复杂因素耦合的飞行器机构系统概率分析模型和计算方法研究
项目介绍
AI项目解读
基本信息
- 批准号:51105308
- 项目类别:青年科学基金项目
- 资助金额:25.0万
- 负责人:
- 依托单位:
- 学科分类:E0504.机械结构强度学
- 结题年份:2014
- 批准年份:2011
- 项目状态:已结题
- 起止时间:2012-01-01 至2014-12-31
- 项目参与者:崔卫民; 殷之平; 王慧; 张伟; 林晔; 雷鸣敏; 孙中超; 庞欢;
- 关键词:
项目摘要
本项目以机构系统为研究对象,针对其多因素耦合特性(机-液耦合,刚柔耦合等),以机构系统失效物理为核心,提出基于机构系统机-电-液混合仿真模型的概率分析模型和计算方法,具体内容包括:1)提出考虑多种因素耦合的机构系统仿真建模方法,实现机构系统确定性建模;2)考虑部件失效对机构系统特性的影响和机构系统动力特性变化对部件失效的影响,基于机构系统失效物理,提出多因素耦合的机构系统概率分析模型建模方法;3)提出发展高效的仿真抽样和机构系统仿真模型相结合的方法,应用计算机并行计算技术,解决机构系统的概率分析模型计算难题;4)通过某机构系统可靠性分析和相关试验数据,对所提出基于多因素耦合的仿真模型的机构系统概率分析理论方法进行验证。其成果旨在为飞行器复杂机构系统可靠性设计提供理论支持,对于提高我国飞行器安全性和可靠性具有重要意义。
结项摘要
本项目以机构系统为对象,针对机-液耦合、刚柔耦合等诸多因素耦合特性,以机构系统失效物理为核心,提出了基于机构系统机-电-液混合仿真模型的概率分析模型和计算方法。项目中提出考虑多种因素耦合的机构系统仿真建模方法,实现机构系统确定性建模;在考虑部件失效对机构系统特性的影响和机构系统动力特性变化对部件失效影响的基础上,基于机构系统失效物理,提出多因素耦合的机构系统概率分析模型建模方法;提出发展高效的仿真抽样和机构系统仿真模型相结合的方法,解决了大型分析模型的计算难题;通过某机构系统可靠性分析和相关试验数据,验证了所提出了理论方法。依托本项目的资助,发表论文10余篇,申请专利9项,获得授权2项,软件著作权2项,培养博士6名,硕士9名。本项目为飞行器复杂系统可靠性设计提供理论支持,对提高我国飞行器安全性和可靠性具有重要意义。
项目成果
期刊论文数量(20)
专著数量(0)
科研奖励数量(0)
会议论文数量(5)
专利数量(1)
低空高速飞行器整流罩分离技术研究现状和展望
- DOI:--
- 发表时间:2014
- 期刊:固体火箭技术
- 影响因子:--
- 作者:朱学昌;李浩远;喻天翔;宋笔锋
- 通讯作者:宋笔锋
Reliability Analysis for Close Position Accuracy of Gear Door Mechanism Based on Importance Sampling
基于重要性采样的档门机构关位精度可靠性分析
- DOI:--
- 发表时间:2012
- 期刊:Information-An International Interdisciplinary Journal
- 影响因子:--
- 作者:Zhongchao;Sun;Tianxiang;Yu;Weimin;Cui;Bifeng Song
- 通讯作者:Bifeng Song
平面连杆机构运动精度可靠性分析方法
- DOI:--
- 发表时间:--
- 期刊:中国机械工程
- 影响因子:--
- 作者:庞欢;喻天翔;王慧;宋笔锋
- 通讯作者:宋笔锋
运动机构可靠性仿真试验系统体系结构研究
- DOI:--
- 发表时间:2011
- 期刊:机械工程学报
- 影响因子:--
- 作者:王慧;喻天翔;雷鸣敏;宋笔锋
- 通讯作者:宋笔锋
Reliability Analysis of the Flap Mechanism with Multi-Pivots
多枢点襟翼机构的可靠性分析
- DOI:--
- 发表时间:2012
- 期刊:Information-An International Interdisciplinary Journal
- 影响因子:--
- 作者:Huan;Pang;Hui;Wang;Tianxiang;Yu;Bifeng Song
- 通讯作者:Bifeng Song
数据更新时间:{{ journalArticles.updateTime }}
{{
item.title }}
{{ item.translation_title }}
- DOI:{{ item.doi || "--"}}
- 发表时间:{{ item.publish_year || "--" }}
- 期刊:{{ item.journal_name }}
- 影响因子:{{ item.factor || "--"}}
- 作者:{{ item.authors }}
- 通讯作者:{{ item.author }}
数据更新时间:{{ journalArticles.updateTime }}
{{ item.title }}
- 作者:{{ item.authors }}
数据更新时间:{{ monograph.updateTime }}
{{ item.title }}
- 作者:{{ item.authors }}
数据更新时间:{{ sciAawards.updateTime }}
{{ item.title }}
- 作者:{{ item.authors }}
数据更新时间:{{ conferencePapers.updateTime }}
{{ item.title }}
- 作者:{{ item.authors }}
数据更新时间:{{ patent.updateTime }}
其他文献
飞机连杆机构多铰链磨损寿命综合预测方法
- DOI:10.7527/s1000-6893.2021.25113
- 发表时间:2022
- 期刊:航空学报
- 影响因子:--
- 作者:喻天翔;庄新臣;宋笔锋;孙中超
- 通讯作者:孙中超
考虑不同失效相关性的系统可靠性分配方法
- DOI:--
- 发表时间:2018
- 期刊:机械工程学报
- 影响因子:--
- 作者:张玉刚;孙杰;喻天翔
- 通讯作者:喻天翔
其他文献
{{
item.title }}
{{ item.translation_title }}
- DOI:{{ item.doi || "--" }}
- 发表时间:{{ item.publish_year || "--"}}
- 期刊:{{ item.journal_name }}
- 影响因子:{{ item.factor || "--" }}
- 作者:{{ item.authors }}
- 通讯作者:{{ item.author }}
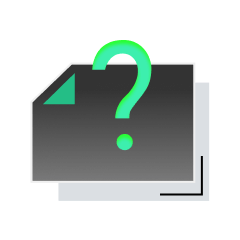
内容获取失败,请点击重试
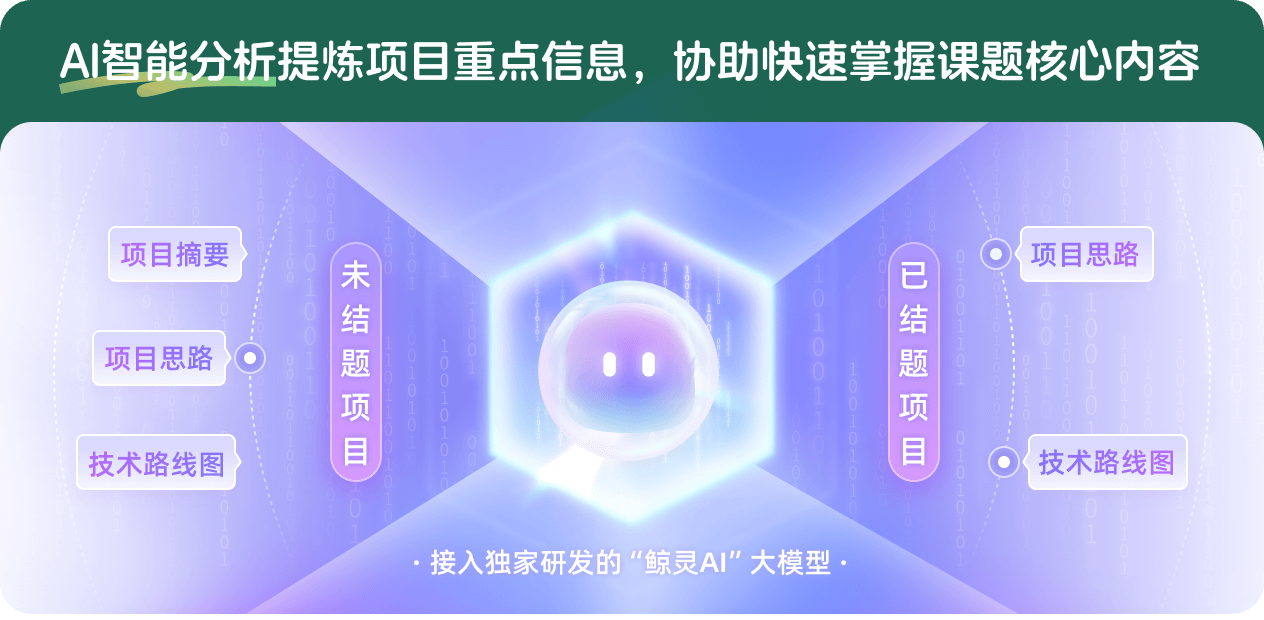
查看分析示例
此项目为已结题,我已根据课题信息分析并撰写以下内容,帮您拓宽课题思路:
AI项目摘要
AI项目思路
AI技术路线图
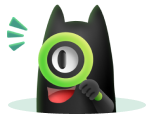
请为本次AI项目解读的内容对您的实用性打分
非常不实用
非常实用
1
2
3
4
5
6
7
8
9
10
您认为此功能如何分析更能满足您的需求,请填写您的反馈:
喻天翔的其他基金
多误差影响下花键载荷动态分配机制及可靠性演化模型研究
- 批准号:
- 批准年份:2020
- 资助金额:58 万元
- 项目类别:面上项目
隐身飞机操纵面封严失效机理及优化设计方法研究
- 批准号:51675428
- 批准年份:2016
- 资助金额:62.0 万元
- 项目类别:面上项目
相似国自然基金
{{ item.name }}
- 批准号:{{ item.ratify_no }}
- 批准年份:{{ item.approval_year }}
- 资助金额:{{ item.support_num }}
- 项目类别:{{ item.project_type }}
相似海外基金
{{
item.name }}
{{ item.translate_name }}
- 批准号:{{ item.ratify_no }}
- 财政年份:{{ item.approval_year }}
- 资助金额:{{ item.support_num }}
- 项目类别:{{ item.project_type }}