A Modular Framework for Data-Driven Neurogenetics to Predict Complex and Multidimensional Autistic Phenotypes
数据驱动神经遗传学预测复杂和多维自闭症表型的模块化框架
基本信息
- 批准号:10826595
- 负责人:
- 金额:$ 46.95万
- 依托单位:
- 依托单位国家:美国
- 项目类别:
- 财政年份:2023
- 资助国家:美国
- 起止时间:2023-06-01 至 2027-05-31
- 项目状态:未结题
- 来源:
- 关键词:
项目摘要
Project Summary/Abstract
Autism Spectrum Disorder (ASD) can be viewed through three complementary lenses: neurologically, it is linked
to distributed changes in brain structure, function, and connectivity; biologically, it is associated with genome-wide
mutations across multiple pathways; and clinically, it manifests as a diverse spectrum of behavioral and cogni-
tive impairments. Despite this richness, treatment options for ASD are based on coarse diagnostics and target
a few specific symptoms, such as social awareness, irritability, and depression. As a result, they have varied,
and often limited, efficacy across patients. Taken together, next-generation therapeutics for ASD will crucially
depend on our ability to bridge its neurological, biological, and clinical viewpoints for personalized intervention.
Imaging-genetics is an emerging field that attempts to link neuroimaging features with genetic variants. How-
ever, most methods focus on a restricted set of biomarkers, and they do not account for clinical phenotype, both
of which provide an incomplete picture of the impacted processes. Our long-term goal is to develop a modu-
lar platform that fuses multimodal neuroimaging and multi-omics data to unravel the complex etiology of ASD.
The overall objective of this proposal is to develop and validate interpretable deep learning models to combine
genome-wide variants with whole-brain structural and functional MRI data. Our innovative strategy is to use
biologically-informed neural network architectures to project each of the modalities to a shared latent space that
is simultaneously predictive of patient-level clinical phenotype. This unique formulation can easily accommodate
missing data modalities, thus maximally utilizing all of the available information. We will devise, implement, vali-
date, and disseminate our model via four specific aims. In Aim 1 we will develop a graph neural network, whose
connections mimic a well-known gene ontology. Hierarchical pooling operations will capture the information flow
through the network, while an attention layer will learn the discriminative biological pathways associated with the
phenotype. In Aim 2 we will develop and integrate a Bayesian feature selection procedure to identify ROI-based
neuroimaging biomarkers and a matrix autoencoder to extract discriminative functional subnetworks from brain
connectivity data. In Aim 3 we will use the fused imaging and genetic architectures to uncover the neural and
biological bases underlying the observed clinical heterogeneity of ASD. Finally, in Aim 4 we will package and dis-
seminate our model as a user-friendly tool for the broader research community. We anticipate the proposed work
will have a transformative impact on ASD research by allowing us to develop refined diagnostic instruments
and on the field of imaging-genetics by providing a new approach for multimodal biomarker discovery.
项目摘要/摘要
自闭症谱系障碍(ASD)可以通过三个完整的镜头观看:神经学上,它是链接的
大脑结构,功能和连通性的分布变化;在生物学上,它与全基因组有关
跨多个途径的突变;在临床上,它表现为行为和认知的不同范围
损害。尽管如此,ASD的治疗选择仍基于粗糙的诊断和目标
一些特定的符号,例如社会意识,易怒和沮丧。结果,它们变化了,
并且通常有限,患者的效率。两者合计,对ASD的下一代疗法将至关重要
取决于我们桥接其神经,生物学和临床观点以进行个性化干预的能力。
成像遗传学是一种新兴领域,试图将神经影像特征与遗传变异联系起来。如何-
有史以来,大多数方法都集中在一组受限制的生物标志物上,并且它们不考虑临床表型,两者都
其中提供了不完整的影响过程。我们的长期目标是开发一个模型
融合多模式神经影像学和多媒体数据的LAR平台以揭示ASD的复杂病因。
该建议的总体目的是开发和验证可解释的深度学习模型以结合
具有全脑结构和功能性MRI数据的全基因组变体。我们的创新策略是使用
生物知识的神经网络体系结构将每种模式都投射到共享的潜在空间
仅预测患者级临床表型。这个独特的公式可以轻松容纳
缺少数据模式,因此最大程度地利用了所有可用信息。我们将设计,实施,vali-
日期,并通过四个特定目标传播我们的模型。在AIM 1中,我们将开发一个图形神经网络,谁
连接模仿众所周知的基因本体。分层池操作将捕获信息流
通过网络,注意力层将学习与
表型。在AIM 2中,我们将开发和集成一个贝叶斯特征选择程序,以识别基于ROI的基于ROI
神经成像生物标志物和矩阵自动编码器从脑中提取歧视功能子网
连接数据。在AIM 3中,我们将使用融合的成像和遗传体系结构来揭示神经和
观察到的ASD临床异质性的基础生物基础。最后,在AIM 4中,我们将包装并脱颖而出
我们的模型将我们的模型作为更广泛的研究社区的用户友好工具。我们期待拟议的工作
通过允许我们开发重定的诊断工具,将对ASD研究产生变革性的影响
并通过为多模式生物标志物发现的新方法提供成像基因的领域。
项目成果
期刊论文数量(0)
专著数量(0)
科研奖励数量(0)
会议论文数量(0)
专利数量(0)
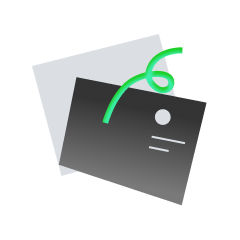
暂无数据
数据更新时间:2024-06-01
Archana Venkatara...的其他基金
Automated Presurgical Language Mapping via Deep Learning for Multimodal Brain Connectivity
通过深度学习进行自动术前语言映射以实现多模式大脑连接
- 批准号:1028618110286181
- 财政年份:2021
- 资助金额:$ 46.95万$ 46.95万
- 项目类别:
相似国自然基金
分布式非凸非光滑优化问题的凸松弛及高低阶加速算法研究
- 批准号:12371308
- 批准年份:2023
- 资助金额:43.5 万元
- 项目类别:面上项目
资源受限下集成学习算法设计与硬件实现研究
- 批准号:62372198
- 批准年份:2023
- 资助金额:50 万元
- 项目类别:面上项目
基于物理信息神经网络的电磁场快速算法研究
- 批准号:52377005
- 批准年份:2023
- 资助金额:52 万元
- 项目类别:面上项目
考虑桩-土-水耦合效应的饱和砂土变形与流动问题的SPH模型与高效算法研究
- 批准号:12302257
- 批准年份:2023
- 资助金额:30 万元
- 项目类别:青年科学基金项目
面向高维不平衡数据的分类集成算法研究
- 批准号:62306119
- 批准年份:2023
- 资助金额:30 万元
- 项目类别:青年科学基金项目
相似海外基金
Previvors Recharge: A Resilience Program for Cancer Previvors
癌症预防者恢复活力计划:癌症预防者恢复力计划
- 批准号:1069896510698965
- 财政年份:2023
- 资助金额:$ 46.95万$ 46.95万
- 项目类别:
Neuronal mechanisms of model-based learning
基于模型的学习的神经机制
- 批准号:1072226110722261
- 财政年份:2023
- 资助金额:$ 46.95万$ 46.95万
- 项目类别:
Move and Snooze: Adding insomnia treatment to an exercise program to improve pain outcomes in older adults with knee osteoarthritis
活动和小睡:在锻炼计划中添加失眠治疗,以改善患有膝骨关节炎的老年人的疼痛结果
- 批准号:1079705610797056
- 财政年份:2023
- 资助金额:$ 46.95万$ 46.95万
- 项目类别:
In vivo Evaluation of Lymph Nodes Using Quantitative Ultrasound
使用定量超声对淋巴结进行体内评估
- 批准号:1073715210737152
- 财政年份:2023
- 资助金额:$ 46.95万$ 46.95万
- 项目类别:
miR-137 Regulation of Intrinsic Excitability
miR-137 内在兴奋性的调节
- 批准号:1071957910719579
- 财政年份:2023
- 资助金额:$ 46.95万$ 46.95万
- 项目类别: