Collaborative Research: FW-HTF-RL: Understanding the Ethics, Development, Design, and Integration of Interactive Artificial Intelligence Teammates in Future Mental Health Work
合作研究:FW-HTF-RL:了解未来心理健康工作中交互式人工智能队友的伦理、开发、设计和整合
基本信息
- 批准号:2326146
- 负责人:
- 金额:$ 80.17万
- 依托单位:
- 依托单位国家:美国
- 项目类别:Standard Grant
- 财政年份:2023
- 资助国家:美国
- 起止时间:2023-09-15 至 2027-08-31
- 项目状态:未结题
- 来源:
- 关键词:
项目摘要
This research project is a response to the national shortage of mental health workers who are skilled in research-supported treatment protocols. The investigators seek to understand how recent innovations in artificial intelligence (AI) can effectively and ethically address and mitigate unmet demands for mental health treatment. Mental health workers include several related professions including clinical psychologists, social workers, and counselors. This undersized workforce is in dire need for scalable and effective upskilling in order to facilitate widespread and routine implementation of research-supported treatment protocols. Upskilling the workforce has been constrained because there are insufficient numbers of expert trainers to keep mental health workers proficient in the best available practices. This workforce has primarily relied on initial human-to-human training (e.g., graduate school) followed by relatively minimal follow-up observation and feedback throughout one’s career. As a result, millions of Americans with mental health conditions have restricted access to effective, research-supported care. The mental health workforce will benefit from technology that helps clinicians learn and sustain their use of research-supported treatment protocols. Important to this need, modern AI systems have developed to such a point where the technology can be considered a teammate in highly skilled work contexts, not simply a data processing tool. Integrating recent advancements in AI, the interdisciplinary team of investigators will develop an interactive AI system that can quickly evaluate a mental health worker’s performance with a patient, provide actionable feedback to the worker, and receive input from the worker so that feedback is based on what that individual worker needs to learn. This computational system, called the Trustworthy, Explainable, and Adaptive Monitoring Machine for AI Teams (TEAMMAIT), will function as an objective, nonjudgmental, and confidential colleague who can provide individualized feedback over a period of time. This type of Worker-AI Teaming has potential to transform the upskilling process by reducing the reliance on cost-prohibitive and scarcely available human-to-human training. While this project focuses on mental health work due to critical unmet demands, insights from this project can generalize to other healthcare and educational contexts.This project brings together several disciplines including clinical psychology, industrial-organizational psychology, human-computer interaction, and information science. The team is structured to achieve multiple convergent goals. First, the investigators aim to better understand how introducing Worker-AI Teams will impact the expected competencies of mental health workers including how to collaborate with AI and respond to risks. Second, the investigators aim to gain insights regarding how to design AI Teammates in mental health work that facilitate ethical and effective Worker-AI Teaming. And third, the investigators aim to learn how to develop and deploy AI Teammates that can upskill the mental health workforce. A prototype of TEAMMAIT will be evaluated in diverse settings and with diverse workers and diverse patient populations. Data collected from prototype users will result in a set of development guidelines for Worker-AI Teaming in mental health work, as well as a set of generalizable ethical guidelines for developing and using these systems. Interviews with users will provide insights into how mental health workplaces can best prepare for Worker-AI Teaming and optimize its use while maintaining worker well-being and high-quality clinical care. The research plan will provide insights that will help make mental health worker upskilling more scalable and effective in real-world clinics, improving access to best practices for diverse patient populations across the United States. This project has been funded by the Future of Work at the Human-Technology Frontier cross-directorate program to promote deeper basic understanding of the interdependent human-technology partnership in work contexts by advancing the design of intelligent work technologies that operate in harmony with human workers.This award reflects NSF's statutory mission and has been deemed worthy of support through evaluation using the Foundation's intellectual merit and broader impacts review criteria.
该研究项目是对全国范围内精神卫生工作者短缺的回应,这些精神卫生工作者熟练于研究支持治疗方案。研究人员试图了解人工智能(AI)的最新创新如何有效和道德上解决,并减轻对心理健康治疗的未满足需求。精神卫生工作者包括几位相关专业人员,包括临床心理学家,社会工作者和共同工作者。这个尺寸不足的劳动力迫切需要可扩展有效的高技能,以促进研究支持的治疗方案的宽度和常规实施。劳动力受到限制,因为没有足够数量的专家培训师来保持精神卫生工作者精通最佳实践。该劳动力主要依靠最初的人类对人类培训(例如研究生院),随后在整个职业生涯中进行了相对最小的后续观察和反馈。结果,数百万具有精神健康状况的美国人限制了获得有效的研究支持的护理。心理健康劳动力将受益于帮助临床医生学习和维持其对研究支持的治疗方案的技术。对于这种需求来说,现代AI系统已经发展到这样的程度,即该技术可以被视为高技能工作环境中的队友,而不仅仅是数据处理工具。将最新进步整合在AI中,研究人员的跨学科团队将开发一个交互式AI系统,该系统可以快速评估心理健康工作者与患者的表现,向工人提供可行的反馈,并收到工人的意见,以便反馈基于个人工人所需的学习所需的内容。该计算系统称为AI团队(TeamMait)的可信赖,可解释和自适应监视机,将充当客观,非判断和自信的同事,他们可以在一段时间内提供个性化的反馈。这种类型的工人组合有可能通过减少对成本良好和几乎不可用的人类对人类培训的依赖来改变高技能的过程。尽管该项目由于未满足的需求而着重于心理健康工作,但该项目的见解可以推广到其他医疗保健和教育环境。该项目汇集了几个学科,包括临床心理学,工业组织心理学,人力计算机互动和信息科学。该团队的结构是实现多个收敛目标。首先,调查人员旨在更好地了解介绍工人AI团队将如何影响精神卫生工作者的预期能力,包括如何与AI合作并应对风险。其次,调查人员旨在获得有关如何设计AI在心理健康工作中的AI队友的见解,以促进道德和有效的工人组合。第三,调查人员旨在学习如何发展和部署可以提高心理健康劳动力的AI队友。 Teammait的原型将在潜水员的环境以及潜水员和潜水员的患者人群中进行评估。从原型用户收集的数据将制定一系列针对心理健康工作中的工人组合的开发准则,以及一套可开发和使用这些系统的可通用的道德准则。与用户的访谈将提供有关心理健康工作场所如何最好地为工人组合做准备并优化其使用的洞察力,同时保持工人福祉和高质量的临床护理。该研究计划将提供见解,这将有助于使精神卫生工作者在现实诊所更加可扩展和有效,从而改善美国潜水者患者人群的最佳实践的机会。该项目的资助是由人类技术前沿跨领域的工作的未来资助,旨在通过推进与人类工人和谐相处的智能工作技术的设计,对工作环境中相互依存的人类技术伙伴关系的更深入的理解,这反映了NSF的法定任务和支持者的支持者,这表明了这一奖项的良好依据,这表明了这一范围的范围。
项目成果
期刊论文数量(0)
专著数量(0)
科研奖励数量(0)
会议论文数量(0)
专利数量(0)
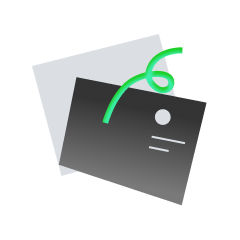
暂无数据
数据更新时间:2024-06-01
相似国自然基金
基于DES/FW-H方法的共轴刚性旋翼气动噪声预测方法及机理研究
- 批准号:
- 批准年份:2021
- 资助金额:30 万元
- 项目类别:青年科学基金项目
基于DES/FW-H方法的共轴刚性旋翼气动噪声预测方法及机理研究
- 批准号:12102154
- 批准年份:2021
- 资助金额:24.00 万元
- 项目类别:青年科学基金项目
番茄果重基因FW9.1的图位克隆及与其它果重基因互作效应研究
- 批准号:31872949
- 批准年份:2018
- 资助金额:56.0 万元
- 项目类别:面上项目
Fw2.2同源基因调控库尔勒香梨果实大小的分子机理研究
- 批准号:31760561
- 批准年份:2017
- 资助金额:38.0 万元
- 项目类别:地区科学基金项目
番茄果实重量基因FW11.3控制细胞大小的分子机理研究
- 批准号:31471889
- 批准年份:2014
- 资助金额:85.0 万元
- 项目类别:面上项目
相似海外基金
Collaborative Research [FW-HTF-RL]: Enhancing the Future of Teacher Practice via AI-enabled Formative Feedback for Job-Embedded Learning
协作研究 [FW-HTF-RL]:通过人工智能支持的工作嵌入学习形成性反馈增强教师实践的未来
- 批准号:23261702326170
- 财政年份:2023
- 资助金额:$ 80.17万$ 80.17万
- 项目类别:Standard GrantStandard Grant
Collaborative Research: FW-HTF-RM: Human-in-the-Lead Construction Robotics: Future-Proofing Framing Craft Workers in Industrialized Construction
合作研究:FW-HTF-RM:人类主导的建筑机器人:工业化建筑中面向未来的框架工艺工人
- 批准号:23261602326160
- 财政年份:2023
- 资助金额:$ 80.17万$ 80.17万
- 项目类别:Standard GrantStandard Grant
Collaborative Research: FW-HTF-RL: Trapeze: Responsible AI-assisted Talent Acquisition for HR Specialists
合作研究:FW-HTF-RL:Trapeze:负责任的人工智能辅助人力资源专家人才获取
- 批准号:23261932326193
- 财政年份:2023
- 资助金额:$ 80.17万$ 80.17万
- 项目类别:Standard GrantStandard Grant
Collaborative Research: FW-HTF-RM: Artificial Intelligence Technology for Future Music Performers
合作研究:FW-HTF-RM:未来音乐表演者的人工智能技术
- 批准号:23261982326198
- 财政年份:2023
- 资助金额:$ 80.17万$ 80.17万
- 项目类别:Standard GrantStandard Grant
FW-HTF-RL/Collaborative Research: Future of Digital Facility Management (Future of DFM)
FW-HTF-RL/协作研究:数字设施管理的未来(DFM 的未来)
- 批准号:23264072326407
- 财政年份:2023
- 资助金额:$ 80.17万$ 80.17万
- 项目类别:Standard GrantStandard Grant