Jian Zhao
V-SOINN: A topology preserving visualization method for multidimensional data
V-SOINN:多维数据的拓扑保留可视化方法
- DOI:10.1016/j.neucom.2021.03.113
- 发表时间:2021
- 期刊:Neurocomputing
- 影响因子:6
- 作者:Hui Dou;Baile Xu;Furao Shen;Jian Zhao
- 通讯作者:Jian Zhao
A density-based competitive data stream clustering network with self-adaptive distance metric
一种基于密度的自适应距离度量的竞争数据流聚类网络
- DOI:10.1016/j.neunet.2018.11.008
- 发表时间:2019
- 期刊:Neural Networks
- 影响因子:7.8
- 作者:Baile Xu;Furao Shen;Jian Zhao
- 通讯作者:Jian Zhao
一种自适应在线核密度估计方法
- DOI:10.13328/j.cnki.jos.005674
- 发表时间:2020
- 期刊:软件学报
- 影响因子:--
- 作者:邓齐林;邱天宇;申富饶;赵金熙
- 通讯作者:赵金熙
数据更新时间:{{ journalArticles.updateTime }}
{{
item.title }}
{{ item.translation_title }}
- DOI:{{ item.doi || "--"}}
- 发表时间:{{ item.publish_year || "--" }}
- 期刊:{{ item.journal_name }}
- 影响因子:{{ item.factor || "--"}}
- 作者:{{ item.authors }}
- 通讯作者:{{ item.author }}
数据更新时间:{{ journalArticles.updateTime }}
{{ item.title }}
- 作者:{{ item.authors }}
数据更新时间:{{ monograph.updateTime }}
{{ item.title }}
- 作者:{{ item.authors }}
数据更新时间:{{ sciAawards.updateTime }}
{{ item.title }}
- 作者:{{ item.authors }}
数据更新时间:{{ conferencePapers.updateTime }}
{{ item.title }}
- 作者:{{ item.authors }}
数据更新时间:{{ patent.updateTime }}
其他文献
自组织增量学习神经网络综述
- DOI:10.13328/j.cnki.jos.005068
- 发表时间:2016
- 期刊:软件学报
- 影响因子:--
- 作者:邱天宇;申富饶;赵金熙
- 通讯作者:赵金熙
自组织增量学习神经网络综述,软件学报,27(9):2230-2247
- DOI:--
- 发表时间:2016
- 期刊:软件学报
- 影响因子:--
- 作者:申富饶;邱天宇;赵金熙
- 通讯作者:赵金熙
其他文献
{{
item.title }}
{{ item.translation_title }}
- DOI:{{ item.doi || "--" }}
- 发表时间:{{ item.publish_year || "--"}}
- 期刊:{{ item.journal_name }}
- 影响因子:{{ item.factor || "--" }}
- 作者:{{ item.authors }}
- 通讯作者:{{ item.author }}
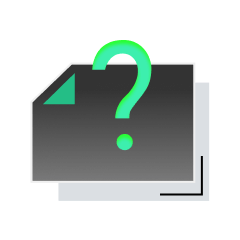
内容获取失败,请点击重试
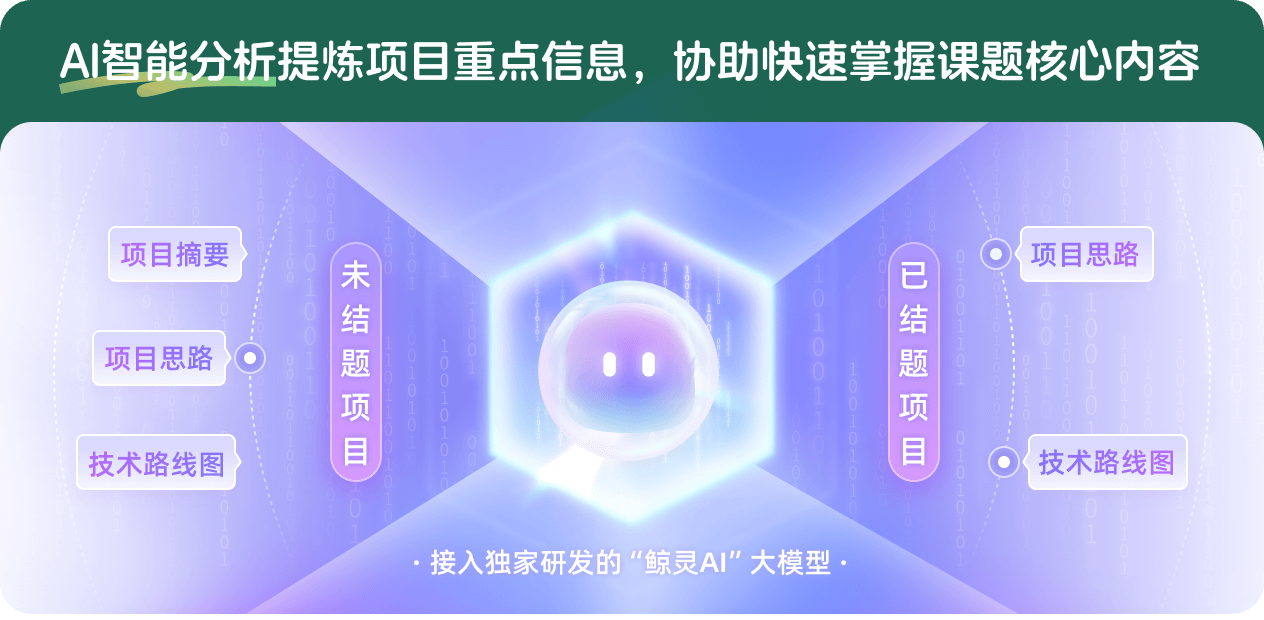
查看分析示例
此项目为已结题,我已根据课题信息分析并撰写以下内容,帮您拓宽课题思路:
AI项目摘要
AI项目思路
AI技术路线图
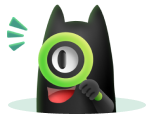
请为本次AI项目解读的内容对您的实用性打分
非常不实用
非常实用
1
2
3
4
5
6
7
8
9
10
您认为此功能如何分析更能满足您的需求,请填写您的反馈:
申富饶的其他基金
面向增量式无监督学习的新型神经网络研究
- 批准号:
- 批准年份:2022
- 资助金额:53 万元
- 项目类别:面上项目
实际环境中基于联想记忆网络的增量式推理系统研究
- 批准号:61375064
- 批准年份:2013
- 资助金额:67.0 万元
- 项目类别:面上项目
通用型记忆和联想增量学习神经网络研究
- 批准号:60975047
- 批准年份:2009
- 资助金额:29.0 万元
- 项目类别:面上项目
相似国自然基金
{{ item.name }}
- 批准号:{{ item.ratify_no }}
- 批准年份:{{ item.approval_year }}
- 资助金额:{{ item.support_num }}
- 项目类别:{{ item.project_type }}
相似海外基金
{{
item.name }}
{{ item.translate_name }}
- 批准号:{{ item.ratify_no }}
- 财政年份:{{ item.approval_year }}
- 资助金额:{{ item.support_num }}
- 项目类别:{{ item.project_type }}