面向网络化系统的分布式半监督学习研究
项目介绍
AI项目解读
基本信息
- 批准号:61871346
- 项目类别:面上项目
- 资助金额:63.0万
- 负责人:
- 依托单位:
- 学科分类:F0111.信号理论与信号处理
- 结题年份:2022
- 批准年份:2018
- 项目状态:已结题
- 起止时间:2019-01-01 至2022-12-31
- 项目参与者:李英明; 徐震; 王盛南; 王贺雨; 陈斯聪; 刘欢; 权钲杰; 黄生帅; 王涛;
- 关键词:
项目摘要
Semi-supervised learning (SSL) employs both labeled data and unlabeled data to train the classification hyperplane so as to improve the learning performance. Owing to its good performance, SSL has attracted much attention and many algorithms have been developed. By exploiting potential information of both labeled data and unlabeled data, it has been proved that the SSL can significantly improve the learning performance. ..Recently, many SSL methods have been proposed. However, most of recent studies are based on centralized processing. In many practical networked systems, because of the high communication cost, limited processing capacity, or the concern of privacy and economic profit, we cannot perform centralized processing. Distributed processing is an efficient manner to deal with in-network data processing. In distributed processing, each node shares some computation, and exchanges information with its nearest neighbors through a certain cooperative manner. Compared to the centralized processing, distributed processing can fully use the local resource of each node, reduce the data transmission, save the bandwidth and power consumption. However, the study on distributed semi-supervised learning over networked systems has not yet received much attention currently. ..Considering this, in this project, we would like to carry out a systematic study on distributed semi-supervised learning over networked systems, develop some distributed semi-supervised learning algorithms, and solve some practical problems. Moreover, considering the existence of incomplete data in many practical applications, distributed semi-supervised learning with incomplete data is also considered. We expect that by exploiting unlabeled, labeled, and incomplete labeled data distributed over multiple nodes, the performance of learning can be improved, while the transmission of the original data is avoided such that the privacy of data is protected. This study is meaningful not only for developing the theory of distributed machine learning, but also for some practical applications.
半监督学习是机器学习领域的研究热点,研究如何同时利用有类标签和无类标签样本来改进学习的性能。但目前大多数研究都是基于集中式的信息处理方式。然而,在许多实际的网络化系统中,由于通信代价高昂、处理能力有限或出于安全、商业利益等考虑,我们通常无法采用集中式处理。分布式处理是网络化系统进行信息处理的一种有效手段。在分布式处理中,每个节点分担一定的局部计算,并与其邻居节点以一定的方式进行信息交换,协作地实现数据处理。相比集中式处理,分布式处理能够充分利用各个节点的局部资源,减少了数据的传输,节省了带宽和能耗。但迄今为止,国内外在分布式半监督学习方面还鲜见文献报道。鉴于国内外研究现状,本项目拟系统地开展面向网络化系统的分布式半监督学习研究,包括在数据缺失情况下的分布式半监督学习研究,建立一套分布式半监督学习的理论和算法,解决一些实际的工程问题。本项目属于应用基础研究,具有重要的理论意义和实际应用价值。
结项摘要
半监督学习是机器学习领域的研究热点之一,半监督学习通过同时利用有类标签和无类标签样本来改进学习的性能。但目前大多数研究都采用集中式半监督学习方式。然而,在许多实际的网络化系统中,由于通信代价高昂、处理能力有限或出于安全、商业利益等考虑,我们通常无法采用集中式处理。本项目基于半监督学习和分布式信息处理的理论基础,针对网络化系统中仅有少量有类标签和大量无类标签样本的情况,系统地开展了网络化系统的分布式半监督学习研究,深度挖掘了无标签样本信息,提高学习的性能。本项目研究分别针对属性完整、属性缺失、流数据、标签歧义、正例和无标注学习(PU学习)、多标签学习、宽度学习以及在隐私保护下的分布式半监督学习等几部分内容展开研究,在不直接传输原始数据的情况下,克服了分布式实现的难点,建立了一套分布式半监督学习的理论和算法。在分布式网络中,网络中每个节点充分利用本地节点的有标签和无标签数据信息训练局部的学习模型,分担一定的局部计算,并与其邻居节点以一定的方式进行信息交换,协作地实现数据处理任务。本项目通过理论分析和大量的仿真实验,验证了提出算法的有效性。此外,考虑到实际场景中的数据隐私问题,本项目还开展了隐私保护下的分布式半监督学习研究,在保护数据隐私的同时,有效地实现了多节点的联合训练。本项目的开发有助于我们解决一些实际的工程问题,为物联网、云计算等应用提供技术支持。项目依照研究计划执行,各项研究内容都已完成,并且取得了较好的研究成果。
项目成果
期刊论文数量(9)
专著数量(0)
科研奖励数量(0)
会议论文数量(2)
专利数量(2)
Distributed Differential Utility/Cost Analysis for Privacy Protection
隐私保护的分布式差异效用/成本分析
- DOI:10.1109/lsp.2019.2932915
- 发表时间:2019-08
- 期刊:Signal Processing Letter
- 影响因子:--
- 作者:Xicong Shen;Ying Liu
- 通讯作者:Ying Liu
Privacy-Preserving Distributed Estimation Over Multitask Networks
多任务网络上的隐私保护分布式估计
- DOI:10.1109/taes.2021.3124866
- 发表时间:2022-06
- 期刊:IEEE Transactions on Aerospace and Electronic Systems
- 影响因子:--
- 作者:Shen Xicong;Liu Ying
- 通讯作者:Liu Ying
Distributed Semi-Supervised Learning With Missing Data
缺失数据的分布式半监督学习
- DOI:10.1109/tcyb.2020.2967072
- 发表时间:2020-02
- 期刊:IEEE Transactions on Cybernetics
- 影响因子:11.8
- 作者:Zhen Xu;Ying Liu;Chunguang Li
- 通讯作者:Chunguang Li
Distributed Semi-Supervised Partial Label Learning over Networks
网络上的分布式半监督部分标签学习
- DOI:10.1109/tai.2022.3148059
- 发表时间:2022
- 期刊:IEEE Transactions on Artificial Intelligence
- 影响因子:--
- 作者:Liu Ying;Xu Zhen;Zhang Chen
- 通讯作者:Zhang Chen
Robust Distributed Parameter Estimation of Nonlinear Systems With Missing Data Over Networks
网络上丢失数据的非线性系统的鲁棒分布参数估计
- DOI:10.1109/taes.2019.2948515
- 发表时间:2020-06-01
- 期刊:IEEE TRANSACTIONS ON AEROSPACE AND ELECTRONIC SYSTEMS
- 影响因子:4.4
- 作者:Chen, Sicong;Liu, Ying
- 通讯作者:Liu, Ying
数据更新时间:{{ journalArticles.updateTime }}
{{
item.title }}
{{ item.translation_title }}
- DOI:{{ item.doi || "--"}}
- 发表时间:{{ item.publish_year || "--" }}
- 期刊:{{ item.journal_name }}
- 影响因子:{{ item.factor || "--"}}
- 作者:{{ item.authors }}
- 通讯作者:{{ item.author }}
数据更新时间:{{ journalArticles.updateTime }}
{{ item.title }}
- 作者:{{ item.authors }}
数据更新时间:{{ monograph.updateTime }}
{{ item.title }}
- 作者:{{ item.authors }}
数据更新时间:{{ sciAawards.updateTime }}
{{ item.title }}
- 作者:{{ item.authors }}
数据更新时间:{{ conferencePapers.updateTime }}
{{ item.title }}
- 作者:{{ item.authors }}
数据更新时间:{{ patent.updateTime }}
其他文献
考虑信用融资的期权和实物产品最优组合采购策略
- DOI:10.13195/j.kzyjc.2015.1256
- 发表时间:2016
- 期刊:控制与决策
- 影响因子:--
- 作者:刘英;慕银平
- 通讯作者:慕银平
前列消汤对EAP湿热证模型前列腺内NF-κB表达的影响
- DOI:10.13193/j.issn.1673-7717.2020.08.032
- 发表时间:2019
- 期刊:中华中医药学刊
- 影响因子:--
- 作者:买鹏宇;徐楠;朱闽;戴芳;刘英
- 通讯作者:刘英
Vegetation Monitoring in Shendong Mining Area by Remote Sensing
神东矿区植被遥感监测
- DOI:10.14257/ijsip.2015.8.6.15
- 发表时间:2015-06
- 期刊:International Journal of Signal Processing, Image Processing and Pattern Recognition
- 影响因子:--
- 作者:刘英;岳辉
- 通讯作者:岳辉
酵母菌降解胞外聚合物提升剩余活性污泥的脱水性能
- DOI:10.13227/j.hjkx.201905001
- 发表时间:2019
- 期刊:环境科学
- 影响因子:--
- 作者:俞心怡;向金豪;刘英;龚天翼;张彬辉;吕文洲
- 通讯作者:吕文洲
过表达β-catenin诱导人表皮干细胞向成釉质细胞分化
- DOI:--
- 发表时间:2014
- 期刊:中国细胞生物学学报
- 影响因子:--
- 作者:刘英;林鑫;曾金镇;柳志坚
- 通讯作者:柳志坚
其他文献
{{
item.title }}
{{ item.translation_title }}
- DOI:{{ item.doi || "--" }}
- 发表时间:{{ item.publish_year || "--"}}
- 期刊:{{ item.journal_name }}
- 影响因子:{{ item.factor || "--" }}
- 作者:{{ item.authors }}
- 通讯作者:{{ item.author }}
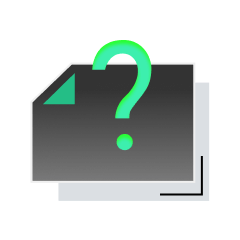
内容获取失败,请点击重试
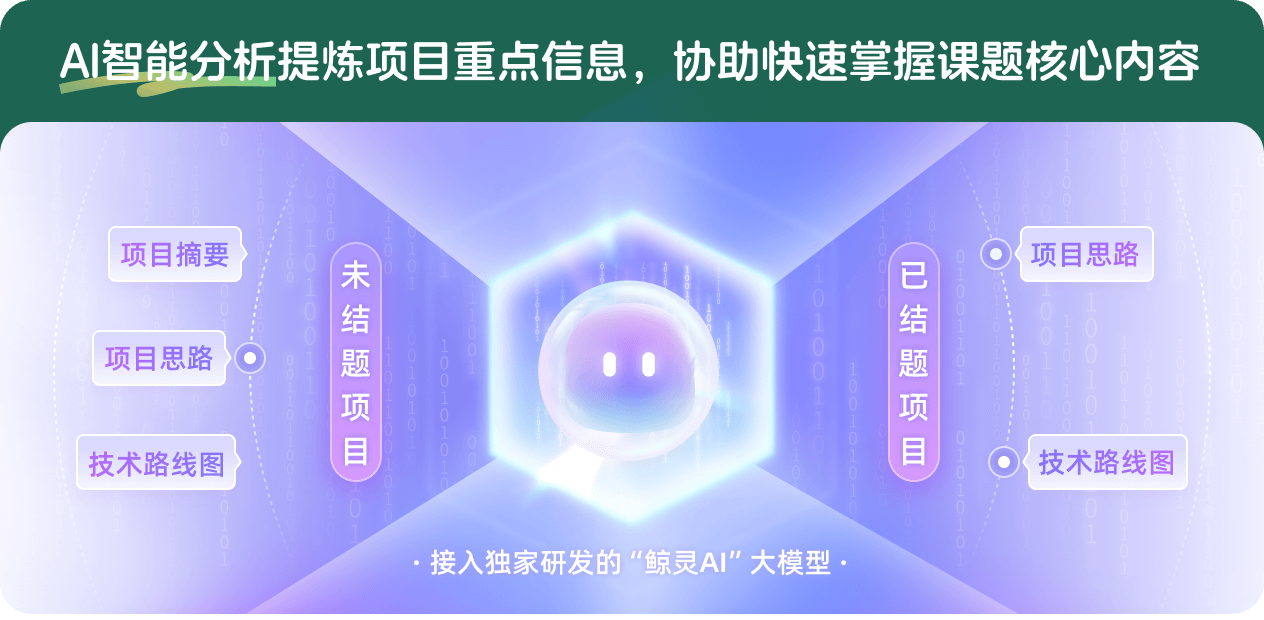
查看分析示例
此项目为已结题,我已根据课题信息分析并撰写以下内容,帮您拓宽课题思路:
AI项目摘要
AI项目思路
AI技术路线图
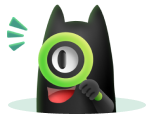
请为本次AI项目解读的内容对您的实用性打分
非常不实用
非常实用
1
2
3
4
5
6
7
8
9
10
您认为此功能如何分析更能满足您的需求,请填写您的反馈:
刘英的其他基金
传感器网络中分布式盲信号估计研究
- 批准号:61471320
- 批准年份:2014
- 资助金额:81.0 万元
- 项目类别:面上项目
复杂网络中分布式观测器的设计及应用
- 批准号:61101045
- 批准年份:2011
- 资助金额:24.0 万元
- 项目类别:青年科学基金项目
相似国自然基金
{{ item.name }}
- 批准号:{{ item.ratify_no }}
- 批准年份:{{ item.approval_year }}
- 资助金额:{{ item.support_num }}
- 项目类别:{{ item.project_type }}
相似海外基金
{{
item.name }}
{{ item.translate_name }}
- 批准号:{{ item.ratify_no }}
- 财政年份:{{ item.approval_year }}
- 资助金额:{{ item.support_num }}
- 项目类别:{{ item.project_type }}