Data driven model adaptation for identifying stochastic digital twins of bridges
用于识别桥梁随机数字孪生的数据驱动模型适应
基本信息
- 批准号:501811638
- 负责人:
- 金额:--
- 依托单位:
- 依托单位国家:德国
- 项目类别:Priority Programmes
- 财政年份:
- 资助国家:德国
- 起止时间:
- 项目状态:未结题
- 来源:
- 关键词:
项目摘要
The goal of the proposal is the development of methods to support the creation of digital twins of bridges based on simulation models. In particular, this should include an estimate of the quality of prognosis of simulation results. This estimate is obtained via Bayes inference procedures using both laboratory as well as monitoring data. A particular challenge in the development of a simulation model for bridges is that in many cases the modelling assumptions from the design differ from the real implementation. For this reason, an adaptive process is necessary in which additional physical effects are added or changed (boundary conditions, geometry of the reinforcement layers, constitutive laws) until the digital and the real twin have identical properties within prescribed tolerances. However, the comparison between measured data and simulation results cannot be done manually, on the one hand due to the high dimension of the data space (large number and different types of sensors), on the other hand also due to the large amount of data resulting from monitoring. Furthermore, due to the ellipticity of the underlying differential equations, local changes at one point in the model lead to changes of the global behavior, i.e. the localization of model bias only from the data is often not possible. Therefore, the goal of the project is to develop a semi-automatic approach. Based on an initial, physically motivated model, the parameters of the model are calibrated using the data. In addition, a possible model improvement is identified from the data. Different approaches based on Gaussian processes, random fields for material parameters or a sparse dictionary learning approaches will be compared. Based on this data-driven model bias, the modeler shall develop a physically motivated model improvement (possibly with additional parameters) until the simulation model is sufficiently accurate. A large number of calculations of the forward model (FE model of the bridge) is required to calibrate the model parameters. In order to improve the performance of the methodology, the forward models are replaced by metamodels. In particular, Gaussian processes and Bayesian physics-informed neural networks are used for this purpose. The methodology will be validated within the project on different examples with varying degrees of complexity, starting with virtual data with simple beam models (including faults), existing bridge demonstrators on our test site and, finally, with real data from the demonstrator in the SPP. Parallel to the implementation of the methodology, a continuous development of the interfaces to the other projects in the SPP and an integration into a common platform is intended.
该提案的目的是开发基于仿真模型来支持数字双桥创建数字双胞胎的方法。特别是,这应该包括对模拟结果预后质量的估计。该估计值是通过使用实验室和监视数据的贝叶斯推理程序获得的。 开发桥梁模拟模型的一个特殊挑战是,在许多情况下,设计的建模假设与实际实施不同。因此,必须进行自适应过程,其中添加或更改了其他物理效应(边界条件,增强层的几何形状,本构定律),直到数字和实际双胞胎在规定的公差内具有相同的属性。但是,另一方面,由于数据空间的高维度(大数量和不同类型的传感器),不能手动进行比较,另一方面也无法手动进行,另一方面也是由于监测导致的大量数据。此外,由于基础微分方程的椭圆度,模型中一个点的局部变化会导致全局行为的变化,即通常不可能从数据中定位模型偏差。 因此,该项目的目的是开发一种半自动方法。基于最初的,物理动机的模型,使用数据校准了模型的参数。另外,从数据中确定了可能的改进。将根据高斯过程,材料参数的随机字段或稀疏的词典学习方法的不同方法进行比较。基于此数据驱动的模型偏差,建模者应开发出具有物理动机的模型改进(可能具有其他参数),直到模拟模型足够准确。 需要大量的向前模型计算(桥的Fe模型)来校准模型参数。为了提高方法的性能,向前模型被元模型所取代。特别是,用于此目的的高斯过程和贝叶斯物理知识的神经网络用于此目的。 该方法将在项目中的不同示例中以不同程度的复杂性进行验证,从简单的梁模型(包括故障)开始,我们的测试站点上的现有桥梁演示器,最后是来自SPP示威者的真实数据。与实施方法的实施相似,旨在将接口的界面连续开发到SPP中的其他项目,并将集成到通用平台中。
项目成果
期刊论文数量(0)
专著数量(0)
科研奖励数量(0)
会议论文数量(0)
专利数量(0)
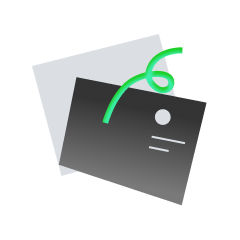
暂无数据
数据更新时间:2024-06-01
Dr.-Ing. Jörg F. ...的其他基金
An adaptive hyperreduced domain decomposition approach for nonlinear heterogeneous structures
非线性异质结构的自适应超简化域分解方法
- 批准号:394350870394350870
- 财政年份:2017
- 资助金额:----
- 项目类别:Research GrantsResearch Grants
Numerical and experimental investigations for the modeling of the time-dependent deformation characteristics of concrete on the mesoscale with coupled models for mechanical and hygric effects
利用机械和湿度效应耦合模型对介观尺度上的混凝土随时间变形特性进行建模的数值和实验研究
- 批准号:252766671252766671
- 财政年份:2014
- 资助金额:----
- 项目类别:Research GrantsResearch Grants
Homogenisierung und Multiskalensimulationen von Lokalisierungsphänomenen
定位现象的均质化和多尺度模拟
- 批准号:166630204166630204
- 财政年份:2010
- 资助金额:----
- 项目类别:Research FellowshipsResearch Fellowships
CISM-Kurs "Advances of Soft Computing in Engineering" (08.-12.10.2007 in Udine/Italien)
CISM 课程“工程软计算的进展”(2007 年 10 月 8 日至 12 日,意大利乌迪内)
- 批准号:6149902361499023
- 财政年份:2007
- 资助金额:----
- 项目类别:Research GrantsResearch Grants
CISM-Kurs "Multiscale Modelling of Damage and Fracture Processes in Composite Materials"
CISM 课程“复合材料损伤和断裂过程的多尺度建模”
- 批准号:54362905436290
- 财政年份:2004
- 资助金额:----
- 项目类别:Research GrantsResearch Grants
A regularized concrete model for high strain rates with a FAIR parameter estimation framework
具有 FAIR 参数估计框架的高应变率正则化混凝土模型
- 批准号:544609570544609570
- 财政年份:
- 资助金额:----
- 项目类别:Research GrantsResearch Grants
相似国自然基金
基于数据-机理协同驱动降阶模型的质子交换膜燃料电池多物理场孪生
- 批准号:52306112
- 批准年份:2023
- 资助金额:30 万元
- 项目类别:青年科学基金项目
复杂荷载下热活化煤矸石混凝土数据驱动细观损伤本构模型研究
- 批准号:52304079
- 批准年份:2023
- 资助金额:30 万元
- 项目类别:青年科学基金项目
基于模型与数据双驱动深度字典网络的稀疏角度CT重建方法研究
- 批准号:62371414
- 批准年份:2023
- 资助金额:50 万元
- 项目类别:面上项目
城市复杂场景下的数据-模型联合驱动遥感影像阴影校正方法
- 批准号:42371366
- 批准年份:2023
- 资助金额:48 万元
- 项目类别:面上项目
模型与数据双驱动的新能源电力系统电压稳定多时间尺度优化控制方法研究
- 批准号:52377096
- 批准年份:2023
- 资助金额:50 万元
- 项目类别:面上项目
相似海外基金
A data-driven modeling approach for augmenting climate model simulations and its application to Pacific-Atlantic interbasin interactions
增强气候模型模拟的数据驱动建模方法及其在太平洋-大西洋跨流域相互作用中的应用
- 批准号:23K2594623K25946
- 财政年份:2024
- 资助金额:----
- 项目类别:Grant-in-Aid for Scientific Research (B)Grant-in-Aid for Scientific Research (B)
Identification of Prospective Predictors of Alcohol Initiation During Early Adolescence
青春期早期饮酒的前瞻性预测因素的鉴定
- 批准号:1082391710823917
- 财政年份:2024
- 资助金额:----
- 项目类别:
Data-driven model links BMIz to gene expression in pediatric asthma
数据驱动模型将 BMIz 与小儿哮喘基因表达联系起来
- 批准号:493135493135
- 财政年份:2023
- 资助金额:----
- 项目类别:
A data-driven modeling approach for augmenting climate model simulations and its application to Pacific-Atlantic interbasin interactions
增强气候模型模拟的数据驱动建模方法及其在太平洋-大西洋跨流域相互作用中的应用
- 批准号:23H0125023H01250
- 财政年份:2023
- 资助金额:----
- 项目类别:Grant-in-Aid for Scientific Research (B)Grant-in-Aid for Scientific Research (B)
Dissociating respiratory depression and analgesia via a data-driven model of interacting respiratory and pain networks
通过呼吸和疼痛网络相互作用的数据驱动模型分离呼吸抑制和镇痛
- 批准号:1064430010644300
- 财政年份:2023
- 资助金额:----
- 项目类别: