Somatic Repeat Expansions as a Therapeutic Target for Trinucleotide Repeat Disorders
体细胞重复扩增作为三核苷酸重复疾病的治疗靶点
基本信息
- 批准号:10605261
- 负责人:
- 金额:$ 41.28万
- 依托单位:
- 依托单位国家:美国
- 项目类别:
- 财政年份:2022
- 资助国家:美国
- 起止时间:2022-04-15 至 2027-03-31
- 项目状态:未结题
- 来源:
- 关键词:
项目摘要
ABSTRACT
Huntington’s disease (HD) and Friedreich ataxia (FA) are rare neurodegenerative diseases caused
by expanded trinucleotide repeats (CAG and GAA, respectively) in the HTT and FXN genes,
respectively, with larger alleles being associated with earlier disease onset and more severe clinical
phenotypes. Despite these being single gene disorders, where the respective underlying genetic
mutations have been known for over 20 years, there remains no cure or disease-modifying therapies,
indicating that novel approaches are critical. A hallmark of most repeat expansion disorders is that the
repeats are highly unstable, both intergenerationally (parent to child) and in somatic tissues, where the
repeat expands progressively over time in a cell-/tissue-specific manner. Notably, in HD, medium-spiny
neurons of the striatum, which succumb most severely to the effects of the HTT mutation, exhibit the
most dramatic CAG expansions. Similarly, larger GAA repeat expansions have been reported in the
heart and dorsal root ganglia of FA patients, where such tissues are most severely affected. These
observations, together with growing evidence from GWAS and candidate gene association studies in
HD patients, support the hypothesis that progressive repeat length increases in somatic tissues
contribute to the pathogenic process. Thus, understanding the roles of disease modifiers in somatic
repeat expansion may provide novel targets for therapeutic intervention directed at the repeat mutation
itself. To that end, we have leveraged a CRISPR-based in vivo system, recently developed by us, to
screen a number of candidate DNA repair genes and determine their role as potential modifiers of
somatic CAG repeat instability in HD. Remarkably, this has resulted in the identification of novel genes,
which when knocked out in the liver of HD mice, reduced CAG expansions and promoted contractions.
We hereby propose a set of experiments aimed at: 1) Identifying non-invasive samples to study
CAG repeat instability as a potential biomarker of disease, as well as developing novel long-read
sequencing-based methodologies to more accurately size and quantify repeat instability; 2) Validating
candidate modifier genes in a new model of CAG expansions using HD patient-derived fibroblasts,
recently developed by us, as well as understand the potential adverse implications associated with
inactivating such DNA repair genes. We also propose to investigate if such genes are equally involved
in FA GAA expansions, using the same in vivo CRISPR platform and patient derived cellular models;
3) Development and testing of novel antisense oligonucleotide- and CRISPR-based therapeutic
approaches targeting the repeat expansion process to suppress repeat expansions or actually promote
contractions. This will lead to a better understanding of shared mechanisms across these diseases and
potentially result in novel therapeutics that can be used in all repeat expansion disorders.
抽象的
亨廷顿氏病(HD)和弗里德里希共济失调(FA)是罕见的神经退行性疾病
通过在HTT和FXN基因中分别扩展的三核苷酸重复序列(CAG和GAA),
分别较大的等位基因与较早的疾病发作和更严重的临床有关
表型。尽管这些是单个基因疾病,其中相对基础遗传
突变已有20多年了,尚无治愈或修改疾病的疗法,
表明新方法至关重要。大多数重复扩张障碍的标志是
重复次数是高度不稳定的,既有代间(父母对儿童)和在体细胞组织中,
重复以细胞/组织特异性方式逐渐扩展。值得注意的是,在高清中
纹状体的神经元最严重地屈服于HTT突变的作用,暴露了
最戏剧性的CAG扩展。同样,据报道了较大的GAA重复扩展
FA患者的心脏和背根神经节,这些组织受到最严重影响。这些
观察结果,以及GWAS和候选基因关联研究的越来越多的证据
高清患者,支持以下假设
有助于致病过程。这是了解疾病修饰剂在躯体中的作用
重复扩展可能为针对重复突变的治疗干预提供新的靶标
本身。为此,我们利用了一个基于CRISPR的体内系统,该系统最近由我们开发,用于
筛选许多候选DNA修复基因,并确定其作为潜在修饰符的作用
体细胞CAG在高清中重复不稳定性。值得注意的是,这导致了新基因的鉴定,
当在HD小鼠的肝脏中撞倒时,CAG膨胀并促进了收缩。
我们在此提出了一组针对以下实验:1)识别非侵入性样本以研究
CAG重复不稳定性作为潜在的疾病生物标志物,并发展了新的长阅读
基于测序的方法更准确地大小并量化重复不稳定性; 2)验证
使用HD患者衍生的成纤维细胞的新型CAG扩展模型中的候选修饰符基因,
我们最近开发的,以及了解与
灭活这种DNA修复基因。我们还建议调查此类基因是否同样涉及
在FA GAA扩展中,使用相同的体内CRISPR平台和患者衍生的细胞模型;
3)新型反义寡核苷酸和基于CRISPR的疗法的开发和测试
针对重复扩展过程以抑制重复扩展或实际促进的方法
收缩。这将使人们更好地了解这些疾病中的共同机制,并
可能导致新的疗法可用于所有重复扩张障碍。
项目成果
期刊论文数量(0)
专著数量(0)
科研奖励数量(0)
会议论文数量(0)
专利数量(0)
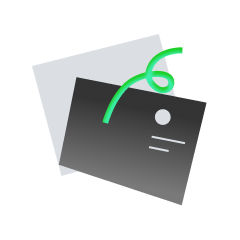
暂无数据
数据更新时间:2024-06-01
相似国自然基金
KIR3DL1等位基因启动子序列变异影响其差异表达的分子机制研究
- 批准号:82200258
- 批准年份:2022
- 资助金额:30.00 万元
- 项目类别:青年科学基金项目
KIR3DL1等位基因启动子序列变异影响其差异表达的分子机制研究
- 批准号:
- 批准年份:2022
- 资助金额:30 万元
- 项目类别:青年科学基金项目
NUP205双等位基因突变影响纤毛发生而致内脏转位合并先天性心脏病的机理研究
- 批准号:82171845
- 批准年份:2021
- 资助金额:54.00 万元
- 项目类别:面上项目
NUP205双等位基因突变影响纤毛发生而致内脏转位合并先天性心脏病的机理研究
- 批准号:
- 批准年份:2021
- 资助金额:54 万元
- 项目类别:面上项目
全基因组范围内揭示杂交肉兔等位基因特异性表达模式对杂种优势遗传基础的影响
- 批准号:32102530
- 批准年份:2021
- 资助金额:30 万元
- 项目类别:青年科学基金项目
相似海外基金
Genetic and Environmental Influences on Individual Sweet Preference Across Ancestry Groups in the U.S.
遗传和环境对美国不同血统群体个体甜味偏好的影响
- 批准号:1070938110709381
- 财政年份:2023
- 资助金额:$ 41.28万$ 41.28万
- 项目类别:
Multi-omic phenotyping of human transcriptional regulators
人类转录调节因子的多组学表型分析
- 批准号:1073315510733155
- 财政年份:2023
- 资助金额:$ 41.28万$ 41.28万
- 项目类别:
Genetic Dissection of Stress Responses in Shwachman-Diamond Syndrome
什瓦赫曼-戴蒙德综合征应激反应的基因剖析
- 批准号:1059436610594366
- 财政年份:2023
- 资助金额:$ 41.28万$ 41.28万
- 项目类别:
The immunogenicity and pathogenicity of HLA-DQ in solid organ transplantation
HLA-DQ在实体器官移植中的免疫原性和致病性
- 批准号:1065866510658665
- 财政年份:2023
- 资助金额:$ 41.28万$ 41.28万
- 项目类别:
Effects of Aging on Neuronal Lysosomal Damage Responses Driven by CMT2B-linked Rab7
衰老对 CMT2B 相关 Rab7 驱动的神经元溶酶体损伤反应的影响
- 批准号:1067878910678789
- 财政年份:2023
- 资助金额:$ 41.28万$ 41.28万
- 项目类别: