Quantitative Image Analysis Techniques for Optic Nerve Disease
视神经疾病的定量图像分析技术
基本信息
- 批准号:8620598
- 负责人:
- 金额:$ 22.51万
- 依托单位:
- 依托单位国家:美国
- 项目类别:
- 财政年份:2013
- 资助国家:美国
- 起止时间:2013-12-01 至 2015-11-30
- 项目状态:已结题
- 来源:
- 关键词:
项目摘要
PROJECT SUMMARY/ABSTRACT
Disorders of the optic nerve (ON) account for a significant percentage of the 20 most impactful ophthalmological
conditions. Collectively, diseases of the ON are the number one cause of irreversible blindness worldwide, and present
serious public health concerns in the U.S. Consider, for example, that glaucoma impacts more than three million Ameri-
cans and costs the U.S. economy almost $3 billion per year. Optic neuritis (i.e., inflammatory demyelination of the ON) is
the initial symptom in ~25% of all multiple sclerosis (MS) cases (which impacts over 400 thousand Americans and intro-
duces societal health care costs of nearly $30 billion per year). Nearly two thirds of MS patients will experience episodes
of optic neuritis in their lifetimes, and 40-60% of patients have visual defects localized to the ON. These disorders irre-
versibly damage the ON. Even so, damage to axons in the ON is progressive, defined by a window of opportunity for
treatment between loss of function and actual degeneration. The potential for recovery exists because there are
treatments that can help prevent progression if administered during this window of opportunity. Yet, we do not have
effective means to assess who is in the window and who will benefit from treatment.
We propose to translate computational imaging methods from the neuroimaging community to provide ro-
bust, quantitative tools for assessing the optic nerve (ON) on clinical and research imaging sequences. These efforts
will improve prognostic accuracy, lead to better understanding of patient responses, and enhance targeted interven-
tions. The technical hypothesis of this work is that quantitative image processing can robustly and accurately segment,
register, and fuse ON data from modern MRI and CT clinical sequences. The central hypothesis of this proposal is that
qualitative ON phenotypes on longitudinal clinical imaging will differentiate individuals who respond to treatment versus
those who do not.
The overall goal of this research is to provide a foundation for image analysis of the ON and its relationships
with pathological disorders. We will build upon recent advances in robust medical image computing to segment the ON
in clinical CT and MRI acquisitions, develop registration procedures to establish intra- and inter-subject correspondence,
and bring together information from the multi-modal battery of imaging studies that are typically used in clinical care
(aim 1). With these new methods, we will address the exploratory hypothesis that quantitative use of clinical imaging
data can increase prognostic accuracy (aim 2). We note that aim 2 is particularly exploratory and in line with the high-
risk/high-reward aspect of this mechanism; many studies have shown that baseline imaging does not conclusively pre-
dict long term outcome or treatment response. We hypothesize that this may be because early findings are related to
edema and inflammation rather than cellular damage per se. Once this exploratory phase is complete, we will pursue
promising prognostic biomarkers using more detailed condition staging criteria and including more than two longitudinal
time points in the analysis. Ultimately, these efforts will improve assessment ON disease and, in turn, patient care.
项目摘要/摘要
视神经(ON)的疾病占20种最有影响力的眼科的很大比例
状况。总体而言,ON的疾病是全球不可逆失明的第一起,并且存在
例如,美国对公共卫生的严重关注考虑,例如,青光眼会影响超过300万美洲
罐头和每年损失美国经济近30亿美元。视神经炎(即,ON的炎性脱髓鞘)为
所有多发性硬化症(MS)病例中约有25%的最初症状(这会影响超过40万的美国人和介入
DUCES社会医疗保健每年近300亿美元)。将近三分之二的MS患者会经历发作
其一生中的视神经炎和40-60%的患者的视觉缺陷局部位于ON。这些疾病无关
可用的损坏。即便如此,对轴突的损坏是渐进的,由一个机会窗口定义
功能丧失和实际变性之间的处理。存在恢复的潜力,因为有
如果在此机会之窗中进行管理,可以帮助防止进步的治疗方法。但是,我们没有
有效评估谁在窗户中的人,谁将从治疗中受益。
我们建议从神经影像社区转换计算成像方法,以提供RO-
胸围,用于评估临床和研究成像序列上视神经(ON)的定量工具。这些努力
将提高预后准确性,更好地了解患者反应,并提高目标介入
tions。这项工作的技术假设是,定量图像处理可以稳健,准确地分割,
注册,并融合来自现代MRI和CT临床序列的数据。该提议的核心假设是
关于纵向临床成像的表型的定性将使对治疗的反应与
那些没有的人。
这项研究的总体目标是为ON及其关系的图像分析提供基础
患有病理障碍。我们将基于最新的强大医学图像计算的进步,以分割ON
在临床CT和MRI获取中,制定了建立内部和受试者间对应的注册程序,
并将通常用于临床护理中通常使用的多模式成像研究中的信息聚集在一起
(目标1)。使用这些新方法,我们将解决探索性假设,即临床成像的定量使用
数据可以提高预后精度(AIM 2)。我们注意到AIM 2特别探索性,并且与高
这种机制的风险/高回报;许多研究表明,基线成像并未最终预先
将长期结局或治疗反应命令。我们假设这可能是因为早期发现与
水肿和炎症,而不是细胞损伤本身。一旦这个探索阶段完成,我们将追求
使用更详细的条件分期标准,有希望的预后生物标志物,包括两个以上的纵向
分析中的时间点。最终,这些努力将改善对疾病以及患者护理的评估。
项目成果
期刊论文数量(0)
专著数量(0)
科研奖励数量(0)
会议论文数量(0)
专利数量(0)
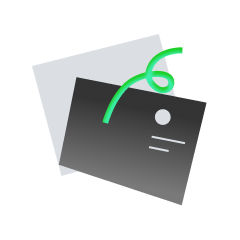
暂无数据
数据更新时间:2024-06-01
Bennett A. Landman其他文献
Nucleus subtype classification using inter-modality learning
使用跨模态学习进行细胞核亚型分类
- DOI:
- 发表时间:20242024
- 期刊:
- 影响因子:0
- 作者:Lucas W. Remedios;Shunxing Bao;Samuel W. Remedios;Ho Hin Lee;L. Cai;Thomas Z. Li;Ruining Deng;Can Cui;Jia Li;Qi Liu;Ken S. Lau;Joseph T. Roland;M. K. Washington;Lori A. Coburn;Keith T. Wilson;Yuankai Huo;Bennett A. LandmanLucas W. Remedios;Shunxing Bao;Samuel W. Remedios;Ho Hin Lee;L. Cai;Thomas Z. Li;Ruining Deng;Can Cui;Jia Li;Qi Liu;Ken S. Lau;Joseph T. Roland;M. K. Washington;Lori A. Coburn;Keith T. Wilson;Yuankai Huo;Bennett A. Landman
- 通讯作者:Bennett A. LandmanBennett A. Landman
RAISE - Radiology AI Safety, an End-to-end lifecycle approach
RAISE - 放射学人工智能安全,一种端到端生命周期方法
- DOI:10.48550/arxiv.2311.1457010.48550/arxiv.2311.14570
- 发表时间:20232023
- 期刊:
- 影响因子:0
- 作者:M. Cardoso;Julia Moosbauer;Tessa S. Cook;B. S. Erdal;Brad W. Genereaux;Vikash Gupta;Bennett A. Landman;Tiarna Lee;P. Nachev;Elanchezhian Somasundaram;Ronald M. Summers;Khaled Younis;S. Ourselin;Franz MJ PfisterM. Cardoso;Julia Moosbauer;Tessa S. Cook;B. S. Erdal;Brad W. Genereaux;Vikash Gupta;Bennett A. Landman;Tiarna Lee;P. Nachev;Elanchezhian Somasundaram;Ronald M. Summers;Khaled Younis;S. Ourselin;Franz MJ Pfister
- 通讯作者:Franz MJ PfisterFranz MJ Pfister
DeepN4: Learning N4ITK Bias Field Correction for T1-weighted Images
DeepN4:学习 T1 加权图像的 N4ITK 偏置场校正
- DOI:10.1007/s12021-024-09655-910.1007/s12021-024-09655-9
- 发表时间:20242024
- 期刊:
- 影响因子:3
- 作者:Praitayini Kanakaraj;Tianyuan Yao;L. Cai;Ho Hin Lee;Nancy R. Newlin;Michael E. Kim;Chenyu Gao;Kimberly R. Pechman;D. Archer;Timothy Hohman;Angela L. Jefferson;L. Beason;Susan M. Resnick;E. Garyfallidis;Adam Anderson;K. Schilling;Bennett A. Landman;Daniel MoyerPraitayini Kanakaraj;Tianyuan Yao;L. Cai;Ho Hin Lee;Nancy R. Newlin;Michael E. Kim;Chenyu Gao;Kimberly R. Pechman;D. Archer;Timothy Hohman;Angela L. Jefferson;L. Beason;Susan M. Resnick;E. Garyfallidis;Adam Anderson;K. Schilling;Bennett A. Landman;Daniel Moyer
- 通讯作者:Daniel MoyerDaniel Moyer
Enhancing Single-Slice Segmentation with 3D-to-2D Unpaired Scan Distillation
通过 3D 到 2D 不成对扫描蒸馏增强单切片分割
- DOI:
- 发表时间:20242024
- 期刊:
- 影响因子:0
- 作者:Xin Yu;Qi Yang;Han Liu;Ho Hin Lee;Yucheng Tang;Lucas W. Remedios;Michael Kim;Shunxing Bao;Ann Xenobia Moore;Luigi Ferrucci;Bennett A. LandmanXin Yu;Qi Yang;Han Liu;Ho Hin Lee;Yucheng Tang;Lucas W. Remedios;Michael Kim;Shunxing Bao;Ann Xenobia Moore;Luigi Ferrucci;Bennett A. Landman
- 通讯作者:Bennett A. LandmanBennett A. Landman
Broadband nanosensing using heterodyne interferometry
- DOI:
- 发表时间:20022002
- 期刊:
- 影响因子:0
- 作者:Bennett A. LandmanBennett A. Landman
- 通讯作者:Bennett A. LandmanBennett A. Landman
共 9 条
- 1
- 2
Bennett A. Landman的其他基金
Novel Integrative Approach for the Early Detection of Lung Cancer using Repeated Measures
使用重复测量早期检测肺癌的新综合方法
- 批准号:1032271210322712
- 财政年份:2021
- 资助金额:$ 22.51万$ 22.51万
- 项目类别:
Novel Integrative Approach for the Early Detection of Lung Cancer using Repeated Measures
使用重复测量早期检测肺癌的新综合方法
- 批准号:1059657010596570
- 财政年份:2021
- 资助金额:$ 22.51万$ 22.51万
- 项目类别:
Controlling Quality and Capturing Uncertainty in Advanced Diffusion Weighted MRI
控制质量并捕捉高级扩散加权 MRI 的不确定性
- 批准号:1049090410490904
- 财政年份:2015
- 资助金额:$ 22.51万$ 22.51万
- 项目类别:
Controlling Quality and Capturing Uncertainty in Advanced Diffusion Weighted MRI
控制质量并捕捉高级扩散加权 MRI 的不确定性
- 批准号:1031667110316671
- 财政年份:2015
- 资助金额:$ 22.51万$ 22.51万
- 项目类别:
Controlling Quality and Capturing Uncertainty in Advanced Diffusion Weighted MRI
控制质量并捕捉高级扩散加权 MRI 的不确定性
- 批准号:1068330610683306
- 财政年份:2015
- 资助金额:$ 22.51万$ 22.51万
- 项目类别:
Controlling Quality and Capturing Uncertainty in Advanced Diffusion Weighted MRI
控制质量并捕捉高级扩散加权 MRI 的不确定性
- 批准号:91469519146951
- 财政年份:2015
- 资助金额:$ 22.51万$ 22.51万
- 项目类别:
Resource Development for the Java Image Science Toolkit
Java 图像科学工具包的资源开发
- 批准号:80137018013701
- 财政年份:2010
- 资助金额:$ 22.51万$ 22.51万
- 项目类别:
相似国自然基金
阿魏酸基天然抗氧化抗炎纳米药物用于急性肾损伤诊疗一体化研究
- 批准号:82302281
- 批准年份:2023
- 资助金额:30 万元
- 项目类别:青年科学基金项目
SGO2/MAD2互作调控肝祖细胞的细胞周期再进入影响急性肝衰竭肝再生的机制研究
- 批准号:82300697
- 批准年份:2023
- 资助金额:30 万元
- 项目类别:青年科学基金项目
基于hemin-MOFs的急性心肌梗塞标志物负背景光电化学-比色双模分析
- 批准号:22304039
- 批准年份:2023
- 资助金额:30 万元
- 项目类别:青年科学基金项目
RNA甲基转移酶NSUN2介导SCD1 mRNA m5C修饰调控急性髓系白血病细胞铁死亡的机制研究
- 批准号:82300173
- 批准年份:2023
- 资助金额:30 万元
- 项目类别:青年科学基金项目
基于IRF5/MYD88信号通路调控巨噬细胞M1极化探讨针刀刺营治疗急性扁桃体炎的机制研究
- 批准号:82360957
- 批准年份:2023
- 资助金额:30 万元
- 项目类别:地区科学基金项目
相似海外基金
Climate Change Effects on Pregnancy via a Traditional Food
气候变化通过传统食物对怀孕的影响
- 批准号:1082220210822202
- 财政年份:2024
- 资助金额:$ 22.51万$ 22.51万
- 项目类别:
Determining medications associated with drug-induced pancreatic injury through novel pharmacoepidemiology techniques that assess causation
通过评估因果关系的新型药物流行病学技术确定与药物引起的胰腺损伤相关的药物
- 批准号:1063824710638247
- 财政年份:2023
- 资助金额:$ 22.51万$ 22.51万
- 项目类别:
TIER-PALLIATIVE CARE: A population-based care delivery model to match evolving patient needs and palliative care services for community-based patients with heart failure or cancer
分级姑息治疗:基于人群的护理提供模式,以满足不断变化的患者需求,并为社区心力衰竭或癌症患者提供姑息治疗服务
- 批准号:1088099410880994
- 财政年份:2023
- 资助金额:$ 22.51万$ 22.51万
- 项目类别:
Identification of gene variants mediating the behavioral and physiological response to THC
鉴定介导 THC 行为和生理反应的基因变异
- 批准号:1066080810660808
- 财政年份:2023
- 资助金额:$ 22.51万$ 22.51万
- 项目类别:
Kinetochore Assembly and Regulation
着丝粒组装和调控
- 批准号:1071720210717202
- 财政年份:2023
- 资助金额:$ 22.51万$ 22.51万
- 项目类别: