Collaborative Research: SaTC: TTP: Small: DeFake: Deploying a Tool for Robust Deepfake Detection
协作研究:SaTC:TTP:小型:DeFake:部署强大的 Deepfake 检测工具
基本信息
- 批准号:2310131
- 负责人:
- 金额:$ 11.42万
- 依托单位:
- 依托单位国家:美国
- 项目类别:Standard Grant
- 财政年份:2022
- 资助国家:美国
- 起止时间:2022-10-01 至 2024-09-30
- 项目状态:已结题
- 来源:
- 关键词:
项目摘要
Deepfakes – videos that are generated or manipulated by artificial intelligence – pose a major threat for spreading disinformation, threatening blackmail, and new forms of phishing. They are already widely used in creating non-consensual pornography, and have begun to be used to undermine governments and elections. Even the threat of deepfakes has cast doubts on the authenticity of videos in the news. Journalists, who have a key role in verifying information, especially need help to deal with ever-improving deepfake technology. Recent results on detecting deepfakes are promising, with close to 100% accuracy in lab tests, but few systems are available for real-world use. It is critical to move beyond accuracy on curated datasets and address the needs of journalists who could benefit from these advances.The objective of this transition-to-practice project is to develop the DeFake tool, a system that utilizes advanced machine learning to help journalists detect deepfakes in a way that is robust, intuitive, and provides results that are explainable to the general public. To meet this objective, the project team is engaged in four main tasks: (1) Making the tool robust to new types of deepfakes, and having it show users why a video is fake; (2) Protecting the tool from adversarial examples – small perturbations to a video that are specially crafted to fool detection systems; (3) Working with journalists to understand what they need from the tool, and building an online community to discuss deepfakes and their detection; and (4) Integrating advances from the other tasks into a stable, efficient, and useful tool, and actively disseminating this tool to journalists. The project team is also leveraging visually interesting deepfakes to develop engaging education and outreach efforts, such as a museum-style exhibit on deepfake detection meant for broad audiences of all ages.This award reflects NSF's statutory mission and has been deemed worthy of support through evaluation using the Foundation's intellectual merit and broader impacts review criteria.
Deepfakes - 由人工智能产生或操纵的视频 - 对传播虚假信息,威胁勒索和新形式的网络钓鱼构成了主要威胁。它们已经被广泛用于创建非自愿性色情制品,并已开始破坏政府和选举。即使是深击的威胁也引起了新闻中视频真实性的怀疑。记者在验证信息方面具有关键作用,尤其需要帮助来处理不断改进的深层技术。有望在实验室测试中获得有关检测深泡沫的最新结果,但在实验室测试中的准确性接近100%,但很少有用于现实世界的系统。超越策划数据集的准确性并满足可以从这些进步中受益的新闻工作者的需求是至关重要的。这个过渡到实践的项目的目标是开发Defake工具,该系统利用先进的机器学习来帮助新闻记者以强大的,直觉的方式来检测深层攻击,并为公众提供结果。为了满足这一目标,项目团队从事四个主要任务:(1)使工具适合新型Deepfakes,并让用户向用户展示为什么视频是假的; (2)保护该工具免受对抗性示例 - 针对专门为愚弄检测系统而设计的视频的小扰动; (3)与记者合作,了解他们从工具中需要什么,并建立在线社区讨论深层摄影及其检测; (4)将其他任务的进步整合到稳定,高效且有用的工具中,并积极地将此工具传播给记者。该项目团队还利用视觉上有趣的深层发展来开发引人入胜的教育和外展工作,例如有关所有年龄段的广泛受众的博物馆风格的展览。该奖项反映了NSF的法定任务,并通过使用该基金会的智力和更广泛的影响来评估诚实的支持,并被认为是诚实的支持。
项目成果
期刊论文数量(0)
专著数量(0)
科研奖励数量(0)
会议论文数量(0)
专利数量(0)
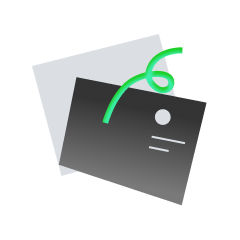
暂无数据
数据更新时间:2024-06-01
Andrea Hickerson的其他基金
Collaborative Research: SaTC: TTP: Small: DeFake: Deploying a Tool for Robust Deepfake Detection
协作研究:SaTC:TTP:小型:DeFake:部署强大的 Deepfake 检测工具
- 批准号:20401252040125
- 财政年份:2021
- 资助金额:$ 11.42万$ 11.42万
- 项目类别:Standard GrantStandard Grant
相似国自然基金
支持二维毫米波波束扫描的微波/毫米波高集成度天线研究
- 批准号:62371263
- 批准年份:2023
- 资助金额:52 万元
- 项目类别:面上项目
腙的Heck/脱氮气重排串联反应研究
- 批准号:22301211
- 批准年份:2023
- 资助金额:30 万元
- 项目类别:青年科学基金项目
水系锌离子电池协同性能调控及枝晶抑制机理研究
- 批准号:52364038
- 批准年份:2023
- 资助金额:33 万元
- 项目类别:地区科学基金项目
基于人类血清素神经元报告系统研究TSPYL1突变对婴儿猝死综合征的致病作用及机制
- 批准号:82371176
- 批准年份:2023
- 资助金额:49 万元
- 项目类别:面上项目
FOXO3 m6A甲基化修饰诱导滋养细胞衰老效应在补肾法治疗自然流产中的机制研究
- 批准号:82305286
- 批准年份:2023
- 资助金额:30 万元
- 项目类别:青年科学基金项目
相似海外基金
Collaborative Research: SaTC: CORE: Medium: Using Intelligent Conversational Agents to Empower Adolescents to be Resilient Against Cybergrooming
合作研究:SaTC:核心:中:使用智能会话代理使青少年能够抵御网络诱骗
- 批准号:23309402330940
- 财政年份:2024
- 资助金额:$ 11.42万$ 11.42万
- 项目类别:Continuing GrantContinuing Grant
Collaborative Research: SaTC: CORE: Medium: Differentially Private SQL with flexible privacy modeling, machine-checked system design, and accuracy optimization
协作研究:SaTC:核心:中:具有灵活隐私建模、机器检查系统设计和准确性优化的差异化私有 SQL
- 批准号:23172322317232
- 财政年份:2024
- 资助金额:$ 11.42万$ 11.42万
- 项目类别:Continuing GrantContinuing Grant
Collaborative Research: NSF-BSF: SaTC: CORE: Small: Detecting malware with machine learning models efficiently and reliably
协作研究:NSF-BSF:SaTC:核心:小型:利用机器学习模型高效可靠地检测恶意软件
- 批准号:23383012338301
- 财政年份:2024
- 资助金额:$ 11.42万$ 11.42万
- 项目类别:Continuing GrantContinuing Grant
Collaborative Research: SaTC: CORE: Medium: Differentially Private SQL with flexible privacy modeling, machine-checked system design, and accuracy optimization
协作研究:SaTC:核心:中:具有灵活隐私建模、机器检查系统设计和准确性优化的差异化私有 SQL
- 批准号:23172332317233
- 财政年份:2024
- 资助金额:$ 11.42万$ 11.42万
- 项目类别:Continuing GrantContinuing Grant
Collaborative Research: NSF-BSF: SaTC: CORE: Small: Detecting malware with machine learning models efficiently and reliably
协作研究:NSF-BSF:SaTC:核心:小型:利用机器学习模型高效可靠地检测恶意软件
- 批准号:23383022338302
- 财政年份:2024
- 资助金额:$ 11.42万$ 11.42万
- 项目类别:Continuing GrantContinuing Grant