基于深度学习的动态三维数字全息像重建方法研究
项目介绍
AI项目解读
基本信息
- 批准号:61905197
- 项目类别:青年科学基金项目
- 资助金额:24.0万
- 负责人:
- 依托单位:
- 学科分类:F0516.交叉学科中的光学问题
- 结题年份:2022
- 批准年份:2019
- 项目状态:已结题
- 起止时间:2020-01-01 至2022-12-31
- 项目参与者:--
- 关键词:
项目摘要
Digital holography is a significant imaging technique that can record the whole wavefront information of a three-dimensional (3D) object in real time. It is one of the hot topics in the optical imaging field since it can provide a simple but effective way for microscopic imaging and quantitative measurement. For the quantitative phase imaging of a dynamic 3D target, however, conventional holographic reconstruction methods can only handle one single 2D hologram in a sequential way. Afterwards, the stack of 2D reconstructed images is synthesized to a 3D volume. Although functional, the process is computational demanding and the quality is barely satisfactory. In addition, the underlying relationships between frames (i.e., holograms) are not taken into consideration and not utilized sufficiently. Both of the cumbersome operations and neglect of hidden information lead to unprecedented difficulties in the development of real-time applications with digital holography. In recent years, deep learning, or artificial intelligence, is an emerging powerful tool for image processing. It provides a promising solution since it can not only deal with 2D images, but also process video streams in an end-to-end manner. This project aims at studying the enhancement and optimization of reconstruction methods by making use of deep learning in digital holographic imaging. The three main targets that will be involved in are: 1. Design a generalized deep learning-based reconstruction method and achieve a generalized physical realization for quantitative phase imaging; 2. Design an end-to-end 3D reconstruction model for holographic 3D image reconstruction; 3. Based on high-dimensional deep learning, achieve real-time object recognition, holographic image reconstruction and tracking in dynamic 3D multi-sample scenarios. The research achievements will reveal the mechanism of applying deep learning to optical imaging. This project will also benefit to novel real-time holographic image reconstruction methods and pave the way to biological imaging and object detection in digital holography.
数字全息术能够实时记录三维物体的全部波前信息,为显微成像、定量检测提供了一种简单而有效的实现途径,因此成为光学成像领域的研究热点之一。然而对于动态三维目标的定量相位成像,传统的全息像重建算法只能对每一帧二维全息图分别重建、再进行三维合成。这种方法不但耗时长、效果差,也未充分利用帧与帧之间的内在联系,特别不利于实时动态全息应用场景的开发。深度学习不仅可以处理二维图像,还能对视频信息实现端到端式处理,有助于解决上述问题。本项目旨在研究深度学习对数字全息术中全息像重建算法的增强与优化。主要研究内容:①通用型深度学习网络架构建立及通用型定量相位成像的物理实现; ②三维物体实现端到端式三维全息像的重建方法; ③动态多目标实时检测、全息像重建以及追迹的高维度深度学习网络架构。研究成果将有助于揭示深度学习在光学成像应用中的新机制,并为发展新型实时三维全息像重建算法及其在生物目标检测中的应用提供新的途径。
结项摘要
数字全息术能够实时记录三维物体的全部波前信息,为显微成像、定量检测提供了一种简单而有效的实现途径,因此成为光学成像领域的研究热点之一。然而对于动态三维目标的定量相位成像,传统的全息像重建算法只能对每一帧二维全息图分别重建、再进行三维合成。这种方法不但耗时长、效果差,也未充分利用帧与帧之间的内在联系,特别不利于实时动态全息应用场景的开发。深度学习不仅可以处理二维图像,还能对视频信息实现端到端式处理,有助于解决上述问题。本项目旨在研究深度学习对数字全息术中全息像重建算法的增强与优化。主要研究内容:①通用型深度学习网络架构建立及通用型定量相位成像的物理实现; ②三维物体实现端到端式三维全息像的重建方法; ③动态多目标实时检测、全息像重建以及追迹的高维度深度学习网络架构。在本项目的支持下,共发表期刊论文12篇,其中包括APL Photonics一篇,Optics Express三篇,Optics and Lasers in Engineering三篇,IEEE Photonics Journal一篇,IEEE Access一篇,《光学学报》两篇,《激光与光电子学进展》一篇;会议论文2篇;已提交专利申请2项。相关研究成果将有助于揭示深度学习在光学成像应用中的新机制,并为发展新型实时三维全息像重建算法及其在生物目标检测中的应用提供新的途径。
项目成果
期刊论文数量(12)
专著数量(0)
科研奖励数量(0)
会议论文数量(2)
专利数量(0)
光学扫描全息术研究进展
- DOI:10.3788/aos202040.0111009
- 发表时间:2020
- 期刊:光学学报
- 影响因子:--
- 作者:任振波;林彥民
- 通讯作者:林彥民
Extended focused imaging in microscopy using structure tensor and guided filtering
使用结构张量和引导滤波在显微镜中扩展聚焦成像
- DOI:10.1016/j.optlaseng.2021.106549
- 发表时间:2021
- 期刊:Optics and Lasers in Engineering
- 影响因子:4.6
- 作者:Zhenbo Ren;Peiyan Guan;Edmund Y. Lam;Jianlin Zhao
- 通讯作者:Jianlin Zhao
Automatic compensation of phase aberrations in digital holographic microscopy based on sparse optimization
基于稀疏优化的数字全息显微镜相位像差自动补偿
- DOI:10.1063/1.5115079
- 发表时间:2019
- 期刊:APL Photonics
- 影响因子:5.6
- 作者:Zhenbo Ren;Jianlin Zhao;Edmund Y. Lam
- 通讯作者:Edmund Y. Lam
Object-independent tilt detection for optical sparse aperture system with large-scale piston error via deep convolution neural network
通过深度卷积神经网络对具有大规模活塞误差的光学稀疏孔径系统进行与对象无关的倾斜检测
- DOI:10.1364/oe.444501
- 发表时间:2021
- 期刊:Optics Express
- 影响因子:3.8
- 作者:Ju Tang;Zhenbo Ren;Xiaoyan Wu;Jianglei Di;Guodong Liu;Jianlin Zhao
- 通讯作者:Jianlin Zhao
Single-Shot Diffraction Autofocusing: Distance Prediction via an Untrained Physics-Enhanced Network
单次衍射自动聚焦:通过未经训练的物理增强网络进行距离预测
- DOI:10.1109/jphot.2021.3138548
- 发表时间:2022
- 期刊:IEEE Photonics Journal
- 影响因子:2.4
- 作者:Ju Tang;Ji Wu;Jiawei Zhang;Zhenbo Ren;Jianglei Di;Jianlin Zhao
- 通讯作者:Jianlin Zhao
数据更新时间:{{ journalArticles.updateTime }}
{{
item.title }}
{{ item.translation_title }}
- DOI:{{ item.doi || "--"}}
- 发表时间:{{ item.publish_year || "--" }}
- 期刊:{{ item.journal_name }}
- 影响因子:{{ item.factor || "--"}}
- 作者:{{ item.authors }}
- 通讯作者:{{ item.author }}
数据更新时间:{{ journalArticles.updateTime }}
{{ item.title }}
- 作者:{{ item.authors }}
数据更新时间:{{ monograph.updateTime }}
{{ item.title }}
- 作者:{{ item.authors }}
数据更新时间:{{ sciAawards.updateTime }}
{{ item.title }}
- 作者:{{ item.authors }}
数据更新时间:{{ conferencePapers.updateTime }}
{{ item.title }}
- 作者:{{ item.authors }}
数据更新时间:{{ patent.updateTime }}
其他文献
Secure and noise-free holographic encryption with a quick-response code
安全无噪音的全息加密,具有快速响应代码
- DOI:10.1016/j.jart.2015.10.015
- 发表时间:2024-09-14
- 期刊:Journal of Applied Research and Technology
- 影响因子:--
- 作者:任振波;苏萍;马建设;金国藩
- 通讯作者:金国藩
其他文献
{{
item.title }}
{{ item.translation_title }}
- DOI:{{ item.doi || "--" }}
- 发表时间:{{ item.publish_year || "--"}}
- 期刊:{{ item.journal_name }}
- 影响因子:{{ item.factor || "--" }}
- 作者:{{ item.authors }}
- 通讯作者:{{ item.author }}
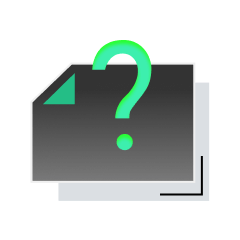
内容获取失败,请点击重试
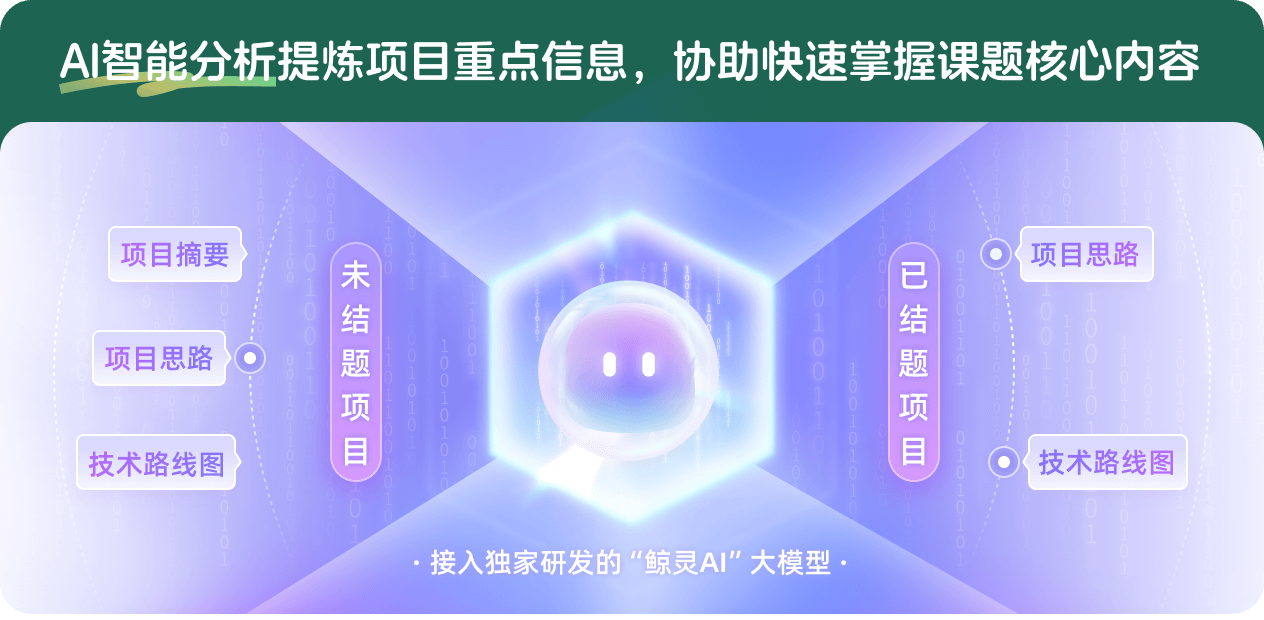
查看分析示例
此项目为已结题,我已根据课题信息分析并撰写以下内容,帮您拓宽课题思路:
AI项目摘要
AI项目思路
AI技术路线图
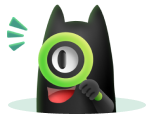
请为本次AI项目解读的内容对您的实用性打分
非常不实用
非常实用
1
2
3
4
5
6
7
8
9
10
您认为此功能如何分析更能满足您的需求,请填写您的反馈:
任振波的其他基金
基于波前调制和深度学习的显微成像虚拟重聚焦方法研究
- 批准号:
- 批准年份:2022
- 资助金额:52 万元
- 项目类别:面上项目
相似国自然基金
{{ item.name }}
- 批准号:{{ item.ratify_no }}
- 批准年份:{{ item.approval_year }}
- 资助金额:{{ item.support_num }}
- 项目类别:{{ item.project_type }}
相似海外基金
{{
item.name }}
{{ item.translate_name }}
- 批准号:{{ item.ratify_no }}
- 财政年份:{{ item.approval_year }}
- 资助金额:{{ item.support_num }}
- 项目类别:{{ item.project_type }}