Guided Analytics for the Visual Exploration of Higher Dimensional Data
高维数据可视化探索的引导分析
基本信息
- 批准号:RGPIN-2022-03894
- 负责人:
- 金额:$ 1.31万
- 依托单位:
- 依托单位国家:加拿大
- 项目类别:Discovery Grants Program - Individual
- 财政年份:2022
- 资助国家:加拿大
- 起止时间:2022-01-01 至 2023-12-31
- 项目状态:已结题
- 来源:
- 关键词:
项目摘要
With modern data, size matters. Large numbers, perhaps millions, of observations are available for almost every variable we might measure, and, increasingly, it has become routine to measure 100s, even 1000s of variables on each observation. For example, imagine looking at daily closing prices of S&P 500 stocks over a five-year period. The data consists of about 1,250 (= 5 x 250) days (or observations) and about 500 stocks (or variables). These counts could be dramatically larger, if, for example, hourly prices were recorded and for each hour the opening, closing, and average prices for that hour were recorded for every stock. In examining such data, we hope to find patterns, to uncover something not previously anticipated, to encounter an "aha!" moment of scientific discovery. To this end, the human visual system has evolved to literally spot the unusual, to notice patterns, and to see relations. Computer interactive data visualization literally allows one "to see" what is going on in the data. But the size of the data is overwhelming. To simply look at the relation between every pair of stocks, we would have to look at 124,750 plots! It is not possible. The proposed research is to bring mathematical structure, computational resources, and statistical modelling to bear on the problem, to have the computer guide the analyst to view only those few plots (even 100 would be a saving) that might be "interesting" to look at. It will provide not just the means to find these, but the technology to efficiently view them, and incorporate them into a report. This requires determining which plots are "interesting", and what we might calculate on the data to tell that a plot was interesting. What is interesting in some analyses is not interesting in others, so many different measures of "interestingness" must be considered. This research proposes to develop several such measures that are of wide applicability as well as some that are peculiar to selected applications. Once we have calculated different measures of interestingness on our 124,750 possible plots, we must select amongst them. The research is providing tools for such selection. Once we have selected our subsets of 10s or 100s of interesting plots, we need to understand how they are related, one pair of variables to another, and what we might infer from those relations if anything. Here, the research brings mathematical graph theory to provide a structure relating the plots to one another. Analysis of that structure might then shed light on the relations between variables. Probability and statistical models of these structures will be developed to help ensure our inferences are reliable. Throughout, software will be designed and disseminated (through open-source licensing) to the general public. By putting the software in the hands of the data analysts we hope to make exploratory visualization, data analysis, and scientific discovery a little easier.
使用现代数据,大小很重要。几乎可以衡量的每个变量,大量的观察值可供选择,并且越来越多地测量100秒,甚至在每个观察结果上甚至有1000次变量。例如,想象一下,在五年期间,每天关闭标准普尔500股股票的每日收盘价。数据包括约1,250(= 5 x 250)天(或观察)和约500个股票(或变量)。这些计数可能会大大更大,例如,如果记录小时价格,并且每小时记录了每个股票的开放,收盘和平均价格。在检查此类数据时,我们希望找到模式,揭示以前没有预料的东西,遇到“啊哈!”科学发现的时刻。为此,人类的视觉系统已经演变为从字面上发现了不寻常的,注意到模式并看到关系。计算机交互式数据可视化实际上允许一个“查看”数据中发生的事情。但是数据的大小是压倒性的。要简单地查看每对股票之间的关系,我们必须查看124,750个地块!不可能。拟议的研究是将数学结构,计算资源和统计建模带入问题上,以使计算机指导分析师仅查看那些少数图(即使是100个储蓄),这些图可能会“有趣”。它不仅提供找到这些方法的手段,而且还将提供有效查看它们并将其纳入报告的技术。这需要确定哪些图“有趣”,以及我们可以在数据上计算的内容,以说明绘图很有趣。在某些分析中有趣的是,在其他分析中并不有趣,因此必须考虑许多不同的“有趣”度量。这项研究建议开发几种具有广泛适用性的措施,以及某些对选定应用程序特有的措施。一旦我们计算了124,750个可能的情节中的不同兴趣度量,我们就必须在其中选择。该研究为这种选择提供了工具。一旦我们选择了10或100s有趣的图的子集,我们就需要了解它们的相关性,一对变量到另一个变量,以及如果有的话,我们可以从这些关系中推断出来。在这里,该研究带来了数学图理论,以提供将阴谋相关的结构。然后,对该结构的分析可能会阐明变量之间的关系。将开发这些结构的概率和统计模型,以帮助确保我们的推论可靠。在整个过程中,软件将被设计和传播(通过开源许可)给公众。通过将软件掌握在数据分析师的手中,我们希望使探索性可视化,数据分析和科学发现更加容易一些。
项目成果
期刊论文数量(0)
专著数量(0)
科研奖励数量(0)
会议论文数量(0)
专利数量(0)
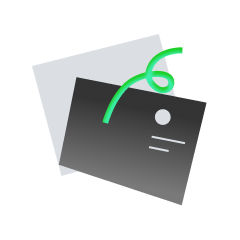
暂无数据
数据更新时间:2024-06-01
相似国自然基金
教室课堂中基于多视觉分析的情感投入检测研究
- 批准号:62307009
- 批准年份:2023
- 资助金额:30 万元
- 项目类别:青年科学基金项目
复杂视觉模式的自适应表征学习方法
- 批准号:62376291
- 批准年份:2023
- 资助金额:50.00 万元
- 项目类别:面上项目
基于流形分析的遥感图像视觉信息文字解译
- 批准号:62301161
- 批准年份:2023
- 资助金额:30.00 万元
- 项目类别:青年科学基金项目
面向全景内容的视觉质量智能分析
- 批准号:62372042
- 批准年份:2023
- 资助金额:50 万元
- 项目类别:面上项目
人与物体交互关系驱动的第一视角视频分析和视觉问答
- 批准号:62372014
- 批准年份:2023
- 资助金额:50 万元
- 项目类别:面上项目
相似海外基金
CAREER: Promoting Metacognition in Visual Analytics
职业:促进视觉分析中的元认知
- 批准号:23405392340539
- 财政年份:2024
- 资助金额:$ 1.31万$ 1.31万
- 项目类别:Continuing GrantContinuing Grant
Cloud-Based Machine Learning and Biomarker Visual Analytics for Salivary Proteomics
基于云的机器学习和唾液蛋白质组生物标志物可视化分析
- 批准号:1082764910827649
- 财政年份:2023
- 资助金额:$ 1.31万$ 1.31万
- 项目类别:
Cell Therapy Program with Scale-up cGMP Manufacturing of Human Corneal Stromal Stem Cells
细胞治疗计划,扩大人类角膜基质干细胞的 cGMP 生产
- 批准号:1072056210720562
- 财政年份:2023
- 资助金额:$ 1.31万$ 1.31万
- 项目类别:
Visual Analytics for Exploration and Hypothesis Generation Using Highly MultiplexedSpatial Data of Tissues and Tumors
使用组织和肿瘤的高度多重空间数据进行探索和假设生成的可视化分析
- 批准号:1074332910743329
- 财政年份:2023
- 资助金额:$ 1.31万$ 1.31万
- 项目类别:
Developing Machine Learning Models for Decision Support and Allocation Optimization in Heart Transplantation
开发用于心脏移植决策支持和分配优化的机器学习模型
- 批准号:1073534810735348
- 财政年份:2023
- 资助金额:$ 1.31万$ 1.31万
- 项目类别: