3D形变体的保测变换与稀疏流形嵌入识别方法研究
项目介绍
AI项目解读
基本信息
- 批准号:61671397
- 项目类别:面上项目
- 资助金额:50.0万
- 负责人:
- 依托单位:
- 学科分类:F0116.图像信息处理
- 结题年份:2020
- 批准年份:2016
- 项目状态:已结题
- 起止时间:2017-01-01 至2020-12-31
- 项目参与者:王其聪; 郑炜; 谢怡; 赵冲; 张艳; 陈蓉; 余胜; 赵鑫; 曾晓嘉;
- 关键词:
项目摘要
The feature detection and recognition of 3D objects are among the frontier research topics and the techniques have many applications in the fields like vision cognition, autonomous robot, intelligent surveillance, medical image processing, etc. To tackle the unsolved yet important problems of recognizing the non-rigid 3D objects which encounter deformation, we propose to: 1. Develop the new theory and algorithms to present the features of dynamic deformation, where the deformation may lead to great changes of appearance and the features of the object are rich and correlative. The new ways of measure preserving transformation, especially the preserving of volume and surface area, are to be established to model the dynamic shape changes of non-rigid 3D objects; and by using the symplectic geometry difference scheme in the scope of homeomorphic mapping of differential manifold to keep the solutions of dynamic deformation be stable with high accuracy. 2. Study the data-driven sparse representation embedded graphs, and the discriminative sparse regularization based manifold regression techniques, thus combine the sparsity, the discriminative knowledge of data and the local geometric structure into manifold regression, so to obtain a more discriminative solution for the recognition of 3D deformable objects that cannot be gotten via only manifold regularization. Our research results will form a new and systematic theory and the algorithms of feature representation and recognition for non-rigid deformable 3D objects.
表达三维(3D)形变物体的特征并识别3D物体是一个前沿研究热点,在视觉认知、自主机器人、智能监控、医学图像处理等领域有着广泛的应用价值。本项目针对非刚性3D物体形变前后的特征表达及识别这一待解决的重要问题展开研究。1.发展新的形变表征方法:形变引起的3D动态变化范围很大、表观特征众多且互相关联,故提出保测度尤其是保面积、保体积的动态形变模型的新思路,解决3D物体自主变化从而相当于动态形变的问题。提出动态形变时考虑微分流形同胚影射下的辛几何差分格式计算思路,以使形变的求解过程稳定并获得较高计算精度。2. 研究基于数据驱动的稀疏表示构图,以及基于判别的稀疏正则化流形回归技术,将稀疏性、数据的判别知识和局部几何结构融入到流形回归,能够给出流形正则化上不能获得的具有更强判别力的稀疏解,从而解决3D形变体的识别问题。研究成果可对形变3D物体的特征表达及识别问题贡献创新的理论方法和算法。
结项摘要
表达三维(3D)形变物体的特征并识别3D物体是一个前沿研究热点,在视觉认知、自主机器人、智能监控、医学图像处理等领域有着广泛的应用价值。本项目针对非刚性3D物体形变前后的特征表达及识别这一重要问题展开了研究。a).提出了一种针对3D点云对象的端到端无监督推理、空间关键点深度学习的SK-Net,对点云特征学习是一个具有通用性的框架算法。针对3D人体姿态估计中传统基于样例方法的局限性,提出了一种基于相似局部检索的3D人体姿态估计算法。提出了一种重新加权L1正则化策略,给出了一种基于残差补偿的重加权稀疏表达在单张RGB图像下的人体3D姿态估计方法。提出了一种基于稀疏表达模型的无监督学习的多几何先验3D人体姿态估计方法SDM3d,能够在有限的训练数据下较好地估计各种复杂3D姿态。以上的结果具有可扩展至多类3D物体的变形、查找和识别等。b).提出了一种用于视频动作识别的时空卷积神经网络框架,采用稀疏自动组合策略对多输入特征图进行组合,提高其特征学习能力和分类能力。提出了面向大规模图像分类的本体驱动层次的一种稀疏编码方法,构建了两层本体来按层次进行大规模的图像分类。提出了基于当前统计模型的模糊交互多模型H∞粒子滤波算法,用于机动目标追踪。提出了用于异常检测的基于粒子群优化广义帕累托模型。c).给出了带有隐式反馈的 SVD 推荐模型高效求解算法,设计了BCDSVD++并实现模型的高效求解。基于语义图嵌入及跨模态注意机制来学习语义标签的嵌入,给出了一种多标签分类方法。d).因涉及到的视频与3D物体数据量巨大,研究了大数据工作流调度的几个重要优化问题。提出了云环境下多目标工作流调度的分解方法;提出了一个用于在任务执行和数据传输中存在时间不可预测性的DAG应用的增强的基于优先级的调度启发式算法;给出了一个用于大数据处理系统中多个受时间限制的工作流的在线调度的基准测试方法及其工具包。
项目成果
期刊论文数量(15)
专著数量(0)
科研奖励数量(0)
会议论文数量(10)
专利数量(3)
An enhanced priority-based scheduling heuristic for DAG applications with temporal unpredictability in task execution and data transmission
一种增强的基于优先级的调度启发式,适用于任务执行和数据传输中具有时间不可预测性的 DAG 应用程序
- DOI:10.1016/j.future.2019.04.030
- 发表时间:2019
- 期刊:Future Generation Computer Systems-The International Journal of eScience
- 影响因子:7.5
- 作者:Zhang Xinbo;Zhang Dongzhan;Zheng Wei;Chen Jinjun
- 通讯作者:Chen Jinjun
SDM3d: shape decomposition of multiple geometric priors for 3D pose estimation
SDM3d:用于 3D 姿态估计的多个几何先验的形状分解
- DOI:10.1007/s00521-020-05086-0
- 发表时间:2020-06
- 期刊:Neural Computing and Applications
- 影响因子:6
- 作者:Mengxi Jiang;Zhuliang Yu;Cuihua Li;Yunqi Lei
- 通讯作者:Yunqi Lei
How to achieve auto-identification in Raman analysis by spectral feature extraction & Adaptive Hypergraph
如何通过光谱特征提取实现拉曼分析中的自动识别
- DOI:10.1016/j.saa.2019.04.078
- 发表时间:2019
- 期刊:Spectrochimica Acta Part A: Molecular and Biomolecular Spectroscopy
- 影响因子:--
- 作者:Yi Xie;Qiaobei You;Pingyang Dai;Shuyi Wang;Peiyi Hong;Guokun Liu;Jun Yu;Xilong Sun;Yongming Zeng
- 通讯作者:Yongming Zeng
Fuzzy Interacting Multiple Model H∞ Particle Filter Algorithm Based on Current Statistical Model
基于现行统计模型的模糊交互多模型H·粒子滤波算法
- DOI:10.1007/s40815-019-00678-y
- 发表时间:2019
- 期刊:INTERNATIONAL JOURNAL OF FUZZY SYSTEMS
- 影响因子:4.3
- 作者:Qicong Wang;Xiaoqiang Chen;Lin Zhang;Jin Li;Chong Zhao;Man Qi
- 通讯作者:Man Qi
Generalized Pareto Model Based on Particle Swarm Optimization for Anomaly Detection
基于粒子群优化的广义帕累托模型异常检测
- DOI:10.1109/access.2019.2957806
- 发表时间:2019
- 期刊:IEEE ACCESS
- 影响因子:3.9
- 作者:YAN HUANG;FUYU DU;JIAN CHEN;YAN CHEN;QICONG WANG;MAOZHEN L
- 通讯作者:MAOZHEN L
数据更新时间:{{ journalArticles.updateTime }}
{{
item.title }}
{{ item.translation_title }}
- DOI:{{ item.doi || "--"}}
- 发表时间:{{ item.publish_year || "--" }}
- 期刊:{{ item.journal_name }}
- 影响因子:{{ item.factor || "--"}}
- 作者:{{ item.authors }}
- 通讯作者:{{ item.author }}
数据更新时间:{{ journalArticles.updateTime }}
{{ item.title }}
- 作者:{{ item.authors }}
数据更新时间:{{ monograph.updateTime }}
{{ item.title }}
- 作者:{{ item.authors }}
数据更新时间:{{ sciAawards.updateTime }}
{{ item.title }}
- 作者:{{ item.authors }}
数据更新时间:{{ conferencePapers.updateTime }}
{{ item.title }}
- 作者:{{ item.authors }}
数据更新时间:{{ patent.updateTime }}
其他文献
其他文献
{{
item.title }}
{{ item.translation_title }}
- DOI:{{ item.doi || "--" }}
- 发表时间:{{ item.publish_year || "--"}}
- 期刊:{{ item.journal_name }}
- 影响因子:{{ item.factor || "--" }}
- 作者:{{ item.authors }}
- 通讯作者:{{ item.author }}
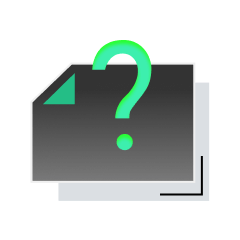
内容获取失败,请点击重试
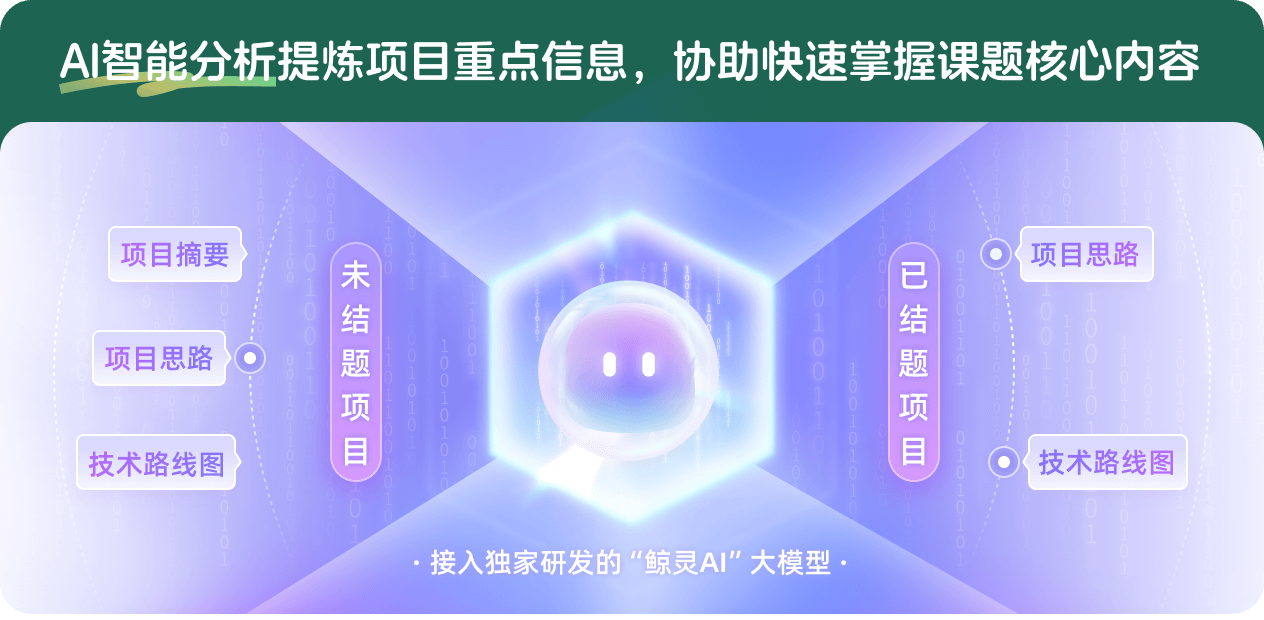
查看分析示例
此项目为已结题,我已根据课题信息分析并撰写以下内容,帮您拓宽课题思路:
AI项目摘要
AI项目思路
AI技术路线图
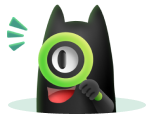
请为本次AI项目解读的内容对您的实用性打分
非常不实用
非常实用
1
2
3
4
5
6
7
8
9
10
您认为此功能如何分析更能满足您的需求,请填写您的反馈:
相似国自然基金
{{ item.name }}
- 批准号:{{ item.ratify_no }}
- 批准年份:{{ item.approval_year }}
- 资助金额:{{ item.support_num }}
- 项目类别:{{ item.project_type }}
相似海外基金
{{
item.name }}
{{ item.translate_name }}
- 批准号:{{ item.ratify_no }}
- 财政年份:{{ item.approval_year }}
- 资助金额:{{ item.support_num }}
- 项目类别:{{ item.project_type }}