Derivation of a Clinical Prediction Rule for Pediatric Abusive Fractures
儿童虐待性骨折临床预测规则的推导
基本信息
- 批准号:10331949
- 负责人:
- 金额:$ 40.7万
- 依托单位:
- 依托单位国家:美国
- 项目类别:
- 财政年份:2022
- 资助国家:美国
- 起止时间:2022-04-01 至 2027-01-31
- 项目状态:未结题
- 来源:
- 关键词:
项目摘要
PROJECT SUMMARY
Child abuse and neglect represent one of the most serious pediatric public health crises, affecting nearly
1 in 7 children. Fractures are the 2nd most common abusive injury after skin and soft tissue injuries and there is
much overlap between the types of fractures caused by abuse and unintentional mechanisms. The diagnosis of
child abuse is complex and necessitates an accurate understanding of typical pediatric injury patterns within the
context of history, mechanism, socio-demographics, and developmental capabilities. Many studies evaluating
the relationship between fractures and abuse focused on specific fracture types, were restricted to children with
a pre-defined abusive injury or included only admitted patients, and/or relatively small cohorts, thus limiting
conclusions and raising concerns of spectrum bias. Additionally, prior literature has shown implicit and explicit
biases related to socio-demographic factors in the identification and evaluation of abuse, likely resulting in over-
and underdiagnosis of abuse in some populations. Furthermore, over 75% of children seeking ED care are seen
in general ED’s by providers without specialized training in child development and abuse, and up to 1 in 5 children
with abusive fractures may be missed in a general ED setting. Despite the frequency of abusive fractures and
the potential limitations and biases in making the diagnosis, there are no validated clinical decision rules (CDRs)
to assist clinicians in the real-time identification of children with fracture presentations associated with abuse.
Our long-term goal is to develop a validated CDR that can be used by clinicians evaluating injured children to
assist in the identification of abusive fracture presentations. Our primary objective is to utilize gradient boosted
decision tree ensembles to develop a CDR that will identify fracture presentations highly concerning for abuse
among patients ≤5 years presenting for emergency department (ED) care. An institutional child protection
database that includes outcomes of thorough expert child abuse investigations will be used as a reference
standard. The study objectives will be accomplished by 1) analyzing structured variables in the electronic health
record (EHR) of patients with fractures evaluated in the Hasbro Children’s Hospital (HCH) ED and HCH Child
Protection Program (CPP) using descriptive statistics, 2) applying natural language processing (NLP) techniques
to extract data from clinical narratives and radiology reports to generate text-derived variables, 3) employing
machine learning (ML) techniques to identify predictor variables to derive and iteratively refine a CDR, and 4)
validating this CDR with a different HCH cohort of patients. The expected immediate outcome of this project is
the development of a refined CDR to identify fracture presentations that are highly concerning for abuse among
children ≤5 years old. This will inform the design of a prospective multi-center follow-up study for broad validation
of CDR’s ability to identify high risk patient presentations, improve real-time clinical detection of potentially
abusive injuries, and decrease disparities in clinical decision making.
项目摘要
虐待和忽视儿童是最严重的小儿公共卫生危机之一,几乎影响
七分之一的孩子。骨折是皮肤和软组织损伤后第二大最常见的虐待伤害,并且有
由滥用和无意的机制引起的骨折类型之间的重叠。诊断
虐待儿童是复杂的,必要的准确理解对典型的小儿损伤模式
历史,机制,社会人口统计学和发展能力的背景。许多研究评估
裂缝与虐待之间的关系集中在特定的骨折类型上,仅限于
预定义的虐待伤害或仅包括入院的患者和/或相对较小的同类群体,从而限制
结论和提高对频谱偏见的关注。此外,先前的文献显示了隐式和明确的
与社会人口统计学因素有关的识别和评估滥用的偏见,可能导致过度
和某些人群中虐待的诊断不足。此外,看到超过75%的寻求ED护理儿童
总体而言,Ed的提供者没有提供儿童发育和虐待方面的专门培训,最多只有1个儿童
在一般的环境中,可能会错过虐待裂缝。尽管发生了虐待骨折的频率和
进行诊断的潜在局限性和偏见,没有经过验证的临床决策规则(CDRS)
帮助临床医生实时识别与虐待相关的骨折表现的儿童。
我们的长期目标是开发一个经过验证的CDR,可以通过评估受伤儿童的临床医生使用该CDR
协助识别虐待骨折表现。我们的主要目标是利用梯度提升
决策树的合奏开发一个CDR,该CDR可以识别极大关注虐待的断裂表现
在急诊室(ED)护理≤5岁的患者中。机构儿童保护
包括全面专家虐待儿童调查结果的数据库将用作参考
标准。研究目标将通过1)在电子健康中分析的结构化变量来实现
在孩之宝儿童医院(HCH)ED和HCH儿童中评估的骨折患者的记录(EHR)
使用描述性统计数据的保护计划(CPP),2)应用自然语言处理(NLP)技术
从临床叙述和放射学报告中提取数据以生成文本衍生变量,3)采用
机器学习(ML)技术以识别预测变量以得出并迭代地完善CDR,4)
用不同的HCH患者验证此CDR。该项目的预期直接结果是
开发精致的CDR,以确定非常关注虐待的裂缝表现
儿童≤5岁。这将为一项预期的多中心后续研究提供信息,以进行广泛验证
CDR鉴定高风险患者表现的能力,改善实时临床检测
虐待伤害,并减少临床决策的差异。
项目成果
期刊论文数量(0)
专著数量(0)
科研奖励数量(0)
会议论文数量(0)
专利数量(0)
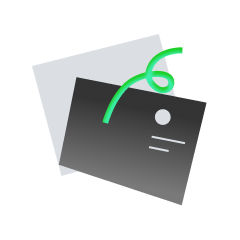
暂无数据
数据更新时间:2024-06-01
Stephanie Ruest的其他基金
Derivation of a Clinical Prediction Rule for Pediatric Abusive Fractures
儿童虐待性骨折临床预测规则的推导
- 批准号:1059808210598082
- 财政年份:2022
- 资助金额:$ 40.7万$ 40.7万
- 项目类别:
相似海外基金
Derivation of a Clinical Prediction Rule for Pediatric Abusive Fractures
儿童虐待性骨折临床预测规则的推导
- 批准号:1059808210598082
- 财政年份:2022
- 资助金额:$ 40.7万$ 40.7万
- 项目类别:
Paid Family Leave and Prevention of Respiratory Tract Infections in Young Infants
带薪家事假与幼儿呼吸道感染的预防
- 批准号:1070990810709908
- 财政年份:2022
- 资助金额:$ 40.7万$ 40.7万
- 项目类别:
Paid Family Leave and Prevention of Respiratory Tract Infections in Young Infants
带薪家事假与幼儿呼吸道感染的预防
- 批准号:1059290110592901
- 财政年份:2022
- 资助金额:$ 40.7万$ 40.7万
- 项目类别:
Infant specific-IgE, rhinovirus-C bronchiolitis, and incident asthma in MARC-35
MARC-35 中的婴儿特异性 IgE、鼻病毒 C 细支气管炎和哮喘事件
- 批准号:89748108974810
- 财政年份:2014
- 资助金额:$ 40.7万$ 40.7万
- 项目类别:
Entrainment-based mechanical ventilation
基于夹带的机械通气
- 批准号:78140847814084
- 财政年份:2009
- 资助金额:$ 40.7万$ 40.7万
- 项目类别: