基于特征学习的空间非合作目标单目视觉位姿测量研究
项目介绍
AI项目解读
基本信息
- 批准号:61501429
- 项目类别:青年科学基金项目
- 资助金额:20.0万
- 负责人:
- 依托单位:
- 学科分类:F0113.信息获取与处理
- 结题年份:2018
- 批准年份:2015
- 项目状态:已结题
- 起止时间:2016-01-01 至2018-12-31
- 项目参与者:刘恩海; 洪裕珍; 颜坤; 游迪;
- 关键词:
项目摘要
It’s proposed that the method of pose measurement of the non-cooperative target in space using monocular vision based on feature learning, and the strategy of “feature learning based on monocular vision” + “pose estimation based on feature corresponding ” is established. Firstly, the machine learning is melt into the FAST(Features from Accelerated Segment Test) and BRIEF(Binary Robust Independent Elementary Feature) to extract and match feature, which achieves feature accuracy and efficiency of extraction and matching because the feature matching problem skillfully converted to feature classification problem using the unsupervised machine learning; Then the method combined between fundamental matrix estimation and bundle adjustment is applied to establishing 3D feature library of target in image sequences, which solves reasonably the problem of maintain precision of 3D feature reconstruction with feature mismatching, and keep contradictions balance well between the complexity and the speed of reconstruction ; Finally, PPPIT (Pose from Orthography and Scaling with Iterations) and PROSAC(PROgressive random SAmple Consensus) are integrated to put forward the method of removing outliers of corresponding between 2-D image features and 3D feature library ,which improves the accuracy of pose measurement. Therefore, the study on pose measurement of the non-cooperative target using monocular vision based on feature learning will be Solid foundation in theory and practice for much space application including spacecraft RVD(rendezvous and docking) and robot capture.
本项目研究提出一种基于特征学习的空间非合作目标单目视觉位姿测量方法,并建立了“单目特征学习”+“特征对应测姿”策略。首先将机器学习思想创新融入到加速分割测试特征检测(FAST)和二元鲁棒独立基本特征(BRIEF)提取匹配过程中,将特征匹配问题巧妙转换为特征在无监督学习下的分类问题,达到特征提取匹配准确性、高效性;随后将基本矩阵估计和光束平差方法相结合用于序列图像的三维特征库建立,合理解决了误匹配下三维特征重建精度保持问题,平衡了重建速度和复杂度之间矛盾;最后提出平行投影的迭代测姿方法(PPPIT),并融合累进随机抽样一致(PROSAC)思想,提出图像二维特征与三维特征库之间2D-3D特征对应去野值方法,提高了位姿解算精度。基于特征学习的空间非合作目标单目视觉位姿测量方法研究将为航天器交会对接、机器人抓捕等空间应用打下坚实的理论基础。
结项摘要
本项目研究提出一种基于特征学习的空间非合作目标单目视觉位姿测量方法,并建立了“单目特征学习”+“特征对应测姿”策略。首先将机器学习思想创新融入到加速分割测试特征检测(FAST)和二元鲁棒独立基本特征(BRIEF)提取匹配过程中,将特征匹配问题巧妙转换为特征在无监督学习下的分类问题,达到特征提取匹配准确性、高效性;随后将基本矩阵估计和光束平差方法相结合用于序列图像的三维特征库建立,合理解决了误匹配下三维特征重建精度保持问题,平衡了重建速度和复杂度之间矛盾;最后提出平行投影的迭代测姿方法(PPPIT),并融合累进随机抽样一致(PROSAC)思想,提出图像二维特征与三维特征库之间2D-3D特征对应去野值方法,提高了位姿解算精度。基于特征学习的空间非合作目标单目视觉位姿测量方法研究将为航天器交会对接、机器人抓捕等空间应用打下坚实的理论基础。
项目成果
期刊论文数量(8)
专著数量(0)
科研奖励数量(0)
会议论文数量(0)
专利数量(3)
快速鲁棒的基础矩阵估计
- DOI:--
- 发表时间:2018
- 期刊:光学精密工程
- 影响因子:--
- 作者:颜坤;刘恩海;赵汝进;田宏;张壮
- 通讯作者:张壮
Fusion of 1D Laser Finding and monocular for Absolute Scale Estimation in SLAM
一维激光寻找和单目融合用于 SLAM 中的绝对尺度估计
- DOI:--
- 发表时间:2018
- 期刊:Sensors
- 影响因子:3.9
- 作者:ZhuangZhang;RujinZhao;Enhai Liu
- 通讯作者:Enhai Liu
A high accuracy method for pose estimation based on rotation parameters
一种基于旋转参数的高精度位姿估计方法
- DOI:10.1016/j.measurement.2018.02.013
- 发表时间:2018
- 期刊:Measurement
- 影响因子:5.6
- 作者:Yan Kun;Zhao Rujin;Tian Hong;Liu Enhai;Zhang Zhuang
- 通讯作者:Zhang Zhuang
A decoupled calibration method for camera intrinsic parameters and distortion coefficients
相机内参与畸变系数解耦标定方法
- DOI:--
- 发表时间:2016
- 期刊:Mathematical Problems in Engineering
- 影响因子:--
- 作者:Kun Yan;Hong Tian;Enhai Liu;Rujin Zhao;Yuzhen Hong;Dan Zuo
- 通讯作者:Dan Zuo
A method of color correction for Chang'E-3 satellite camera topography image
嫦娥三号卫星相机地形图像色彩校正方法
- DOI:10.3873/j.issn.1000-1328.2016.03.013
- 发表时间:2016
- 期刊:Yuhang Xuebao/Journal of Astronautics
- 影响因子:--
- 作者:Zhao Ru-Jin;Liu En-Hai;Wang Jin;Yu Guo-Bin
- 通讯作者:Yu Guo-Bin
数据更新时间:{{ journalArticles.updateTime }}
{{
item.title }}
{{ item.translation_title }}
- DOI:{{ item.doi || "--"}}
- 发表时间:{{ item.publish_year || "--" }}
- 期刊:{{ item.journal_name }}
- 影响因子:{{ item.factor || "--"}}
- 作者:{{ item.authors }}
- 通讯作者:{{ item.author }}
数据更新时间:{{ journalArticles.updateTime }}
{{ item.title }}
- 作者:{{ item.authors }}
数据更新时间:{{ monograph.updateTime }}
{{ item.title }}
- 作者:{{ item.authors }}
数据更新时间:{{ sciAawards.updateTime }}
{{ item.title }}
- 作者:{{ item.authors }}
数据更新时间:{{ conferencePapers.updateTime }}
{{ item.title }}
- 作者:{{ item.authors }}
数据更新时间:{{ patent.updateTime }}
其他文献
其他文献
{{
item.title }}
{{ item.translation_title }}
- DOI:{{ item.doi || "--" }}
- 发表时间:{{ item.publish_year || "--"}}
- 期刊:{{ item.journal_name }}
- 影响因子:{{ item.factor || "--" }}
- 作者:{{ item.authors }}
- 通讯作者:{{ item.author }}
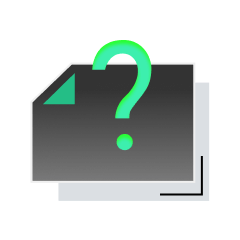
内容获取失败,请点击重试
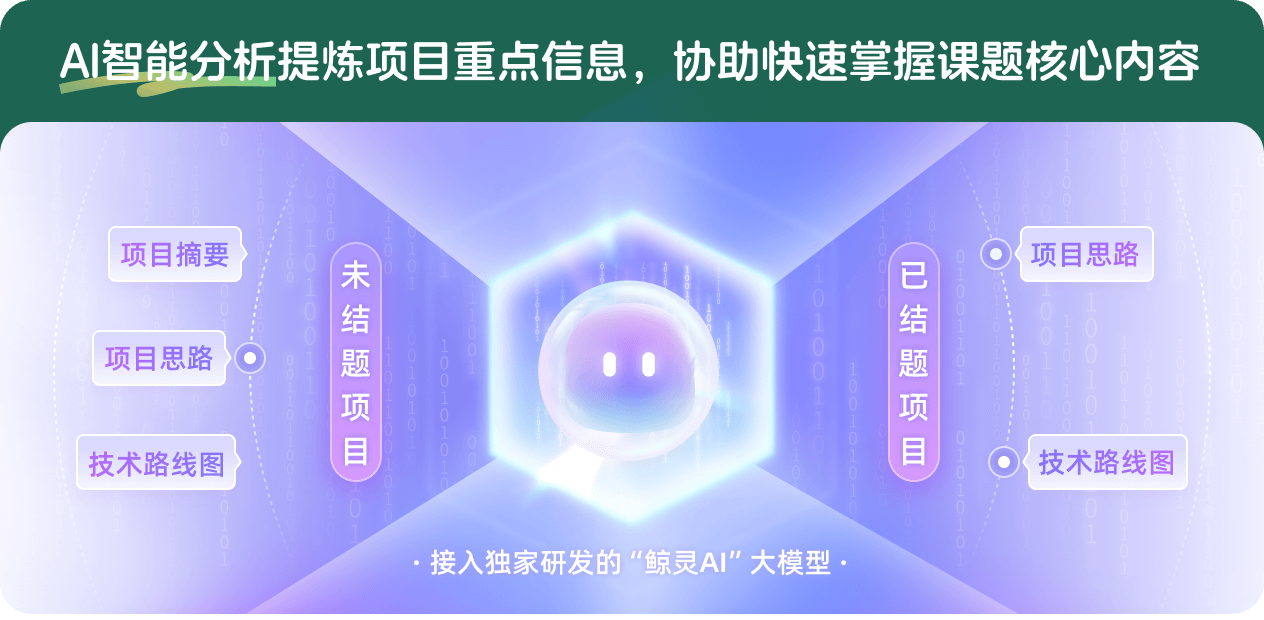
查看分析示例
此项目为已结题,我已根据课题信息分析并撰写以下内容,帮您拓宽课题思路:
AI项目摘要
AI项目思路
AI技术路线图
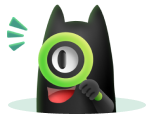
请为本次AI项目解读的内容对您的实用性打分
非常不实用
非常实用
1
2
3
4
5
6
7
8
9
10
您认为此功能如何分析更能满足您的需求,请填写您的反馈:
相似国自然基金
{{ item.name }}
- 批准号:{{ item.ratify_no }}
- 批准年份:{{ item.approval_year }}
- 资助金额:{{ item.support_num }}
- 项目类别:{{ item.project_type }}
相似海外基金
{{
item.name }}
{{ item.translate_name }}
- 批准号:{{ item.ratify_no }}
- 财政年份:{{ item.approval_year }}
- 资助金额:{{ item.support_num }}
- 项目类别:{{ item.project_type }}