MOLECULAR BASIS OF VIRAL PATHOGENESIS
病毒发病的分子基础
基本信息
- 批准号:2058144
- 负责人:
- 金额:$ 12.28万
- 依托单位:
- 依托单位国家:美国
- 项目类别:
- 财政年份:1989
- 资助国家:美国
- 起止时间:1989-09-30 至 2000-04-30
- 项目状态:已结题
- 来源:
- 关键词:
项目摘要
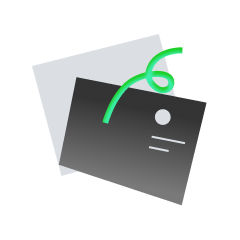
暂无数据
项目成果
期刊论文数量(0)
专著数量(0)
科研奖励数量(0)
会议论文数量(0)
专利数量(0)
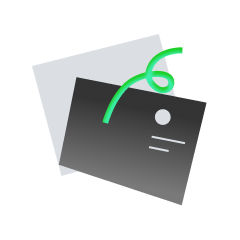
暂无数据
数据更新时间:2024-06-01
Michael Joseph Buc...的其他基金
Vaccines and Antivirals for Arenaviruses
沙粒病毒疫苗和抗病毒药物
- 批准号:82602518260251
- 财政年份:2011
- 资助金额:$ 12.28万$ 12.28万
- 项目类别:
CRYO-EM STRUCTURAL STUDIES OF FELINE, MURINE, AVIAN AND SARS CORONA VIRUSES
猫科动物、鼠科动物、禽类和 SARS 冠状病毒的冷冻电镜结构研究
- 批准号:83624818362481
- 财政年份:2011
- 资助金额:$ 12.28万$ 12.28万
- 项目类别:
Vaccines and Antivirals for Arenaviruses
沙粒病毒疫苗和抗病毒药物
- 批准号:76751697675169
- 财政年份:2009
- 资助金额:$ 12.28万$ 12.28万
- 项目类别:
Human T-cell Epitopes in SARS
SARS 中的人类 T 细胞表位
- 批准号:72200337220033
- 财政年份:2005
- 资助金额:$ 12.28万$ 12.28万
- 项目类别:
Human T-cell Epitopes in SARS
SARS 中的人类 T 细胞表位
- 批准号:74269377426937
- 财政年份:2005
- 资助金额:$ 12.28万$ 12.28万
- 项目类别:
Human T-cell Epitopes in SARS
SARS 中的人类 T 细胞表位
- 批准号:70863827086382
- 财政年份:2005
- 资助金额:$ 12.28万$ 12.28万
- 项目类别:
Human T-cell Epitopes in SARS
SARS 中的人类 T 细胞表位
- 批准号:69689516968951
- 财政年份:2005
- 资助金额:$ 12.28万$ 12.28万
- 项目类别:
相似国自然基金
基于“成分-含量-功效”和“菌-肠-肺”轴关联分析策略的双黄连抗流感病毒性肺炎功效物质基础研究
- 批准号:
- 批准年份:2022
- 资助金额:30 万元
- 项目类别:青年科学基金项目
基于“成分-含量-功效”和“菌-肠-肺”轴关联分析策略的双黄连抗流感病毒性肺炎功效物质基础研究
- 批准号:82204611
- 批准年份:2022
- 资助金额:30.00 万元
- 项目类别:青年科学基金项目
基于呼吸道黏膜防御机制探讨中医药防治病毒性肺炎的现代生物学机制及药效物质基础
- 批准号:82141206
- 批准年份:2021
- 资助金额:240 万元
- 项目类别:专项基金项目
基于病毒性肺炎发病过程探讨中药复方多靶点协同防治的物质基础与作用机制
- 批准号:
- 批准年份:2021
- 资助金额:60 万元
- 项目类别:专项基金项目
基于病毒性肺炎发病过程探讨中药复方多靶点协同防治的物质基础与作用机制
- 批准号:82141207
- 批准年份:2021
- 资助金额:60.00 万元
- 项目类别:专项项目
相似海外基金
The molecular basis of viral tolerance in bats
蝙蝠病毒耐受的分子基础
- 批准号:BB/Y003772/1BB/Y003772/1
- 财政年份:2024
- 资助金额:$ 12.28万$ 12.28万
- 项目类别:Research GrantResearch Grant
The molecular basis of viral tolerance in bats
蝙蝠病毒耐受的分子基础
- 批准号:BB/Y005473/1BB/Y005473/1
- 财政年份:2024
- 资助金额:$ 12.28万$ 12.28万
- 项目类别:Research GrantResearch Grant
Molecular basis and protective efficacy of cross-neutralizing antibodies against four major respiratory viruses
四种主要呼吸道病毒交叉中和抗体的分子基础和保护功效
- 批准号:1065792610657926
- 财政年份:2023
- 资助金额:$ 12.28万$ 12.28万
- 项目类别:
Understanding the Molecular Basis of Translation Inhibition by SARS-CoV-2 NSP14 and its Role in SARS-CoV-2 Immune Evasion
了解 SARS-CoV-2 NSP14 翻译抑制的分子基础及其在 SARS-CoV-2 免疫逃避中的作用
- 批准号:1042768810427688
- 财政年份:2023
- 资助金额:$ 12.28万$ 12.28万
- 项目类别:
Molecular basis of mammalian cochlear regeneration
哺乳动物耳蜗再生的分子基础
- 批准号:1068227210682272
- 财政年份:2023
- 资助金额:$ 12.28万$ 12.28万
- 项目类别: