Computational Image Analysis of Renal Transplant Biopsies to Predict Graft Outcome
肾移植活检的计算图像分析以预测移植结果
基本信息
- 批准号:10733292
- 负责人:
- 金额:$ 60.5万
- 依托单位:
- 依托单位国家:美国
- 项目类别:
- 财政年份:2023
- 资助国家:美国
- 起止时间:2023-09-06 至 2028-07-31
- 项目状态:未结题
- 来源:
- 关键词:
项目摘要
Project Summary
Kidney transplantation is the most effective modality for treating end stage kidney disease. It provides superior
quality of life and significantly improves survival over dialysis. However, the demand for kidney transplants has
surpassed the supply of usable organs. Because of this deficit, it is important to improve the outcomes of first-
time transplant recipients through intelligent management, thereby optimizing donor organ allocation and
reducing the need for secondary transplants. In assessing the health of a renal allograft, time is of critical
importance. Being able to precisely predict delayed graft dysfunction and modifying treatment strategies
accordingly would be greatly impactful in decreasing chronic rejection events. Existing clinical methods, such as
the Kidney Donor Profile Index, which are based solely on donor demographics and clinical data, are minimally
to moderately predictive of allograft outcomes. Further, current visual, semi-quantitative transplant biopsy scoring
metrics, e.g., Banff, the Maryland Aggregate Pathology Index, and Remuzzi are often not predictive of renal graft
function. Digital image analytical methods that quantify chronic changes in kidney that cannot be done visually,
may offer clues to long-term allograft outcome. Therefore, to address the unmet need of intelligent renal
transplant management, we propose a comprehensive multimodal framework, integrating high-resolution renal
transplant biopsy digital whole-slide images (WSIs), and donor and recipient clinical, demographic, and social
determinants of health data. Using this framework we will combine computer vision and explainable artificial
intelligence (XAI) tools to derive autonomous diagnostic and prognostic models for data-driven, long-term
management of renal allografts. As part of their preliminary work, the investigator team has developed a
computational tool to quantify interstitial fibrosis and tubular atrophy, a chronicity measure in renal transplant
biopsies, and demonstrated that the prediction of estimated glomerular filtration rate at a later time-point after
biopsy using machine learning (ML)-derived image features outperforms those based on routine visual
assessment. This tool will be expanded to incorporate a variety of additional analyses including robust
segmentation of renal compartments in WSIs, leveraging pathologist guided attention to train deep-learning
models, state-of-the-art transformer models for multi-task learning, and XAI to increase interoperability and
accessibility of ML-derived predictions to pathologists. The performance of this pipeline to predict renal allograft
function in a future time-point will be compared with existing methods used in a clinical setting as well as ML-
based methods used for explainable prediction of disease progression in other areas of digital pathology. The
tool will be deployed on a cloud-based platform and the usability by important stakeholders, namely, transplant
renal pathologists, nephrologists, and surgeons will be studied with a goal to eventually include the tool in clinical
workflows. The proposed work will be an invaluable asset for clinicians to take advantage of large collections of
renal transplant biopsy WSIs and inform treatment decisions towards improving renal allograft function.
项目摘要
肾脏移植是治疗末期肾脏疾病的最有效方式。它提供了优势
生活质量并显着改善了透析的生存。但是,对肾脏移植的需求有
超过了可用器官的供应。由于这种赤字,重要的是要提高首先的结果
通过智能管理的时间移植接收者,从而优化捐赠者器官分配和
减少对二次移植的需求。在评估肾脏同种异体移植的健康时,时间至关重要
重要性。能够精确预测延迟的移植功能障碍并修改治疗策略
因此,在减少慢性拒绝事件方面会产生很大的影响。现有的临床方法,例如
仅基于供体人口统计学和临床数据的肾脏供体轮廓指数是最小的
适度地预测同种异体的结果。此外,当前的视觉,半定量移植活检评分
指标,例如班夫,马里兰州骨料病理学指数和Remuzzi通常无法预测肾脏移植物
功能。数字图像分析方法可以量化无法视觉上无法完成肾脏的慢性变化,
可能会提供长期同种异体结果的线索。因此,解决未满足的智能肾脏的需求
移植管理,我们提出了一个全面的多模式框架,整合了高分辨率肾脏
移植活检数字全滑动图像(WSIS)以及捐助者和接受者临床,人口和社交
健康数据的决定因素。使用此框架,我们将结合计算机视觉和可解释的人工
智能(XAI)工具,用于得出数据驱动的长期自主诊断和预后模型
肾脏同种异体的管理。作为他们初步工作的一部分,调查员团队已经开发了
量化间质纤维化和管状萎缩的计算工具,这是肾移植中的慢性测量
活检,并证明了估计的肾小球滤过率以后的预测
使用机器学习(ML)衍生的图像功能的活检优于基于常规视觉的图像特征
评估。该工具将扩展,以结合各种其他分析,包括可靠的
WSIS肾脏室的分割,利用病理学家引导注意力训练深入学习
模型,用于多任务学习的最先进的变压器模型以及XAI,以增加互操作性和
ML衍生的病理学家的可及性。该管道的性能预测肾脏同种异体移植
将来的时间点的功能将与临床环境中使用的现有方法以及ML-进行比较
用于解释数字病理其他领域疾病进展的基于可解释的方法。这
工具将部署在基于云的平台上,并由重要的利益相关者(即移植)部署
将研究肾脏病理学家,肾病学家和外科医生,目标最终包括该工具
工作流程。拟议的工作将是临床医生利用大量收藏的宝贵资产
肾脏移植活检WSIS,并为改善肾脏同种异体功能的治疗决定提供信息。
项目成果
期刊论文数量(0)
专著数量(0)
科研奖励数量(0)
会议论文数量(0)
专利数量(0)
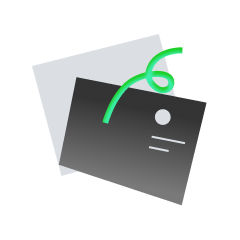
暂无数据
数据更新时间:2024-06-01
相似国自然基金
卫星互联网端到端安全传输模型与安全路由协议研究
- 批准号:62302389
- 批准年份:2023
- 资助金额:30 万元
- 项目类别:青年科学基金项目
中继通信协议下2-D网络化系统的递推状态估计研究
- 批准号:62373103
- 批准年份:2023
- 资助金额:50 万元
- 项目类别:面上项目
新型实用化量子密码协议的高安全等级理论分析
- 批准号:12374473
- 批准年份:2023
- 资助金额:52 万元
- 项目类别:面上项目
云边端架构下联邦学习下行通信压缩算法与协议研究
- 批准号:62372487
- 批准年份:2023
- 资助金额:50.00 万元
- 项目类别:面上项目
面向实际应用的测量设备无关类量子密钥分发协议研究
- 批准号:62371244
- 批准年份:2023
- 资助金额:53.00 万元
- 项目类别:面上项目
相似海外基金
Exploiting Cancer Metabolism and Drug Efflux with Bystander-Assisted Immunotherapy
通过旁观者辅助免疫疗法利用癌症代谢和药物流出
- 批准号:1065508810655088
- 财政年份:2022
- 资助金额:$ 60.5万$ 60.5万
- 项目类别:
Exploiting Cancer Metabolism and Drug Efflux with Bystander-Assisted Immunotherapy
通过旁观者辅助免疫疗法利用癌症代谢和药物流出
- 批准号:1068809710688097
- 财政年份:2022
- 资助金额:$ 60.5万$ 60.5万
- 项目类别:
Exploiting Cancer Metabolism and Drug Efflux with Bystander-Assisted Immunotherapy
通过旁观者辅助免疫疗法利用癌症代谢和药物流出
- 批准号:1022779310227793
- 财政年份:2019
- 资助金额:$ 60.5万$ 60.5万
- 项目类别:
In Vivo Detection And Mechanisms of Regulatory B cell Function in Transplantation
移植中调节性 B 细胞功能的体内检测和机制
- 批准号:1020949610209496
- 财政年份:2015
- 资助金额:$ 60.5万$ 60.5万
- 项目类别:
In Vivo Detection And Mechanisms of Regulatory B cell Function in Transplantation
移植中调节性 B 细胞功能的体内检测和机制
- 批准号:1039302410393024
- 财政年份:2015
- 资助金额:$ 60.5万$ 60.5万
- 项目类别: