Leveraging Computer Vision to Augment Suicide Risk Prediction
利用计算机视觉增强自杀风险预测
基本信息
- 批准号:10285809
- 负责人:
- 金额:$ 26.55万
- 依托单位:
- 依托单位国家:美国
- 项目类别:
- 财政年份:2021
- 资助国家:美国
- 起止时间:2021-09-01 至 2023-08-31
- 项目状态:已结题
- 来源:
- 关键词:
项目摘要
Abstract
Self-injurious behaviors occur at alarmingly high rates among adolescents, with suicide ranking as the second
leading cause of death among those ages 15-24. A history of prior self-injury, including both nonsuicidal self-
injury and suicidal self-injury (e.g., suicide attempts), has been consistently found to be the strongest predictor
of future suicidal behavior, with evidence suggesting that the more severe such behaviors are, the greater the
risk for future self-injury. Importantly, however, our current means of assessing severity of prior self-injury is
almost entirely reliant on self-report, despite the fact that self-injury frequently leaves tangible physical
markings. Although applications of machine learning in medical image analysis are growing exponentially,
none have attempted to augment suicide risk detection through automated analysis of self-directed tissue
damage. Leveraging computer vision to automatically assess images of tissue damage has the potential to
obviate complete reliance on subjective patient report of self-injury severity characteristics. Thus, the objective
of this proposal is to utilize computer vision techniques to automate the assessment of hypothesized self-injury
visual severity indicators, learn new visual severity indicators, and determine the utility of these visual signals
in predicting prospective suicide attempt risk. Community adolescents ages 16 to 18 years old will be recruited
on Facebook and Instagram if they have currently visible physical marking(s) secondary to self-injury.
Participants will securely upload images of markings secondary to intentional self-injury. A subset of
participants will be followed longitudinally for three months to assess prospective suicide attempts. We will
employ deep convolutional neural networks, a class of artificial neural networks, to develop algorithms to
detect severity indices of self-injury and to examine their accuracy in predicting short-term prospective suicide
risk. We will assess the generalizability of a subset of algorithms by applying them to a separate clinical
sample of psychiatrically hospitalized adolescents ages 16 to 18 years old. This proof-of-concept study will set
the stage to determine the feasibility of pursuing our long-term goal of integrating this technology into
psychiatric care entry-points (e.g., emergency departments, inpatient units) to assess whether this technology
can augment current suicide risk assessment models and in turn, serve as a clinical decision-support tool to
help clinicians assess suicide risk. This research is significant in that it aligns with the NIMH/National Action
Alliance for Suicide Prevention’s Prioritized Research Agenda for Suicide Prevention’s Aspirational Goal 2 of
determining suicide risk in diverse populations and settings using feasible and effective assessment
approaches, and Goal 3 of finding novel ways to assess for imminent suicide risk, given that our target
prediction period is three months.
抽象的
自我伤害的行为在青少年中以惊人的速度出现,自杀列为第二个
15-24岁的主要死亡原因。先前的自我伤害的历史,包括非自杀的自我
伤害和自杀自我伤害(例如自杀企图)一直被认为是强大的预测因子
未来自杀行为的证据表明,这种行为越严重,
未来自我伤害的风险。但是,重要的是,我们当前评估先前自我伤害严重程度的手段是
几乎完全依赖自我报告,自我伤害经常留下有形的身体
标记。尽管机器学习在医学图像分析中的应用正在成倍增长,但
没有人试图通过自动分析自定向组织来增强自杀风险检测
损害。利用计算机视觉自动评估组织损伤的图像有可能
消除对患者自我伤害严重程度特征的受试者报告的完全缓解。那,目标
该建议的是利用计算机视觉技术来自动评估假设的自我伤害
视觉严重性指标,学习新的视觉严重性指标,并确定这些视觉信号的效用
预测前瞻性自杀企图风险。将招募16至18岁的社区青少年
在Facebook和Instagram上,如果他们目前具有自我伤害之后的物理标记。
参与者将安全地上传继发于故意自伤的标记图像。一个子集
参与者将在三个月内纵向跟踪,以评估前瞻性自杀尝试。我们将
采用深度卷积神经元网络,一类人工神经元网络,开发算法
检测自我伤害的严重性指数,并检查其在预测短期前瞻性自杀方面的准确性
风险。我们将通过将其应用于单独的临床来评估算法子集的概括性
16至18岁的精神科医院住院青少年样本。这项概念证明的研究将设定
确定追求我们的长期目标的可行性的阶段
精神病护理入口点(例如急诊部门,住院单位)用于评估该技术是否
可以增加当前的自杀风险评估模型,然后作为临床决策支持工具
帮助临床医生评估自杀风险。这项研究很重要,因为它与NIMH/国家行动保持一致
自杀预防联盟的优先研究议程,预防自杀的理想目标2
使用可行有效的评估来确定潜水员人群和环境中的自杀风险
鉴于我们的目标
预测期是三个月。
项目成果
期刊论文数量(0)
专著数量(0)
科研奖励数量(0)
会议论文数量(0)
专利数量(0)
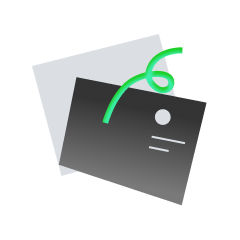
暂无数据
数据更新时间:2024-06-01
Taylor A Burke的其他基金
Multimodal Dynamics of Parent-child Interactions and Suicide Risk
亲子互动和自杀风险的多模态动力学
- 批准号:1051022710510227
- 财政年份:2022
- 资助金额:$ 26.55万$ 26.55万
- 项目类别:
Multimodal Dynamics of Parent-child Interactions and Suicide Risk
亲子互动和自杀风险的多模态动力学
- 批准号:1070098210700982
- 财政年份:2022
- 资助金额:$ 26.55万$ 26.55万
- 项目类别:
Passive Assessment of Behavioral Warning Signs for Suicide Risk in Adolescents: An Idiographic Approach
青少年自杀风险行为警告信号的被动评估:一种具体方法
- 批准号:1036606710366067
- 财政年份:2021
- 资助金额:$ 26.55万$ 26.55万
- 项目类别:
Passive Assessment of Behavioral Warning Signs for Suicide Risk in Adolescents: An Idiographic Approach
青少年自杀风险行为警告信号的被动评估:一种具体方法
- 批准号:1076270110762701
- 财政年份:2021
- 资助金额:$ 26.55万$ 26.55万
- 项目类别:
Passive Assessment of Behavioral Warning Signs for Suicide Risk in Adolescents: An Idiographic Approach
青少年自杀风险行为警告信号的被动评估:一种具体方法
- 批准号:1043304210433042
- 财政年份:2021
- 资助金额:$ 26.55万$ 26.55万
- 项目类别:
Passive Assessment of Behavioral Warning Signs for Suicide Risk in Adolescents: An Idiographic Approach
青少年自杀风险行为警告信号的被动评估:一种具体方法
- 批准号:1061450910614509
- 财政年份:2021
- 资助金额:$ 26.55万$ 26.55万
- 项目类别:
Passive Assessment of Behavioral Warning Signs for Suicide Risk in Adolescents: An Idiographic Approach
青少年自杀风险行为警告信号的被动评估:一种具体方法
- 批准号:1019013110190131
- 财政年份:2021
- 资助金额:$ 26.55万$ 26.55万
- 项目类别:
Leveraging Computer Vision to Augment Suicide Risk Prediction
利用计算机视觉增强自杀风险预测
- 批准号:1047569010475690
- 财政年份:2021
- 资助金额:$ 26.55万$ 26.55万
- 项目类别:
相似海外基金
Uncovering proximal antecedents to Black male suicide using real-time approaches
使用实时方法揭示黑人男性自杀的近因
- 批准号:1064395610643956
- 财政年份:2022
- 资助金额:$ 26.55万$ 26.55万
- 项目类别:
Uncovering proximal antecedents to Black male suicide using real-time approaches
使用实时方法揭示黑人男性自杀的近因
- 批准号:1044875910448759
- 财政年份:2022
- 资助金额:$ 26.55万$ 26.55万
- 项目类别:
Leveraging Computer Vision to Augment Suicide Risk Prediction
利用计算机视觉增强自杀风险预测
- 批准号:1047569010475690
- 财政年份:2021
- 资助金额:$ 26.55万$ 26.55万
- 项目类别:
Feasibility Studies for Clinical Trials
临床试验的可行性研究
- 批准号:82518348251834
- 财政年份:2011
- 资助金额:$ 26.55万$ 26.55万
- 项目类别:
Alcohol Trajectories and Service Use in Young Adults: Nine Years Post Treatment
年轻人的酒精轨迹和服务使用:治疗后九年
- 批准号:78901427890142
- 财政年份:2010
- 资助金额:$ 26.55万$ 26.55万
- 项目类别: