α-强衰减稀疏信号重构理论研究与算法设计
项目介绍
AI项目解读
基本信息
- 批准号:11871248
- 项目类别:面上项目
- 资助金额:50.0万
- 负责人:
- 依托单位:
- 学科分类:A0602.信息技术与不确定性的数学理论与方法
- 结题年份:2022
- 批准年份:2018
- 项目状态:已结题
- 起止时间:2019-01-01 至2022-12-31
- 项目参与者:赵山程; 王立波; Zilong Liu; 李明; 刘炜; 刘家男; 杨文杰; 谢斐; 张佳婕;
- 关键词:
项目摘要
Compressed sensing is a signal recovery technique that has been proposed in the past 10 years, it is able to stably acquire sparse signals from far fewer samples than required by the Shannon-Nyquist sampling theorem. The α-strongly decaying sparse signals are a special class of sparse signals, which arise from many practical applications including speech communication and audio source separation. Compared with regular sparse signals, it is more difficult and challenging to investigate the recovery of this class of signals. Furthermore, there is less work on them. Based on the theories and techniques of compressed sensing, stochastic matrices, statistics, and algorithm design, etc., this project will design algorithms for acquiring α-strongly decaying and generalized α-strongly decaying sparse signals and study their recovery performance. More specifically, we will first study the properties of α-strongly decaying sparse signals, and then investigate the recovery performance of orthogonal matching pursuit for reconstructing α-strongly decaying sparse signals. Furthermore, we will study the design of recovery algorithms for the α-strongly decaying sparse signals with performance analysis. Finally, we will extend the design and analysis for the α-strongly decaying sparse signals to the generalized α-strongly decaying sparse signals. This project is of essential importance from both theoretical and practical points of view.
压缩感知是最近十余年提出的一种信号重构的技术,它能够在远小于奈奎斯特-香农采样率的条件下可靠地重构稀疏信号。α-强衰减稀疏信号是一类特殊的稀疏信号,它来源于广泛的实际应用,如语音通信、音频源分离等。相比于传统的稀疏信号,α-强衰减稀疏信号的研究难度更大,更具挑战性,已有研究结果更少。本项目拟基于压缩感知、随机矩阵、统计、算法设计等理论和技术,深入研究α-强衰减和广义α-强衰减稀疏信号重构的理论分析与算法设计:1)研究α-强衰减稀疏信号的性质;2)系统分析正交匹配追踪算法对α-强衰减稀疏信号重构的效果;3)α-强衰减稀疏信号快速高效重构算法的设计与性能分析;4)广义α-强衰减稀疏信号快速高效重构算法的设计与性能分析。这些课题的研究具有重要的理论意义和应用价值。
结项摘要
α-强衰减稀疏信号是一类特殊的稀疏信号,它广泛来源于语音通信、音频源分离等领域。相比于传统的稀疏信号,α-强衰减稀疏信号的研究难度更大,更具挑战性,已有研究结果更少。本项目基于压缩感知、随机矩阵、统计、算法设计等理论和技术,深入研究α-强衰减和广义α-强衰减稀疏信号重构的理论分析与算法设计:1)建立了α-强衰减稀疏信号的性质,为稀疏重构算法还原这类信号的性能分析提供帮助; 2)系统分析正交匹配追踪算法对α-强衰减稀疏信号重构的效果,改进了国际数学家大会特邀报告人Gilbert等著名学者的正交匹配追踪算法的重构效果分析,为实际应用中是否采用OMP重构这类信号提供理论依据。理论解释了正交匹配追踪算法重构α-强衰减稀疏信号比重构平稀疏信号、高斯稀疏信号的效果更好;3)设计了广义α-强衰减稀疏信号——二值稀疏信号重构的快速算法二值匹配追踪算法 (BMP) 并分析了其重构性能;设计了广义空间调制活跃用户的快速检测算法并分析了其重构性能;4)首次建立了基于互相干性的通过求解最小化1-范数和2-范数差的优化问题稳健重构稀疏信号的充分条件, 为实际应用中是否可以通过解最小化1-范数和2-范数的差的优化问题重构稀疏信号提供了理论依据。本项目的研究成果可用于医疗成像、信号处理、物联网等领域。
项目成果
期刊论文数量(37)
专著数量(1)
科研奖励数量(0)
会议论文数量(2)
专利数量(10)
On Low-Complexity Lattice Reduction Algorithms for Large-Scale MIMO Detection: The Blessing of Sequential Reduction
用于大规模 MIMO 检测的低复杂度格约化算法:顺序约简的祝福
- DOI:10.1109/tsp.2019.2959194
- 发表时间:2020
- 期刊:IEEE Transactions on Signal Processing
- 影响因子:5.4
- 作者:Lyu Shanxiang;Wen Jinming;Weng Jian;Ling Cong
- 通讯作者:Ling Cong
On Maximizing Min Source Rate in Power Beacon Assisted IoTs Networks
功率信标辅助物联网网络中最小源速率最大化
- DOI:10.1109/tvt.2020.3006232
- 发表时间:2020
- 期刊:IEEE Transactions on Vehicular Technology
- 影响因子:6.8
- 作者:Tengjiao He;Kwan-Wu Chin;Sieteng Soh;Changlin Yang;Jinming Wen
- 通讯作者:Jinming Wen
Spatially Coupled Codes via Partial and Recursive Superposition for Industrial IoT With High Trustworthiness
通过部分递归叠加实现高可信度工业物联网的空间耦合代码
- DOI:10.1109/tii.2020.2965952
- 发表时间:2020
- 期刊:IEEE Transactions on Industrial Informatics
- 影响因子:12.3
- 作者:Zhao Shancheng;Wen Jinming;Mumtaz Shahid;Garg Sahil;Choi Bong Jun
- 通讯作者:Choi Bong Jun
On the KZ Reduction
关于 KZ 还原
- DOI:10.1109/tit.2018.2868945
- 发表时间:2019
- 期刊:IEEE Transactions on Information Theory
- 影响因子:2.5
- 作者:Jinming Wen;Xiao-Wen Chang
- 通讯作者:Xiao-Wen Chang
New Security Mechanisms of High-Reliability IoT Communication Based on Radio Frequency Fingerprint
基于射频指纹的高可靠物联网通信安全新机制
- DOI:10.1109/jiot.2019.2913627
- 发表时间:2019
- 期刊:IEEE Internet of Things Journal
- 影响因子:10.6
- 作者:Tian Qiao;Lin Yun;Guo Xinghao;Wen Jinming;Fang Yi;Rodriguez Jonathan;Mumtaz Shahid
- 通讯作者:Mumtaz Shahid
数据更新时间:{{ journalArticles.updateTime }}
{{
item.title }}
{{ item.translation_title }}
- DOI:{{ item.doi || "--"}}
- 发表时间:{{ item.publish_year || "--" }}
- 期刊:{{ item.journal_name }}
- 影响因子:{{ item.factor || "--"}}
- 作者:{{ item.authors }}
- 通讯作者:{{ item.author }}
数据更新时间:{{ journalArticles.updateTime }}
{{ item.title }}
- 作者:{{ item.authors }}
数据更新时间:{{ monograph.updateTime }}
{{ item.title }}
- 作者:{{ item.authors }}
数据更新时间:{{ sciAawards.updateTime }}
{{ item.title }}
- 作者:{{ item.authors }}
数据更新时间:{{ conferencePapers.updateTime }}
{{ item.title }}
- 作者:{{ item.authors }}
数据更新时间:{{ patent.updateTime }}
其他文献
Nearly Optimal Number of Iterations for Sparse Signal Recovery with Orthogonal Multi-Matching Pursuit
正交多重匹配追踪稀疏信号恢复的近乎最佳迭代次数
- DOI:--
- 发表时间:2021
- 期刊:Inverse Problems
- 影响因子:2.1
- 作者:李海锋;温金明;冼军;张静
- 通讯作者:张静
噪声干扰下 m2OLS 算法的稀疏重构分析
- DOI:--
- 发表时间:2021
- 期刊:中国科学: 数学
- 影响因子:--
- 作者:李海锋;谌稳固;温金明
- 通讯作者:温金明
噪声干扰下m~2OLS算法的稀疏重构分析
- DOI:--
- 发表时间:2021
- 期刊:中国科学:数学
- 影响因子:--
- 作者:李海锋;谌稳固;温金明
- 通讯作者:温金明
基于序贯贝叶斯参数学习的Lévy动态波动率模型研究
- DOI:--
- 发表时间:2017
- 期刊:系统工程理论与实践
- 影响因子:--
- 作者:吴恒煜;朱福敏;温金明;AaronKIM
- 通讯作者:AaronKIM
Required Number of Iterations for Sparse Signal Recovery via Orthogonal Least Squares
通过正交最小二乘法恢复稀疏信号所需的迭代次数
- DOI:10.4208/jcm.2104-m2020-0093
- 发表时间:2023
- 期刊:Journal of Computational Mathematics
- 影响因子:0.9
- 作者:李海锋;张静;温金明;李东方
- 通讯作者:李东方
其他文献
{{
item.title }}
{{ item.translation_title }}
- DOI:{{ item.doi || "--" }}
- 发表时间:{{ item.publish_year || "--"}}
- 期刊:{{ item.journal_name }}
- 影响因子:{{ item.factor || "--" }}
- 作者:{{ item.authors }}
- 通讯作者:{{ item.author }}
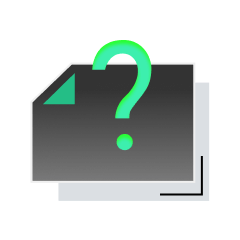
内容获取失败,请点击重试
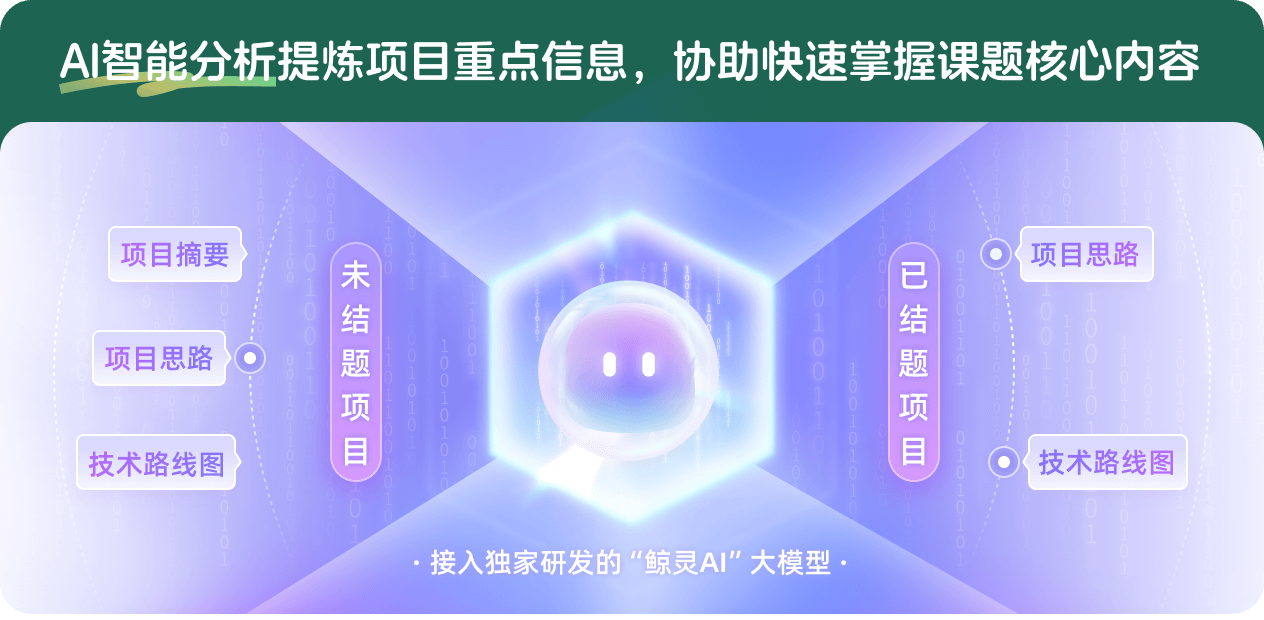
查看分析示例
此项目为已结题,我已根据课题信息分析并撰写以下内容,帮您拓宽课题思路:
AI项目摘要
AI项目思路
AI技术路线图
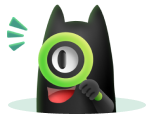
请为本次AI项目解读的内容对您的实用性打分
非常不实用
非常实用
1
2
3
4
5
6
7
8
9
10
您认为此功能如何分析更能满足您的需求,请填写您的反馈:
温金明的其他基金
稀疏信号重构的随机算法设计与理论分析
- 批准号:12271215
- 批准年份:2022
- 资助金额:46 万元
- 项目类别:面上项目
相似国自然基金
{{ item.name }}
- 批准号:{{ item.ratify_no }}
- 批准年份:{{ item.approval_year }}
- 资助金额:{{ item.support_num }}
- 项目类别:{{ item.project_type }}
相似海外基金
{{
item.name }}
{{ item.translate_name }}
- 批准号:{{ item.ratify_no }}
- 财政年份:{{ item.approval_year }}
- 资助金额:{{ item.support_num }}
- 项目类别:{{ item.project_type }}