Optimizing Acquisition and Reconstruction of Under-sampled MRI for Signal Detection
优化欠采样 MRI 的采集和重建以进行信号检测
基本信息
- 批准号:10730707
- 负责人:
- 金额:$ 43.45万
- 依托单位:
- 依托单位国家:美国
- 项目类别:
- 财政年份:2023
- 资助国家:美国
- 起止时间:2023-09-01 至 2026-08-31
- 项目状态:未结题
- 来源:
- 关键词:
项目摘要
PROJECT SUMMARY
Magnetic resonance imaging (MRI) is a versatile imaging modality that suffers from slow
acquisition times, which is a challenge, for both time sensitive applications and for patient
throughput. Accelerating MRI would benefit patients both by reducing the time they need to
be in the scanner and in reducing the cost of healthcare. This project is part of a larger
scientific effort to accelerate MRI while maintaining the diagnostic quality. Acceleration, even
by a factor of two, would result in a major advance for public health. Two of the current
approaches to accelerate MRI rely on collecting less data (under-sampling) and deep learning
reconstruction. These approaches can lead to images with diagnostic quality using significant
under-sampling but may suffer from artifacts which are hard to characterize and may
sometimes resemble anatomy. Specifically, this project will optimize the performance
of accelerated MRI, including undersampling patterns and deep learning
reconstructions, on detecting and localizing subtle lesions. To carry out this
optimization, we will first develop the methods required for detection of lesions by machine
and human observer models. The human observer models will be validated by
psychophysical studies where humans perform the detection task. In the first aim of this
project, we will apply and develop detection tasks and model observers. We will consider
under-sampled acquisition strategies in MRI including one and two-dimensional subsampling
methods using deep learning reconstructions which enforce data consistency. We will
develop detection tasks for signals in anatomical backgrounds were the signal location is
known and when the observer needs to search for the signal. The human and machine
performance in these tasks will be modeled. In the second aim, we will optimize data
acquisition and neural network reconstruction using signal detection with observer models
and psychophysical experiments. We will also introduce a detectability-based loss function
to neural network reconstructions. There is recent interest in exploring the benefits of low/mid
field MRI which has a trade-off with higher noise. In the third aim, we will evaluate the effect
of field strength on signal detection. We will use data from high field acquisitions from a
publicly available database to model images from lower magnetic fields. Using the detection
of subtle lesions, we will evaluate detection performance with varying field strength. This
research project will help to strengthen the research environment and broaden participation
at Manhattan College by involving students in biomedical research incorporating applied
mathematics, statistics and data science.
项目摘要
磁共振成像(MRI)是一种多功能成像方式,遭受缓慢的影响
对于时间敏感的应用和患者的获取时间,这是一个挑战
吞吐量。加速MRI将通过减少他们需要的时间来使患者受益
在扫描仪中降低医疗保健成本。这个项目是更大的一部分
在保持诊断质量的同时加速MRI的科学努力。加速度,甚至
乘以两倍,将为公共卫生带来重大进步。两个电流
加速MRI的方法依赖于收集更少的数据(不足采样)和深度学习
重建。这些方法可以使用重要的
采样不足,但可能会遭受很难表征的文物的困扰,并且可能
有时类似于解剖学。具体而言,该项目将优化性能
加速的MRI,包括不足采样模式和深度学习
重建,检测和定位微妙的病变。执行此操作
优化,我们将首先开发通过机器检测病变所需的方法
和人类观察者模型。人类观察者模型将通过
人类执行检测任务的心理物理研究。在第一个目的
项目,我们将应用和制定检测任务和模型观察者。我们会考虑的
MRI中采样不足的采集策略,包括一个和二维子采样
使用深度学习重建的方法来实施数据一致性。我们将
在解剖背景下的信号的开发检测任务是信号位置为
已知以及观察者需要搜索信号时。人类和机器
这些任务中的性能将进行建模。在第二个目标中,我们将优化数据
使用信号检测与观察者模型的信号检测的获取和神经网络重建
和心理物理实验。我们还将引入基于可检测性的损失功能
进行神经网络重建。最近有兴趣探索低/中期的好处
现场MRI具有更高噪音的权衡。在第三个目标中,我们将评估效果
信号检测时的野外强度。我们将使用来自高现场获取的数据
可公开可用的数据库,以建模来自较低磁场的图像。使用检测
在微妙的病变中,我们将以不同的场强度评估检测性能。这
研究项目将有助于增强研究环境并扩大参与
在曼哈顿学院,通过让学生参与生物医学研究的应用
数学,统计和数据科学。
项目成果
期刊论文数量(0)
专著数量(0)
科研奖励数量(0)
会议论文数量(0)
专利数量(0)
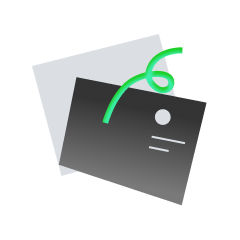
暂无数据
数据更新时间:2024-06-01
相似国自然基金
卫星互联网端到端安全传输模型与安全路由协议研究
- 批准号:62302389
- 批准年份:2023
- 资助金额:30 万元
- 项目类别:青年科学基金项目
新型实用化量子密码协议的高安全等级理论分析
- 批准号:12374473
- 批准年份:2023
- 资助金额:52 万元
- 项目类别:面上项目
中继通信协议下2-D网络化系统的递推状态估计研究
- 批准号:62373103
- 批准年份:2023
- 资助金额:50 万元
- 项目类别:面上项目
云边端架构下联邦学习下行通信压缩算法与协议研究
- 批准号:62372487
- 批准年份:2023
- 资助金额:50.00 万元
- 项目类别:面上项目
隐私比较协议及其应用研究
- 批准号:62372370
- 批准年份:2023
- 资助金额:50.00 万元
- 项目类别:面上项目
相似海外基金
High-Resolution Lymphatic Mapping of the Upper Extremities with MRI
使用 MRI 进行上肢高分辨率淋巴图谱分析
- 批准号:1066371810663718
- 财政年份:2023
- 资助金额:$ 43.45万$ 43.45万
- 项目类别:
Silent Zones of Lung Disease in COPD
慢性阻塞性肺病 (COPD) 肺部疾病的静默区
- 批准号:1059054210590542
- 财政年份:2023
- 资助金额:$ 43.45万$ 43.45万
- 项目类别:
A Novel Therapeutic to Promote Corneal Repair
促进角膜修复的新疗法
- 批准号:1075886310758863
- 财政年份:2023
- 资助金额:$ 43.45万$ 43.45万
- 项目类别:
Role of Sleep Disruption after mTBI as a Driver of Chronic Post-traumatic Headache
mTBI 后睡眠中断是慢性创伤后头痛的驱动因素
- 批准号:1073660210736602
- 财政年份:2023
- 资助金额:$ 43.45万$ 43.45万
- 项目类别:
Machine Learning-based Imaging Biomarkers for Metabolic and Age-related Diseases
基于机器学习的代谢和年龄相关疾病的成像生物标志物
- 批准号:1070735410707354
- 财政年份:2022
- 资助金额:$ 43.45万$ 43.45万
- 项目类别: