微生物大世界
项目介绍
AI项目解读
基本信息
- 批准号:30720001
- 项目类别:专项基金项目
- 资助金额:35.0万
- 负责人:
- 依托单位:
- 学科分类:C01.微生物学
- 结题年份:2009
- 批准年份:2007
- 项目状态:已结题
- 起止时间:2008-01-01 至2009-12-31
- 项目参与者:姚一建; 翟红; 张玮; 程晓; 李丽; 董彩虹; 黄满容;
- 关键词:
项目摘要
微生物是地球上生物多样性最为丰富的自然资源,微生物直接或间接地影响着地球生物圈的物质循环和能量转换。微生物涵盖了有益、有害的众多种类,广泛涉及健康、医药、工农业、环保等诸多领域,"无处不在"的微生物实际上关系到人类的生存质量。然而我国各科普场、馆关于微生物的专题展览很少,公众对与人类"生死相依"的微生物知之不多。我们拟举办"微生物大世界"专题展览,并以基金委资助的多项有关药用、食用等重要经济价值的
结项摘要
项目成果
期刊论文数量(0)
专著数量(0)
科研奖励数量(0)
会议论文数量(0)
专利数量(0)
数据更新时间:{{ journalArticles.updateTime }}
{{
item.title }}
{{ item.translation_title }}
- DOI:{{ item.doi || "--"}}
- 发表时间:{{ item.publish_year || "--" }}
- 期刊:{{ item.journal_name }}
- 影响因子:{{ item.factor || "--"}}
- 作者:{{ item.authors }}
- 通讯作者:{{ item.author }}
数据更新时间:{{ journalArticles.updateTime }}
{{ item.title }}
- 作者:{{ item.authors }}
数据更新时间:{{ monograph.updateTime }}
{{ item.title }}
- 作者:{{ item.authors }}
数据更新时间:{{ sciAawards.updateTime }}
{{ item.title }}
- 作者:{{ item.authors }}
数据更新时间:{{ conferencePapers.updateTime }}
{{ item.title }}
- 作者:{{ item.authors }}
数据更新时间:{{ patent.updateTime }}
其他文献
大连湾60多年来生态环境地质演化
- DOI:--
- 发表时间:--
- 期刊:地质学报
- 影响因子:--
- 作者:林景星;张静;史世云;林防;王绍芳
- 通讯作者:王绍芳
其他文献
{{
item.title }}
{{ item.translation_title }}
- DOI:{{ item.doi || "--" }}
- 发表时间:{{ item.publish_year || "--"}}
- 期刊:{{ item.journal_name }}
- 影响因子:{{ item.factor || "--" }}
- 作者:{{ item.authors }}
- 通讯作者:{{ item.author }}
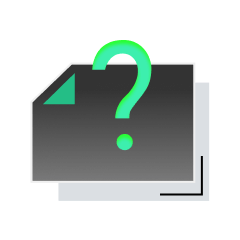
内容获取失败,请点击重试
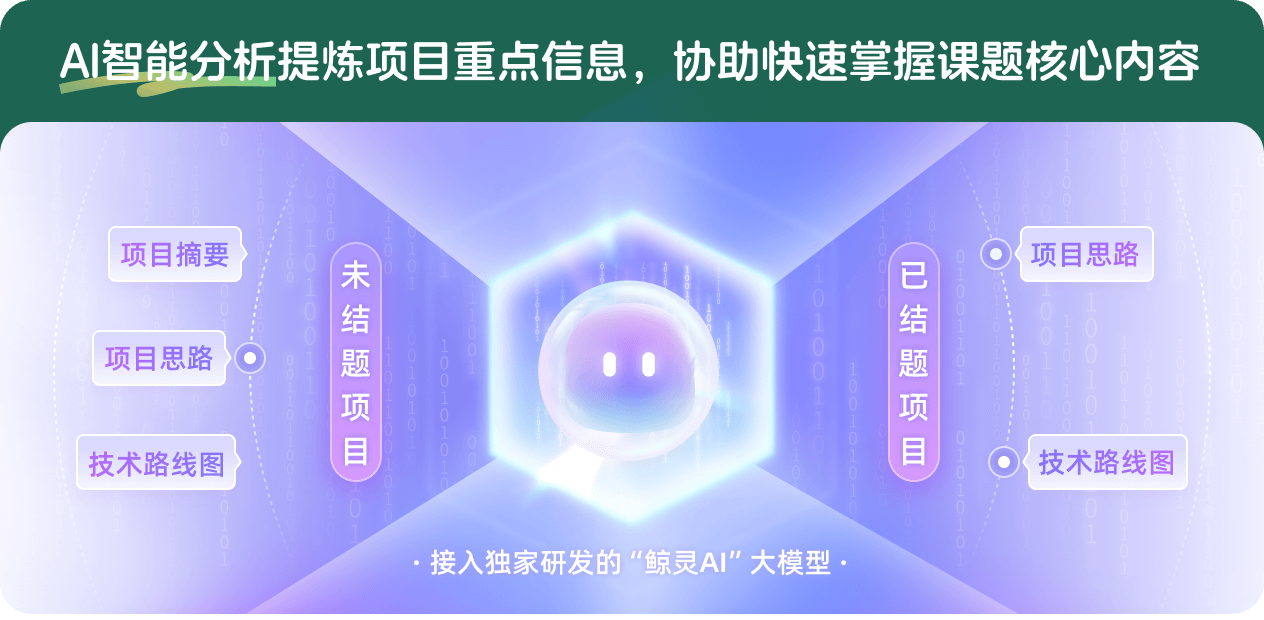
查看分析示例
此项目为已结题,我已根据课题信息分析并撰写以下内容,帮您拓宽课题思路:
AI项目摘要
AI项目思路
AI技术路线图
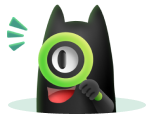
请为本次AI项目解读的内容对您的实用性打分
非常不实用
非常实用
1
2
3
4
5
6
7
8
9
10
您认为此功能如何分析更能满足您的需求,请填写您的反馈:
王绍芳的其他基金
甘肃河西走廊石炭纪群落古生态研究及海平面变化
- 批准号:49302021
- 批准年份:1993
- 资助金额:9.0 万元
- 项目类别:青年科学基金项目
相似国自然基金
{{ item.name }}
- 批准号:{{ item.ratify_no }}
- 批准年份:{{ item.approval_year }}
- 资助金额:{{ item.support_num }}
- 项目类别:{{ item.project_type }}
相似海外基金
{{
item.name }}
{{ item.translate_name }}
- 批准号:{{ item.ratify_no }}
- 财政年份:{{ item.approval_year }}
- 资助金额:{{ item.support_num }}
- 项目类别:{{ item.project_type }}