利用深度学习重构本地宇宙结构演化
项目介绍
AI项目解读
基本信息
- 批准号:11873051
- 项目类别:面上项目
- 资助金额:63.0万
- 负责人:
- 依托单位:
- 学科分类:A1402.宇宙结构的形成和演化
- 结题年份:2022
- 批准年份:2018
- 项目状态:已结题
- 起止时间:2019-01-01 至2022-12-31
- 项目参与者:毛天翔; 蒋桢;
- 关键词:
项目摘要
LCDM cosmological model is supported by a lot of observational evidences and almost becomes the standard cosmological model. However, we are still not very clear on some issues on understanding these observational data, e.g. cosmic variance; bias between observed galaxy distribution and dark matter background; the formation history of observed various structures. So it is very essential to reconstruct the evolution of the observed local universe to advance our understanding on these issues. This proposal will adopt the deep learning method, which is completely different from any traditional methods, to address this problem. With multilayer neural network (MNN), we will find out the mapping between the observed galaxy and total mass distribution, the mapping from the final obvserved mass distribution to their initial condition. With the proper reconstructed initial condition of the observed local universe( e.g. SDSS, 2MASS), we could reconstruct the evolution history of local universe by virtue of high resolution simulations. The MNN method is searching the link between the initial condition and final state directly, so it is much reliable to get the formation history of the structures in the observed universe. Also with more input information for MNN, it is not difficult to make the reconstruction better than any traditional methods at small scales given without overfit problems. The reconstructed mass distribution is very useful on understanding the total mass distribution in the observed universe, and the formation history of any structures inside.
LCDM宇宙学模型获得大量观测支持从而成为标准宇宙学模型。但为了更好的理解这些观测数据,比如大尺度观测上的宇宙方差(cosmic variance),星系分布与背景物质密度偏差(bias),以及理解观测到的本地宇宙中的各类结构的形成历史,我们都有必要重构观测到的宇宙的演化图景。本申请将采用和传统方法完全不一样的深度学习的方法来完成重构。首先利用多层神经网络的方法,寻找观测的星系分布(比如SDSS,2MASS)和背景总体物质(暗物质和重子物质)的联系;再通过深度学习找到从当前物质分布到原初物质分布的映射关系从而得到观测宇宙的原初条件;继而对这一原初条件进行高精度数值模拟重构本地宇宙的演化历史。深度学习是直接获得演化开始和结果的映射,故而可以更可靠的获得结构形成历史。同时可以通过增多对深度学习的信息输入,我们也可能可以获得比以前传统方法在更小尺度上的重构。重构出来的本地宇宙演化图景将有诸多应用
结项摘要
国内外新一代星系巡天都正在逐步开展,如果从星系巡天获得更多有用信息成为当前整个领域的重要挑战之一,本项目另辟蹊径,尝试利用新工具---人工智能技术从全新的途径完成该项目。本项目完成了所有项目规划,基本实现了科学目标:完成了大规模数值模拟Indra.全新有自主知识产权的星系形成与演化模型GABE(程序约2.5万行),从星系巡天到宇宙密度场重构的三个最重要关键模块的建模:非线性演化,红移畸变,偏袒因子。其中对宇宙密度场的重构的精度均与传统方法相当,或者更优。重要成果发表相关论文10余篇。基于目前的成果,我们将能利用已有的星系巡天或者新一代巡天的观测数据完成对宇宙物质密度场的重构,从而对宇宙学模型给出更精确的限制,并理解观测到的天体或者结构的形成演化历史。
项目成果
期刊论文数量(18)
专著数量(0)
科研奖励数量(0)
会议论文数量(0)
专利数量(0)
GABE: Galaxy Assembly with Binary Evolution
来自黑洞和吸积盘系统的幕式喷流的动力沙碰撞
- DOI:10.1088/1674
- 发表时间:2019
- 期刊:Research in Astronomy and Astrophysics
- 影响因子:1.8
- 作者:Jiang Zhen;Wang Jie;Gao Liang;Zhang Fenghui;Guo Qi;Wang Lan;Pan Jun
- 通讯作者:Pan Jun
Radio Frequency Interference Mitigation and Statistics in the Spectral Observations of FAST
FAST 频谱观测中的射频干扰缓解和统计
- DOI:10.1088/1674-4527/ac3f2d
- 发表时间:2021-11
- 期刊:Research in Astronomy and Astrophysics
- 影响因子:1.8
- 作者:Zhang Chuanpeng;Xu Jinlong;Wang Jie;Jing Yingjie;Liu Ziming;Zhu Ming;Jiang Peng
- 通讯作者:Jiang Peng
Universal structure of dark matter haloes over a mass range of 20 orders of magnitude
质量范围为 20 个数量级的暗物质晕的通用结构
- DOI:10.1038/s41586-020-2642-9
- 发表时间:2020-09-03
- 期刊:NATURE
- 影响因子:64.8
- 作者:Wang, J.;Bose, S.;White, S. D. M.
- 通讯作者:White, S. D. M.
Indra: a public computationally accessible suite of cosmological N-body simulations
Indra:公共计算可访问的宇宙 N 体模拟套件
- DOI:10.1093/mnras/stab1823
- 发表时间:2021-01
- 期刊:MONTHLY NOTICES OF THE ROYAL ASTRONOMICAL SOCIETY
- 影响因子:4.8
- 作者:Falck Bridget;Wang Jie;Jenkins Adrian;Lemson Gerard;Medvedev Dmitry;Neyrinck Mark C.;Szalay Alex S.
- 通讯作者:Szalay Alex S.
Simulating kilonovae in the ΛCDM universe
模拟ÎCDM 宇宙中的千新星
- DOI:10.1093/mnras/staa1989
- 发表时间:2020
- 期刊:MNRAS
- 影响因子:--
- 作者:Z. Jiang;J. Wang;张奉辉;L. Li;L. Wang;R. Li;L. Gao;韩占文;J. Pan
- 通讯作者:J. Pan
数据更新时间:{{ journalArticles.updateTime }}
{{
item.title }}
{{ item.translation_title }}
- DOI:{{ item.doi || "--"}}
- 发表时间:{{ item.publish_year || "--" }}
- 期刊:{{ item.journal_name }}
- 影响因子:{{ item.factor || "--"}}
- 作者:{{ item.authors }}
- 通讯作者:{{ item.author }}
数据更新时间:{{ journalArticles.updateTime }}
{{ item.title }}
- 作者:{{ item.authors }}
数据更新时间:{{ monograph.updateTime }}
{{ item.title }}
- 作者:{{ item.authors }}
数据更新时间:{{ sciAawards.updateTime }}
{{ item.title }}
- 作者:{{ item.authors }}
数据更新时间:{{ conferencePapers.updateTime }}
{{ item.title }}
- 作者:{{ item.authors }}
数据更新时间:{{ patent.updateTime }}
其他文献
基于BLSTM与方面注意力模块的情感分类方法
- DOI:10.19678/j.issn.1000-3428.0053929
- 发表时间:2020
- 期刊:计算机工程
- 影响因子:--
- 作者:彭祝亮;刘博文;范程岸;王杰;肖明;廖泽恩
- 通讯作者:廖泽恩
广义Hamilton多机电力系统的广域时滞阻尼控制
- DOI:10.13334/j.0258-8013.pcsee.2014.34.023
- 发表时间:2014
- 期刊:中国电机工程学报
- 影响因子:--
- 作者:古丽扎提·海拉提;王杰
- 通讯作者:王杰
干旱区梭梭茎干液流特性及对土壤水分的响应
- DOI:10.16232/j.cnki.1001-4179.2018.06.006
- 发表时间:2018
- 期刊:人民长江
- 影响因子:--
- 作者:杨明杰;杨广;何新林;陈东;王杰;李鹏飞
- 通讯作者:李鹏飞
湖北巴东地区滑脱构造与地震活动特征
- DOI:10.14075/j.jgg.2018.03.002
- 发表时间:2018
- 期刊:大地测量与地球动力学
- 影响因子:--
- 作者:王杰;王秋良;黄颂;吴海波;赵凌云;陈俊华
- 通讯作者:陈俊华
计及随机小扰动影响下的多机系统稳定性分析
- DOI:10.16081/j.issn.1006-6047.2017.02.025
- 发表时间:2017
- 期刊:电力自动化设备
- 影响因子:--
- 作者:王芮;王杰;弥潇
- 通讯作者:弥潇
其他文献
{{
item.title }}
{{ item.translation_title }}
- DOI:{{ item.doi || "--" }}
- 发表时间:{{ item.publish_year || "--"}}
- 期刊:{{ item.journal_name }}
- 影响因子:{{ item.factor || "--" }}
- 作者:{{ item.authors }}
- 通讯作者:{{ item.author }}
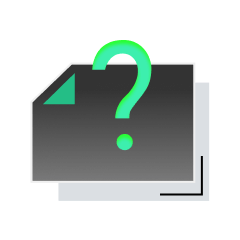
内容获取失败,请点击重试
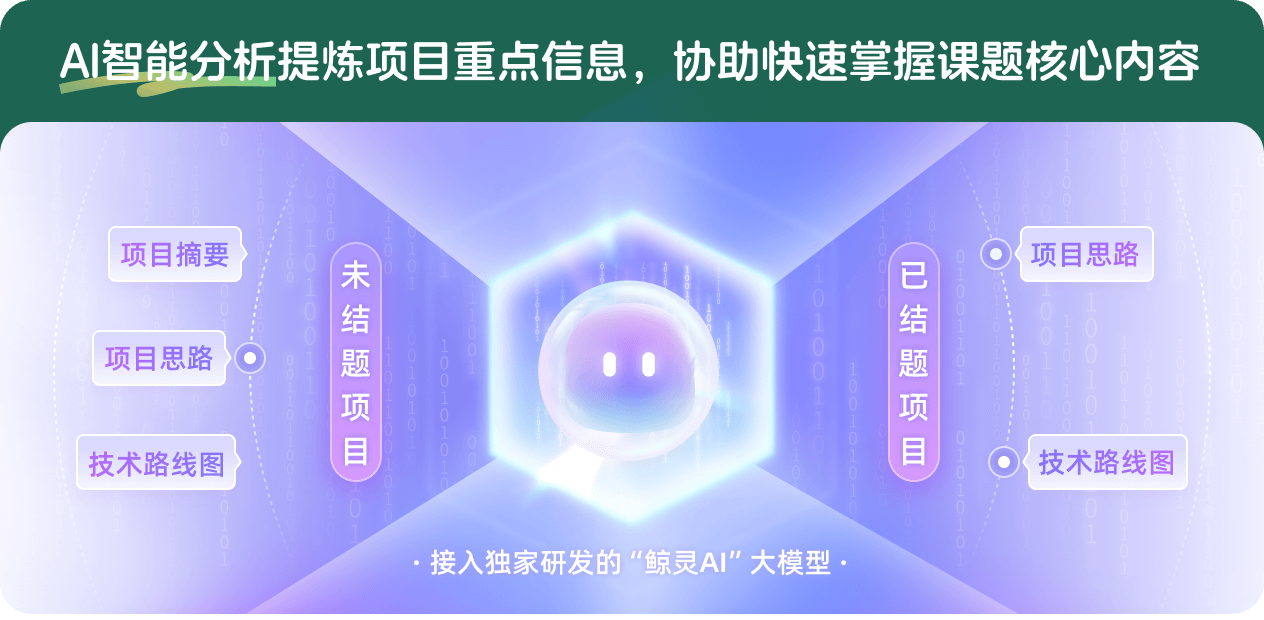
查看分析示例
此项目为已结题,我已根据课题信息分析并撰写以下内容,帮您拓宽课题思路:
AI项目摘要
AI项目思路
AI技术路线图
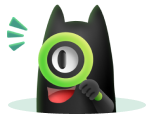
请为本次AI项目解读的内容对您的实用性打分
非常不实用
非常实用
1
2
3
4
5
6
7
8
9
10
您认为此功能如何分析更能满足您的需求,请填写您的反馈:
王杰的其他基金
模拟宇宙空洞中的结构形成
- 批准号:11373029
- 批准年份:2013
- 资助金额:86.0 万元
- 项目类别:面上项目
相似国自然基金
{{ item.name }}
- 批准号:{{ item.ratify_no }}
- 批准年份:{{ item.approval_year }}
- 资助金额:{{ item.support_num }}
- 项目类别:{{ item.project_type }}
相似海外基金
{{
item.name }}
{{ item.translate_name }}
- 批准号:{{ item.ratify_no }}
- 财政年份:{{ item.approval_year }}
- 资助金额:{{ item.support_num }}
- 项目类别:{{ item.project_type }}