RI: Medium: Collaborative Research: Causal Inference: Identification, Learning, and Decision-Making
RI:媒介:协作研究:因果推理:识别、学习和决策
基本信息
- 批准号:2011463
- 负责人:
- 金额:$ 34.19万
- 依托单位:
- 依托单位国家:美国
- 项目类别:Standard Grant
- 财政年份:2019
- 资助国家:美国
- 起止时间:2019-07-01 至 2020-07-31
- 项目状态:已结题
- 来源:
- 关键词:
项目摘要
Understanding the causal mechanisms underlying an observed phenomenon is one of the primary goals of science. The realization that statistical associations in themselves are insufficient for elucidating those mechanisms has led researchers to enrich traditional statistical analysis with techniques based on "causal inference". Most of the recent advances in the field, however, operate under overly optimistic assumptions, which are often not met in practical, large-scale situations. This project seeks to develop a sound and general causal inference theory to cover those situations. The goal is to design a framework for decision-making of intelligent systems, including (1) learning a causal representation of the data-generating environment (learning), (2) performing efficient inference leveraging the learned model (planning/inference), and (3) using the new inferred representation, based on (1) and (2), to decide how to act next (decision-making). The new finding will benefit investigators in every area of the empirical sciences, including artificial intelligence, machine learning, statistics, economics, and the health and social sciences. The research is expected to fundamentally change the practice of data science in areas where the standard causal assumptions are violated (i.e., missing data, selection bias, and confounding bias). The work on decision-making is expected to pave the way toward the design of an "automated scientist", i.e., a program that combines both observational and experimental data, conducts its own experiments, and decides on the best choices of actions and policies. The project also helps to disseminate the principles of causal inference throughout the sciences by (1) engaging in the establishment of new "data science" curriculum where causal inference plays a central role, and (2) developing new educational materials for students and the general public explaining the practice of causal inference (e.g., book). Furthermore, the project supports the causal inference community by fostering a number of educational initiatives such as forums, workshops, and the creation of new incentives for the development of educational material (e.g., a "Causality Education Award").Making claims about the existence of causal connections (structural learning), the magnitude of causal effects (identification), and designing optimal interventions (decision-making) are some of the most important tasks found throughout data-driven fields. This project studies identification, learning, and decision-making settings where (1) data are missing not at random, (2) non-parametric estimation is not feasible, and (3) aggregated behavior does not translate into guidance for individual-level decision-making. Specifically, the project considers the problem when measurements are systematically distorted (missing data), which has received an enormous amount of attention in the statistical literature, but has not essentially been investigated in the context of causal inference when data are missing not at random. The project further aims to leverage the special properties of linear models, the most common first approximation to non-parametric causal inference, to elucidate causal relationships in data, and to facilitate sensitivity analysis in such models. Finally, the project considers the fundamental problem on how causal and counterfactual knowledge can speed-up experimentation and support principled decision-making. The goal is to develop a complete algorithmic theory to determine when a particular causal effect can be learned from data and how to incorporate causal knowledge learned (possibly by experimentation) so that it can be amortized over new environmental conditions.
了解观察到的现象是科学的主要目标之一。意识到,统计关联本身不足以阐明这些机制,这使研究人员通过基于“因果推理”的技术丰富了传统的统计分析。然而,该领域的大多数进步都是在过于乐观的假设下运作的,在实用的大规模情况下通常不会达到这些假设。该项目旨在开发一种合理的因果推理理论来涵盖这些情况。目的是设计一个智能系统决策的框架,包括(1)学习数据生成环境(学习)的因果表示,(2)(2)执行有效的推理,利用了学识渊博的模型(计划/推理),以及(3)使用基于(1)和(2)的新推论代表,以决定下一步(决策)。这一新发现将使经验科学各个领域的研究人员受益,包括人工智能,机器学习,统计,经济学以及健康和社会科学。预计这项研究将在违反标准因果假设(即缺失数据,选择偏见和混杂偏见的领域)从根本上改变数据科学的实践。预计决策的工作将为“自动科学家”的设计铺平道路,即结合观察性数据和实验数据,进行自己的实验,并决定采取行动和政策的最佳选择。该项目还有助于通过(1)参与建立新的“数据科学”课程,从而传播整个科学的因果推理的原理,在该课程中,因果推理起着核心作用,以及(2)为学生和一般公众开发新的教育材料,并解释了因果推理的实践(例如,书籍)。 Furthermore, the project supports the causal inference community by fostering a number of educational initiatives such as forums, workshops, and the creation of new incentives for the development of educational material (e.g., a "Causality Education Award").Making claims about the existence of causal connections (structural learning), the magnitude of causal effects (identification), and designing optimal interventions (decision-making) are some of the most在整个数据驱动字段中找到的重要任务。该项目研究了(1)数据并非随机缺失的识别,学习和决策设置,(2)非参数估计是不可行的,并且(3)汇总行为不会转化为个人级决策的指导。具体而言,当测量结果被系统地扭曲(缺少数据)时,该项目考虑了问题,该测量在统计文献中受到了广泛的关注,但在因果推断的背景下,当数据丢失而不是随机的情况下,基本上没有研究。该项目进一步旨在利用线性模型的特殊属性,这是非参数因果推断的最常见的第一个近似值,以阐明数据中的因果关系,并促进此类模型中的灵敏度分析。 最后,该项目考虑了有关因果关系和反事实知识如何加速实验和支持原则决策的基本问题。目的是开发一种完整的算法理论,以确定何时可以从数据中学习特定的因果效应,以及如何合并学习的因果知识(可能是通过实验),以便可以在新的环境条件下摊销。
项目成果
期刊论文数量(0)
专著数量(0)
科研奖励数量(0)
会议论文数量(0)
专利数量(0)
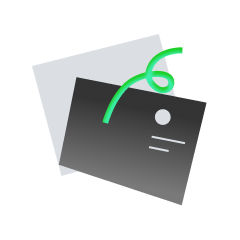
暂无数据
数据更新时间:2024-06-01
Elias Bareinboim的其他基金
CISE: Large: Causal Foundations for Decision Making and Learning
CISE:大型:决策和学习的因果基础
- 批准号:23217862321786
- 财政年份:2023
- 资助金额:$ 34.19万$ 34.19万
- 项目类别:Continuing GrantContinuing Grant
Collaborative Research: EAGER: RI: Causal Decision-Making
协作研究:EAGER:RI:因果决策
- 批准号:22317962231796
- 财政年份:2022
- 资助金额:$ 34.19万$ 34.19万
- 项目类别:Standard GrantStandard Grant
III: Towards Causal Fair Decision-making
III:走向因果公平决策
- 批准号:20409712040971
- 财政年份:2021
- 资助金额:$ 34.19万$ 34.19万
- 项目类别:Standard GrantStandard Grant
CAREER: Approximate Causal Inference
职业:近似因果推理
- 批准号:20114972011497
- 财政年份:2019
- 资助金额:$ 34.19万$ 34.19万
- 项目类别:Continuing GrantContinuing Grant
CAREER: Approximate Causal Inference
职业:近似因果推理
- 批准号:17508071750807
- 财政年份:2018
- 资助金额:$ 34.19万$ 34.19万
- 项目类别:Continuing GrantContinuing Grant
RI: Medium: Collaborative Research: Causal Inference: Identification, Learning, and Decision-Making
RI:媒介:协作研究:因果推理:识别、学习和决策
- 批准号:17049081704908
- 财政年份:2017
- 资助金额:$ 34.19万$ 34.19万
- 项目类别:Standard GrantStandard Grant
相似国自然基金
复合低维拓扑材料中等离激元增强光学响应的研究
- 批准号:12374288
- 批准年份:2023
- 资助金额:52 万元
- 项目类别:面上项目
基于管理市场和干预分工视角的消失中等企业:特征事实、内在机制和优化路径
- 批准号:72374217
- 批准年份:2023
- 资助金额:41.00 万元
- 项目类别:面上项目
托卡马克偏滤器中等离子体的多尺度算法与数值模拟研究
- 批准号:12371432
- 批准年份:2023
- 资助金额:43.5 万元
- 项目类别:面上项目
中等质量黑洞附近的暗物质分布及其IMRI系统引力波回波探测
- 批准号:12365008
- 批准年份:2023
- 资助金额:32 万元
- 项目类别:地区科学基金项目
中等垂直风切变下非对称型热带气旋快速增强的物理机制研究
- 批准号:42305004
- 批准年份:2023
- 资助金额:30 万元
- 项目类别:青年科学基金项目
相似海外基金
Collaborative Research: RI: Medium: Principles for Optimization, Generalization, and Transferability via Deep Neural Collapse
合作研究:RI:中:通过深度神经崩溃实现优化、泛化和可迁移性的原理
- 批准号:23128412312841
- 财政年份:2023
- 资助金额:$ 34.19万$ 34.19万
- 项目类别:Standard GrantStandard Grant
Collaborative Research: RI: Medium: Principles for Optimization, Generalization, and Transferability via Deep Neural Collapse
合作研究:RI:中:通过深度神经崩溃实现优化、泛化和可迁移性的原理
- 批准号:23128422312842
- 财政年份:2023
- 资助金额:$ 34.19万$ 34.19万
- 项目类别:Standard GrantStandard Grant
Collaborative Research: RI: Medium: Lie group representation learning for vision
协作研究:RI:中:视觉的李群表示学习
- 批准号:23131512313151
- 财政年份:2023
- 资助金额:$ 34.19万$ 34.19万
- 项目类别:Continuing GrantContinuing Grant
Collaborative Research: RI: Medium: Principles for Optimization, Generalization, and Transferability via Deep Neural Collapse
合作研究:RI:中:通过深度神经崩溃实现优化、泛化和可迁移性的原理
- 批准号:23128402312840
- 财政年份:2023
- 资助金额:$ 34.19万$ 34.19万
- 项目类别:Standard GrantStandard Grant
Collaborative Research: RI: Medium: Lie group representation learning for vision
协作研究:RI:中:视觉的李群表示学习
- 批准号:23131492313149
- 财政年份:2023
- 资助金额:$ 34.19万$ 34.19万
- 项目类别:Continuing GrantContinuing Grant