Design and Inference for Hybrid Ecological Studies
混合生态研究的设计和推理
基本信息
- 批准号:7434489
- 负责人:
- 金额:$ 18.36万
- 依托单位:
- 依托单位国家:美国
- 项目类别:
- 财政年份:2007
- 资助国家:美国
- 起止时间:2007-06-01 至 2010-05-31
- 项目状态:已结题
- 来源:
- 关键词:
项目摘要
DESCRIPTION (provided by applicant): Ecological studies may be defined examining associations at the group level. They are appealing in that they make use of routinely available data, and also offer the potential of high power due to large populations and broad exposure contrasts. However, they are also susceptible to a range of biases with respect to individual-level associations, collectively termed ecological bias, and may lead to the ecological fallacy. In epidemiology, the fundamental difficulty is the inability of ecological data to characterize within-group variability in exposures and confounders. This results in an inability to control for confounding, and general non-identifiability of the individual-level model. The only solution to the ecological inference problem is to supplement ecological data with individual-level samples; in this proposal we describe and develop a variety of hybrid studies that pursue this solution. Specifically, we develop a hybrid design in which a case-control study is embedded within an ecological study. The intuitive appeal is that the individual-level data provide the basis for the control of bias, while the ecological data provide efficiency gains. In addition, we extend current methods, including the aggregate data design and two-phase method, to the ecological setting. This will be based on the development of Bayesian methods for these designs, which have not been explored. Further, we will compare performance of the various methods in a variety of data/sampling scenarios. A key research question is whether the group-level data provide useful information for the collection of individuals. We will explore optimal study design in terms of how many individuals to sample and from which groups. The methods are illustrated with two cancer data sets and one influenza data set.
描述(由申请人提供):可以定义生态学研究,以检查小组级别的关联。他们很有吸引力的是,他们利用了常规可用的数据,并且由于人口众多和广泛的暴露对比,也提供了高力量的潜力。但是,它们也容易遭受各种偏见,就个人级别的关联而言,共同称为生态偏见,并可能导致生态谬误。在流行病学中,基本困难是生态数据无法表征暴露和混杂因素的组内变异性。这导致无法控制个人级模型的混淆和一般的非识别性。生态推断问题的唯一解决方案是用个体水平的样本补充生态数据。在此提案中,我们描述并开发了采用该解决方案的各种混合研究。具体而言,我们开发了一种混合设计,其中病例对照研究嵌入了生态研究中。直观的吸引力是,个体级别的数据为控制偏见提供了基础,而生态数据则提供了效率提高。此外,我们将电流方法(包括总数据设计和两阶方法)扩展到生态环境。这将基于尚未探讨这些设计的贝叶斯方法的开发。此外,我们将在各种数据/采样方案中比较各种方法的性能。一个关键的研究问题是,小组级数据是否为个人收集提供了有用的信息。我们将根据要采样的个人以及哪些组来探讨最佳研究设计。用两个癌症数据集和一个流感数据集说明了这些方法。
项目成果
期刊论文数量(0)
专著数量(0)
科研奖励数量(0)
会议论文数量(0)
专利数量(0)
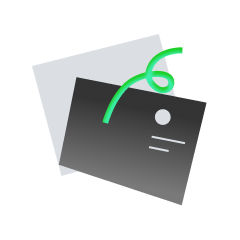
暂无数据
数据更新时间:2024-06-01
SEBASTIEN HANEUSE的其他基金
Robust methods for missing data in electronic health records-based studies
基于电子健康记录的研究中缺失数据的稳健方法
- 批准号:1018187310181873
- 财政年份:2021
- 资助金额:$ 18.36万$ 18.36万
- 项目类别:
Robust methods for missing data in electronic health records-based studies
基于电子健康记录的研究中缺失数据的稳健方法
- 批准号:1039038210390382
- 财政年份:2021
- 资助金额:$ 18.36万$ 18.36万
- 项目类别:
Robust methods for missing data in electronic health records-based studies
基于电子健康记录的研究中缺失数据的稳健方法
- 批准号:1058913310589133
- 财政年份:2021
- 资助金额:$ 18.36万$ 18.36万
- 项目类别:
Clustered semi-competing risks analysis in quality of end-of-life care studies
临终关怀研究质量中的聚类半竞争风险分析
- 批准号:86122758612275
- 财政年份:2014
- 资助金额:$ 18.36万$ 18.36万
- 项目类别:
Clustered semi-competing risks analysis in quality of end-of-life care studies
临终关怀研究质量中的聚类半竞争风险分析
- 批准号:88058348805834
- 财政年份:2014
- 资助金额:$ 18.36万$ 18.36万
- 项目类别:
Design Considerations for Two-Phase Studies
两阶段研究的设计注意事项
- 批准号:77794977779497
- 财政年份:2009
- 资助金额:$ 18.36万$ 18.36万
- 项目类别:
Design Considerations for Two-Phase Studies
两阶段研究的设计注意事项
- 批准号:81933518193351
- 财政年份:2009
- 资助金额:$ 18.36万$ 18.36万
- 项目类别:
Design Considerations for Two-Phase Studies
两阶段研究的设计注意事项
- 批准号:76586407658640
- 财政年份:2009
- 资助金额:$ 18.36万$ 18.36万
- 项目类别:
Design and Inference for Hybrid Ecological Studies
混合生态研究的设计和推理
- 批准号:76263107626310
- 财政年份:2007
- 资助金额:$ 18.36万$ 18.36万
- 项目类别:
Design and Inference for Hybrid Ecological Studies
混合生态研究的设计和推理
- 批准号:71853667185366
- 财政年份:2007
- 资助金额:$ 18.36万$ 18.36万
- 项目类别:
相似国自然基金
无界区域中非局部Klein-Gordon-Schrödinger方程的保结构算法研究
- 批准号:12301508
- 批准年份:2023
- 资助金额:30 万元
- 项目类别:青年科学基金项目
感兴趣区域驱动的主动式采样CT成像算法研究
- 批准号:62301532
- 批准年份:2023
- 资助金额:30.00 万元
- 项目类别:青年科学基金项目
面向多区域单元化生产线协同调度问题的自动算法设计研究
- 批准号:62303204
- 批准年份:2023
- 资助金额:30 万元
- 项目类别:青年科学基金项目
基于深度强化学习的约束多目标群智算法及多区域热电调度应用
- 批准号:62303197
- 批准年份:2023
- 资助金额:30 万元
- 项目类别:青年科学基金项目
面向二氧化碳封存的高可扩展时空并行区域分解算法及其大规模应用
- 批准号:12371366
- 批准年份:2023
- 资助金额:43.5 万元
- 项目类别:面上项目
相似海外基金
Transcranial Ultrasound Algorithms and Device for Rapid Stroke Determination by Paramedics
用于医护人员快速确定中风的经颅超声算法和设备
- 批准号:1073072210730722
- 财政年份:2023
- 资助金额:$ 18.36万$ 18.36万
- 项目类别:
Bayesian Statistical Learning for Robust and Generalizable Causal Inferences in Alzheimer Disease and Related Disorders Research
贝叶斯统计学习在阿尔茨海默病和相关疾病研究中进行稳健且可推广的因果推论
- 批准号:1059091310590913
- 财政年份:2023
- 资助金额:$ 18.36万$ 18.36万
- 项目类别:
Shape-based personalized AT(N) imaging markers of Alzheimer's disease
基于形状的个性化阿尔茨海默病 AT(N) 成像标记
- 批准号:1066790310667903
- 财政年份:2023
- 资助金额:$ 18.36万$ 18.36万
- 项目类别:
Exploiting translation elongation for improved biologics manufacturing
利用平移伸长来改进生物制品的制造
- 批准号:1076092710760927
- 财政年份:2023
- 资助金额:$ 18.36万$ 18.36万
- 项目类别:
Matched Design with Sensitivity Analysis for Observational Survival Data in Cardiovascular Patient Management using EMR Data
使用 EMR 数据对心血管患者管理中的观察性生存数据进行匹配设计和敏感性分析
- 批准号:1073117210731172
- 财政年份:2023
- 资助金额:$ 18.36万$ 18.36万
- 项目类别: