基于时空分类数据的过程质量控制方法研究
项目介绍
AI项目解读
基本信息
- 批准号:71672122
- 项目类别:面上项目
- 资助金额:48.0万
- 负责人:
- 依托单位:
- 学科分类:G0108.工业工程与质量管理
- 结题年份:2020
- 批准年份:2016
- 项目状态:已结题
- 起止时间:2017-01-01 至2020-12-31
- 项目参与者:张阳; 曲亮; 王志琼; 周盼盼; 杨晓曦; 李振;
- 关键词:
项目摘要
Spatiotemporal categorical data are very common in manufacturing and service industries, such as data from wafer quality checking and public health. It is very important to detect the change in products’ quality and events’ probability. However, most conventional statistical process control techniques cannot effectively monitor such attribute data on a real-time basis when spatial and temporal correlation exists. The central idea of the proposed research is to develop methodologies to achieve efficient statistical process control for such spatiotemporal categorical data. This involves the following research contents: (1) developing a hierarchical model to model spatial binomial data, (2) developing control charts to monitor spatial binomial data based on the general likelihood ratio statistic and the proposed model, (3) developing a BVB-AR(1)model to model bivariate autocorrelated binomial data, (4) developing control statistic by integrating EWMA and likelihood function, and calculating ARL by Markov chain methods, moreover, proposing the diagnosing methods by integrating change-point methods together to detect the change points, (5) the effect of model parameter estimation will be studied. The research will expand statistical process control techniques for attribute data from different aspects, and lead to the comprehensive surveillance of products and service quality.
时空分类数据在实际制造及服务业中是很常见的,例如晶片检验数据及公共卫生数据。对产品质量及事件发生进行异常识别是极其重要的问题。然而传统的统计过程控制方法不能有效地对时空分类数据进行监控。因此,本项目的目标是要提出有效的适用于时空分类数据的统计过程控制方法。研究内容将集中在如下几方面:(1)利用层次模型对空间二项数据进行建模,(2)基于模型及数据特点,构建似然比控制图,对其进行监控,(3)利用BVB-AR(1)模型对二元自相关二项分类数据进行建模,(4)基于模型,结合指数加权移动平均(EWMA)与似然函数构建控制图,利用马尔科夫链计算控制图指标(平均运行长度),并利用变点理论对异常发生位置进行诊断,(5)模型参数估计对控制图的影响。项目研究成果对于拓展离散变量统计过程控制的研究方法,推动其在实际中的应用具有重要理论和应用价值。
结项摘要
在自然科学基金青年基金(#71672122)的资助下,课题组针对空间分类数据,自相关分类数据以及轮廓等数据的统计过程控制方法展开研究,完成了如下几个方面的工作:(1)以晶圆缺陷数据为例,考虑了二维空间数据的空间分布和空间结构对空间信息的影响,采用高斯马尔科夫随机场和分层贝叶斯模型对二维空间离散数据建模。然后,基于多元的指数加权移动平均控制图,提出了对二维空间离散数据总体趋势和空间相关性的变化的监测方案;(2)针对实际过程中的自相关分类数据,采用广义估计方程方法对轮廓内相关性进行建模,提出了第一阶段变点诊断方法,然后针对第二阶段监控问题,提出了基于广义似然比的休哈特控制图以及累积和控制图,并通过汽车质保数据进行验证;(3)结合轮廓数据中的输出变量及解释变量之间的函数关系,提出了基于线性及非线性轮廓以及离散轮廓数据的控制图以及变点和异常值诊断方法,改进了现有方法在监控轮廓数据时存在的局限性,并通过实际收集到的数据对所提出的方法进行验证;(4)针对不同类型的离散数据,包括泊松数据,社交网络属性数据以及属性事件发生率或间隔时间等,提出了非参控制图,累积和以及指数加权移动平均控制图等,通过仿真分析,研究了控制图的性能,进行了理论和应用研究。 ..到目前为止,课题组共发表(含录用)论文17篇,其中为SCI检索论文15篇,中文A类1篇。培养博士研究生3名,硕士研究生4名,参加国际学术会议10余次。此外,在项目资助期间,2名项目参与人晋升副教授。在已有成果的基础上,课题组拟在时空分类数据以及高频高维的分类数据(包括轮廓)过程监控与诊断方面进行进一步深入研究。
项目成果
期刊论文数量(16)
专著数量(0)
科研奖励数量(0)
会议论文数量(0)
专利数量(0)
晶圆缺陷数据的建模与仿真
- DOI:10.16468/j.cnki.issn1004-0366.2018.06.026
- 发表时间:2018
- 期刊:甘肃科学学报
- 影响因子:--
- 作者:李涛;王志琼
- 通讯作者:王志琼
Performance evaluation method for network monitoring based on separable temporal exponential random graph models with application to the study of autocorrelation effects
基于可分离时间指数随机图模型的网络监测性能评估方法及其自相关效应研究
- DOI:10.1016/j.cie.2020.106507
- 发表时间:2020
- 期刊:Computers & Industrial Engineering
- 影响因子:7.9
- 作者:Zhou Panpan;Lin Dennis K. J.;Niu Xiaoyue;He Zhen
- 通讯作者:He Zhen
Change point detection in Phase I for autocorrelated Poisson profiles with random or unbalanced designs
具有随机或不平衡设计的自相关泊松分布的第一阶段变化点检测
- DOI:10.1080/00207543.2020.1762017
- 发表时间:2020
- 期刊:International Journal of Production Research
- 影响因子:9.2
- 作者:Shuguang He;Lisha Song;Yanfen Shang;Zhiqiong Wang
- 通讯作者:Zhiqiong Wang
Phase I non-linear profiles monitoring using a modified Hausdorff distance algorithm and clustering analysis
使用改进的 Hausdorff 距离算法和聚类分析进行第一阶段非线性剖面监测
- DOI:10.1108/ijqrm-01-2020-0001
- 发表时间:2020-07
- 期刊:International Journal of Quality & Reliability Management
- 影响因子:2.5
- 作者:Bin Nie;Diqing Liu;Xiaohui Liu;Wenjing Ye
- 通讯作者:Wenjing Ye
Exponential cumulative sums chart for detecting shifts in time-between-events
用于检测事件之间时间变化的指数累积和图
- DOI:10.1080/00207543.2017.1412523
- 发表时间:2018-05
- 期刊:International Journal of Production Research
- 影响因子:9.2
- 作者:Qu Liang;Khoo Michael B. C.;Castagliola Philippe;Zhen He
- 通讯作者:Zhen He
数据更新时间:{{ journalArticles.updateTime }}
{{
item.title }}
{{ item.translation_title }}
- DOI:{{ item.doi || "--"}}
- 发表时间:{{ item.publish_year || "--" }}
- 期刊:{{ item.journal_name }}
- 影响因子:{{ item.factor || "--"}}
- 作者:{{ item.authors }}
- 通讯作者:{{ item.author }}
数据更新时间:{{ journalArticles.updateTime }}
{{ item.title }}
- 作者:{{ item.authors }}
数据更新时间:{{ monograph.updateTime }}
{{ item.title }}
- 作者:{{ item.authors }}
数据更新时间:{{ sciAawards.updateTime }}
{{ item.title }}
- 作者:{{ item.authors }}
数据更新时间:{{ conferencePapers.updateTime }}
{{ item.title }}
- 作者:{{ item.authors }}
数据更新时间:{{ patent.updateTime }}
其他文献
Profile Monitoring with Binary Data and Random Predictors
使用二进制数据和随机预测器进行轮廓监测
- DOI:10.1080/00224065.2011.11917857
- 发表时间:2011-07
- 期刊:Journal of Quality Technology
- 影响因子:2.5
- 作者:商艳芬;宗福季;邹长亮
- 通讯作者:邹长亮
考虑手术分类协变量的风险 调整 EWMA 控制图
- DOI:--
- 发表时间:--
- 期刊:工业工程与管理
- 影响因子:--
- 作者:李龙辉;商艳芬;刘彦利;刘子先
- 通讯作者:刘子先
其他文献
{{
item.title }}
{{ item.translation_title }}
- DOI:{{ item.doi || "--" }}
- 发表时间:{{ item.publish_year || "--"}}
- 期刊:{{ item.journal_name }}
- 影响因子:{{ item.factor || "--" }}
- 作者:{{ item.authors }}
- 通讯作者:{{ item.author }}
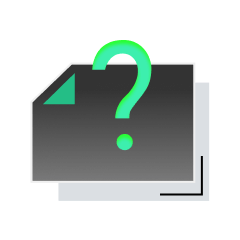
内容获取失败,请点击重试
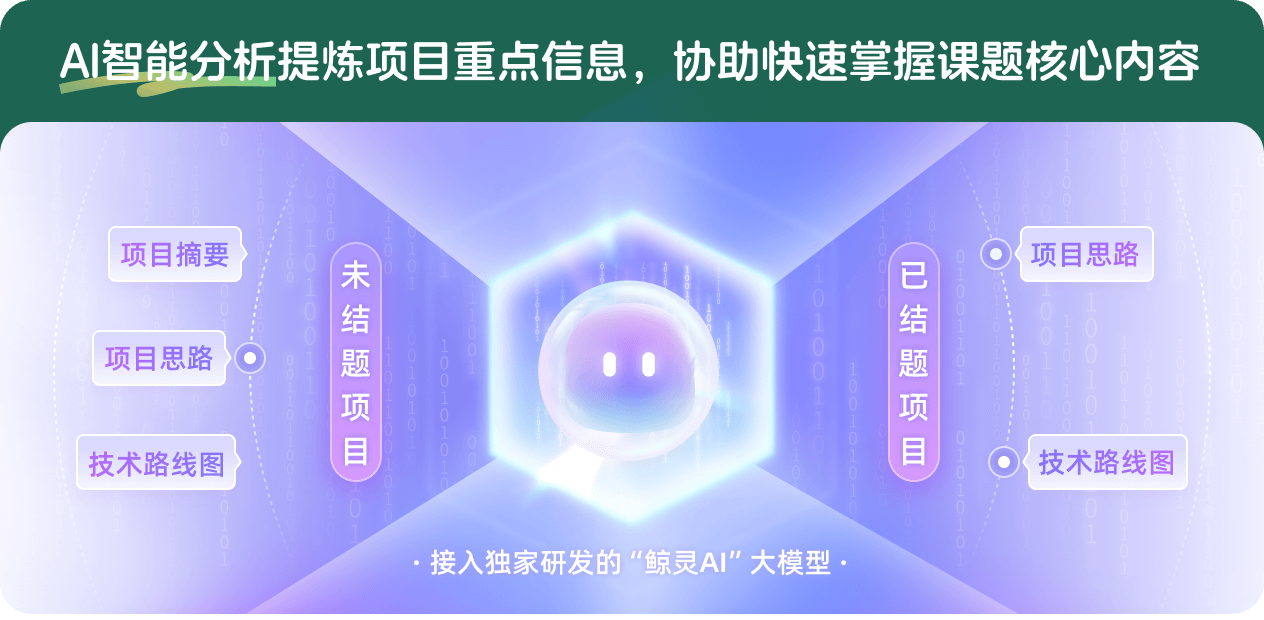
查看分析示例
此项目为已结题,我已根据课题信息分析并撰写以下内容,帮您拓宽课题思路:
AI项目摘要
AI项目思路
AI技术路线图
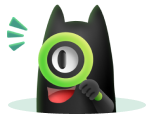
请为本次AI项目解读的内容对您的实用性打分
非常不实用
非常实用
1
2
3
4
5
6
7
8
9
10
您认为此功能如何分析更能满足您的需求,请填写您的反馈:
商艳芬的其他基金
服务过程中分类数据的监控及诊断方法的研究
- 批准号:71202087
- 批准年份:2012
- 资助金额:18.5 万元
- 项目类别:青年科学基金项目
相似国自然基金
{{ item.name }}
- 批准号:{{ item.ratify_no }}
- 批准年份:{{ item.approval_year }}
- 资助金额:{{ item.support_num }}
- 项目类别:{{ item.project_type }}
相似海外基金
{{
item.name }}
{{ item.translate_name }}
- 批准号:{{ item.ratify_no }}
- 财政年份:{{ item.approval_year }}
- 资助金额:{{ item.support_num }}
- 项目类别:{{ item.project_type }}