Collaborative Research: New Bayesian Nonparametric Paradigms of Personalized Medicine for Lung Cancer
合作研究:肺癌个体化医疗的新贝叶斯非参数范式
基本信息
- 批准号:1922567
- 负责人:
- 金额:$ 34.31万
- 依托单位:
- 依托单位国家:美国
- 项目类别:Continuing Grant
- 财政年份:2018
- 资助国家:美国
- 起止时间:2018-09-01 至 2021-08-31
- 项目状态:已结题
- 来源:
- 关键词:
项目摘要
Rapid technological advances have allowed for molecular profiling across multiple domains from a single tumor sample, supporting clinical decision making in many diseases, especially cancer. Key challenges are to effectively assimilate information across these domains to identify genomic signatures and biological entities that may be targeted by drugs, develop accurate risk prediction profiles for future patients, and identify novel patient subgroups for tailored therapy and monitoring. The primary objective of this project is the development of an innovative, flexible and scalable statistical framework for analyzing multi-domain, complex-structured, and high throughput modern array and next generation sequencing-based 'omics datasets. The work is motivated by several investigations related to lung cancer; however, the proposed methods and computational tools are broadly applicable in a variety of contexts involving high-dimensional data. From a broader scientific perspective, the application of these novel methodologies to the motivating clinical and genomic datasets will allow for principled "structure hunting". This will provide more accurate prediction of clinical outcomes, greater statistical power to detect important biologically actionable biomarkers for improved risk estimation and treatment selection for cancer diagnosis and prognosis, and better utilization of biological domain knowledge to find relationships between different platforms. It will lead to subsequent implementation of rational biomarker-based and individualized clinical trials that increase the success rate of personalized therapies based on molecular markers.To achieve these goals, the following specific aims are proposed: (1) Develop versatile and flexible statistical techniques for identifying differential genomic signatures for lung cancer in mixed, heterogeneously scaled single-domain datasets arising from array and next-generation sequencing based studies. A general class of nonparametric Bayesian models based on sound theoretical justifications will be developed and implemented using efficient, scalable algorithms. These models provide biologically interpretable summaries and enable applicability to a wide variety of high-throughput datasets. (2) Formulate integrative probabilistic frameworks for massive multiple-domain data, which coherently incorporate dependence within and between domains to accurately detect tumor subtypes and predict clinical outcomes, thus providing a catalogue of genomic aberrations associated with cancer taxonomy. (3) Foster massively parallel algorithms and high-performance computational and inferential tools that drastically reduce the computation times and increase scalability of high-throughput datasets. These scalable inferential procedures are able to assimilate information from several platforms and select flexible models with the appropriate dependence structures, while detecting optimally sparse, non-linear mechanisms for predicting and identifying tumor subtypes. Because these formulations are fully probabilistic, they offer substantial improvements over purely algorithmic approaches by accounting for different sources of variation and providing measures of inference uncertainty. Since existing simulation-based algorithms do not scale for massive datasets, theoretical properties of these models will be exploited to devise data-squashing algorithms for efficient inference. Furthermore, as traditional CPUs are limited by energy consumption, heat generation and memory access, software that harnesses the power of low-cost massively parallel computing tools such as graphics processing units (GPUs) will be developed and made freely available.
快速的技术进步允许从单个肿瘤样本跨多个域进行分子分析,从而支持许多疾病,尤其是癌症的临床决策。 主要挑战是有效地吸收这些领域的信息,以识别可能由药物靶向的基因组信号和生物实体,为未来患者开发准确的风险预测概况,并确定新的患者亚组进行量身定制治疗和监测。 该项目的主要目的是开发创新,灵活和可扩展的统计框架,用于分析多域,复杂结构和高吞吐量现代阵列和下一代测序的“基于测序”的“ OMICS数据集”。 这项工作是由与肺癌有关的几项研究激发的。但是,所提出的方法和计算工具广泛适用于涉及高维数据的各种情况。 从更广泛的科学角度来看,这些新方法在激励临床和基因组数据集中的应用将允许原则上进行“结构狩猎”。 这将为临床结果提供更准确的预测,更大的统计能力可以检测重要的生物学作用生物标志物,以改善癌症诊断和预后的风险估计和治疗选择,并更好地利用生物领域知识以找到不同平台之间的关系。 这将导致随后实施基于理性生物标志物和个性化的临床试验,从而提高基于分子标记的个性化疗法的成功率。为了实现这些目标,提出了以下特定目标:(1)开发多功能且灵活的统计技术,以确定基于肺癌和杂质量表中的肺癌的差异基因组的多功能且可灵活的统计技术研究。 将使用有效的,可扩展的算法开发和实施一类基于声音理论理由的非参数贝叶斯模型。 这些模型提供了可解释的摘要,并使适用于各种高通量数据集。 (2)为大量多域数据制定了综合概率框架,该框架连贯地纳入了域内和域之间的依赖性,以准确检测肿瘤亚型并预测临床结果,从而提供了与癌症分类法相关的基因组畸变目录。 (3)大规模促进并行算法以及高性能计算和推论工具,可大大减少计算时间并提高高通量数据集的可扩展性。 这些可扩展的推论程序能够从多个平台中吸收信息,并选择具有适当依赖性结构的柔性模型,同时检测最佳稀疏的非线性机制来预测和识别肿瘤亚型。 由于这些配方是完全概率的,因此它们通过考虑不同的变化来源并提供推理不确定性的措施,对纯粹的算法方法进行了实质性改进。 由于现有的基于仿真的算法不能扩展大规模数据集,因此将利用这些模型的理论属性来设计数据键算法以进行有效的推断。 此外,由于传统的CPU受到能源消耗,热量产生和存储器的限制,因此将开发和免费提供低成本平行计算工具(例如图形处理单元(GPU))的低成本平行计算工具的功能。
项目成果
期刊论文数量(0)
专著数量(0)
科研奖励数量(0)
会议论文数量(0)
专利数量(0)
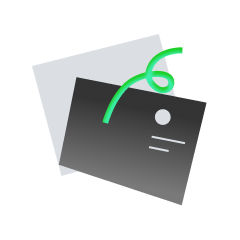
暂无数据
数据更新时间:2024-06-01
Veerabhadran Baladandayuthapani其他文献
Spatially Structured Regression for Non-conformable Spaces: Integrating Pathology Imaging and Genomics Data in Cancer
非整合空间的空间结构化回归:整合癌症病理成像和基因组数据
- DOI:
- 发表时间:20242024
- 期刊:
- 影响因子:0
- 作者:Nathaniel Osher;Jian Kang;Arvind Rao;Veerabhadran BaladandayuthapaniNathaniel Osher;Jian Kang;Arvind Rao;Veerabhadran Baladandayuthapani
- 通讯作者:Veerabhadran BaladandayuthapaniVeerabhadran Baladandayuthapani
Geometry-driven Bayesian Inference for Ultrametric Covariance Matrices
超量协方差矩阵的几何驱动贝叶斯推理
- DOI:
- 发表时间:20242024
- 期刊:
- 影响因子:0
- 作者:Tsung;Zhenke Wu;K. Bharath;Veerabhadran BaladandayuthapaniTsung;Zhenke Wu;K. Bharath;Veerabhadran Baladandayuthapani
- 通讯作者:Veerabhadran BaladandayuthapaniVeerabhadran Baladandayuthapani
共 2 条
- 1
Veerabhadran Balad...的其他基金
Collaborative Research: New Bayesian Nonparametric Paradigms of Personalized Medicine for Lung Cancer
合作研究:肺癌个体化医疗的新贝叶斯非参数范式
- 批准号:14632331463233
- 财政年份:2015
- 资助金额:$ 34.31万$ 34.31万
- 项目类别:Continuing GrantContinuing Grant
III: Small:Collaborative Research: Bayesian Model Computation for Large and High Dimensional Data Sets
III:小型:协作研究:大型高维数据集的贝叶斯模型计算
- 批准号:09151960915196
- 财政年份:2009
- 资助金额:$ 34.31万$ 34.31万
- 项目类别:Standard GrantStandard Grant
相似国自然基金
新骨架紫杉烷二萜baccataxane的化学合成、衍生化和降糖活性研究
- 批准号:82373758
- 批准年份:2023
- 资助金额:49 万元
- 项目类别:面上项目
老年重症新冠患者体内炎性细胞的特点、免疫致病机制及临床转归的研究
- 批准号:82370019
- 批准年份:2023
- 资助金额:65 万元
- 项目类别:面上项目
在幼年型粒单核细胞白血病中鉴定CD69作为其白血病干细胞新表面标记的实验研究
- 批准号:82370146
- 批准年份:2023
- 资助金额:49 万元
- 项目类别:面上项目
基于磁共振APT成像的乳腺癌新辅助治疗敏感性预测研究
- 批准号:82302153
- 批准年份:2023
- 资助金额:30 万元
- 项目类别:青年科学基金项目
新辅助化疗后CXCL12+CAF诱导胰腺癌三级淋巴结构表型特征与空间定位的分子机制研究
- 批准号:82373296
- 批准年份:2023
- 资助金额:49 万元
- 项目类别:面上项目
相似海外基金
Collaborative Research: REU Site: Earth and Planetary Science and Astrophysics REU at the American Museum of Natural History in Collaboration with the City University of New York
合作研究:REU 地点:地球与行星科学和天体物理学 REU 与纽约市立大学合作,位于美国自然历史博物馆
- 批准号:23489982348998
- 财政年份:2025
- 资助金额:$ 34.31万$ 34.31万
- 项目类别:Standard GrantStandard Grant
Collaborative Research: REU Site: Earth and Planetary Science and Astrophysics REU at the American Museum of Natural History in Collaboration with the City University of New York
合作研究:REU 地点:地球与行星科学和天体物理学 REU 与纽约市立大学合作,位于美国自然历史博物馆
- 批准号:23489992348999
- 财政年份:2025
- 资助金额:$ 34.31万$ 34.31万
- 项目类别:Standard GrantStandard Grant
Collaborative Research: New to IUSE: EDU DCL:Diversifying Economics Education through Plug and Play Video Modules with Diverse Role Models, Relevant Research, and Active Learning
协作研究:IUSE 新增功能:EDU DCL:通过具有不同角色模型、相关研究和主动学习的即插即用视频模块实现经济学教育多元化
- 批准号:23157002315700
- 财政年份:2024
- 资助金额:$ 34.31万$ 34.31万
- 项目类别:Standard GrantStandard Grant
Collaborative Research: Resolving the LGM ventilation age conundrum: New radiocarbon records from high sedimentation rate sites in the deep western Pacific
合作研究:解决LGM通风年龄难题:西太平洋深部高沉降率地点的新放射性碳记录
- 批准号:23414262341426
- 财政年份:2024
- 资助金额:$ 34.31万$ 34.31万
- 项目类别:Continuing GrantContinuing Grant
Collaborative Research: Resolving the LGM ventilation age conundrum: New radiocarbon records from high sedimentation rate sites in the deep western Pacific
合作研究:解决LGM通风年龄难题:西太平洋深部高沉降率地点的新放射性碳记录
- 批准号:23414242341424
- 财政年份:2024
- 资助金额:$ 34.31万$ 34.31万
- 项目类别:Continuing GrantContinuing Grant